In this article, we will review the inventory ordering functionality in Epicor P21, explain its limitations, and summarize how Smart Inventory Planning & Optimization (Smart IP&O) can help reduce inventory, minimize stock-outs and restore your organization’s trust in your ERP by providing robust predictive analytics, consensus-based forecasting, and what-if scenario planning.
Replenishment Planning Features within Epicor Prophet 21
Epicor P21 can manage replenishment by suggesting what to order and when via reorder point-based or forecast-driven inventory policies. Users may compute these policies externally or generate them dynamically within P21. Once the policies and forecasts have been specified, P21’s Purchase Order Requirements Generator (PORG) will create automated order suggestions of what to replenish and when by reconciling incoming supply, current on hand, outgoing demand, stocking policies, and demand forecasts.
Epicor P21 has 4 Replenishment Methods
In the item maintenance screen of Epicor P21, users can choose from one of four replenishment methods for each stock item.
- Min/Max
- Order Point/Order Quantity
- EOQ
- Up To
There are additional settings and configurations for determining lead times and accounting for order modifiers such as supplier-imposed minimum and maximum order quantities. Min/Max and Order Point/Order Quantity are considered “static” policies. EOQ and Up To are considered “dynamic” policies and computed within P21.
Min/Max
The reorder point is equal to the Min. Whenever on hand inventory drops below the Min (reorder point) the PORG report will create an order suggestion up to the Max (for example, if on hand after the breach is 20 units and the Max is 100 then the order quantity will be 80). Min/Max is considered a static policy and once entered into P21 will remain unchanged unless overridden by the user. Users often run spreadsheets to compute the Min/Max values and update them from time to time.
Order Point/Order Quantity
This is the same as the Min/Max policy except instead of ordering up to the Max, an order will be suggested for a fixed quantity defined by the user (for example, always order 100 units when the order point is breached). OP/OQ is considered a static policy and will remain unchanged unless overridden by the user. Users often run spreadsheets to compute OP/OQ values and update them from time to time.
EOQ
The EOQ policy is a reorder point-based method. The reorder point is dynamically generated based on P21’s forecast of demand over lead time + demand over the review period + safety stock. The order quantity is based on an Economic Order Quantity calculation that considers holding costs and ordering costs and attempts to recommend an order size that minimizes total cost. When on hand inventory breaches the reorder point, the PORG report will kick out an order equal to the computed EOQ.
Up To
The Up To method is another dynamic policy that relies on a reorder point. It is computed the same way as the EOQ method using P21’s forecasted demand over the lead time + demand over review period + safety stock. The order quantity suggestion is based on whatever is needed to replenish stock back “up to” the reorder point. This tends to equate to an order quantity that is consistent with the lead time demand because as demand drives stock below the reorder point, orders will be suggested “up to” the reorder point.
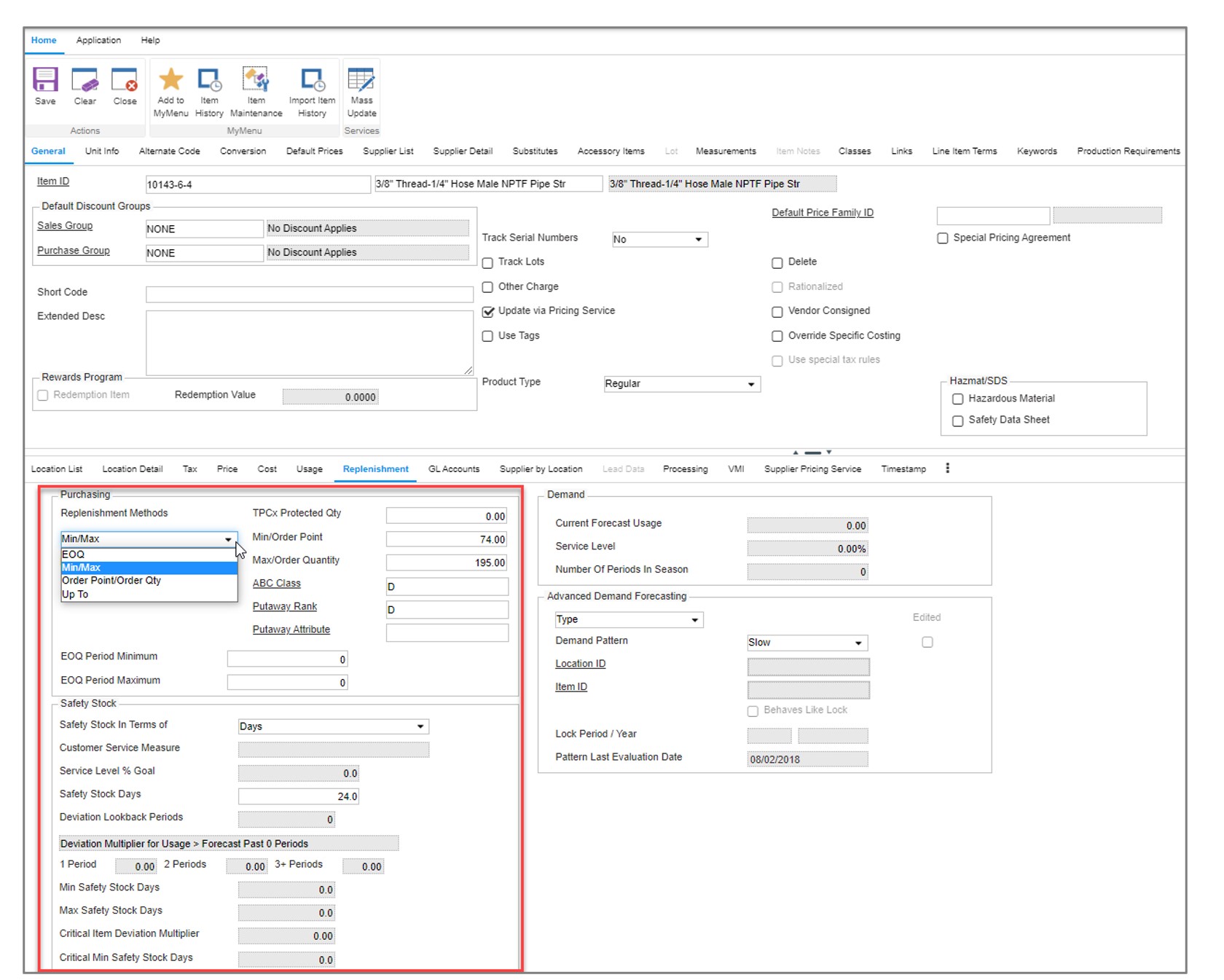
P21’s Item Maintenance Screen where users can specify the desired inventory policy and configure other settings such as safety stock and order modifiers.
Limitations
Forecast Methods
There are two forecast modes in P21: Basic and Advanced. Each use a series of averaging methods and require manual configurations and user determined classification rules to generate a demand forecast. Neither mode is designed with an out-of-the-box expert system that automatically generates forecasts that account for underlying patterns such as trend or seasonality. Lots of configuration is required that tends to inhibit user adoption and modification of the assumed forecasting rules defined in the initial implementation that may no longer be relevant. There isn’t a way to easily compare the forecast accuracy of different configurations. For example, is it better to use 24 months of history or 18 months? Is it more accurate to assume a trend should be applied when an item grows by 2% per month or should it be 10%? Is it better to assume the item is seasonal if 80% or more of it’s demand occurs in 6 months of the year or 4 months of the year? As a result, it is common for classification rules to be too broad or specific resulting in problems such as application of an incorrect forecasting model, using too much or too little history, or over/understating the trend and seasonality. To learn more about how this works, check out this blog post (coming soon)
Forecast Management & Consensus Planning
P21 lacks forecast management features that enable organizations to plan at multiple hierarchy levels such as product family, region, or by customer. Forecasts must be created at the lowest level of granularity (product by location) where demand is often too intermittent to get a good forecast. There isn’t a way to share forecasts, collaborate, review, or create forecasts at aggregate levels, and agree on the consensus plan. It is difficult to incorporate business knowledge, assess forecasts at higher levels of aggregation, and track whether overrides are improving or hurting forecast accuracy. This makes forecasting too one-dimensional and dependent on the initial math configurations.
Intermittent Demand
Many P21 customers rely on static methods (Min/Max and OP/OQ) because of the prevalence of intermittent demand. Otherwise known as “lumpy”, intermittent demand is characterized by sporadic sales, large spikes in demand, and many periods with no demand at all. When demand is intermittent, traditional forecasting and safety stock methods just don’t work. Since distributors don’t have the luxury of stocking only high movers with consistent demand, they need specialized solutions that are engineered to effectively plan intermittently demanded items. 80% or more of a distributor’s parts will have intermittent demand. The stocking policies that are generated using traditional methods such as those available in P21 and other planning applications will result in incorrect estimates of what to stock to achieve the targeted service level. As illustrated in the graph below, it isn’t possible to consistently forecast the spikes. You are stuck with a forecast that is effectively an average of the prior periods.
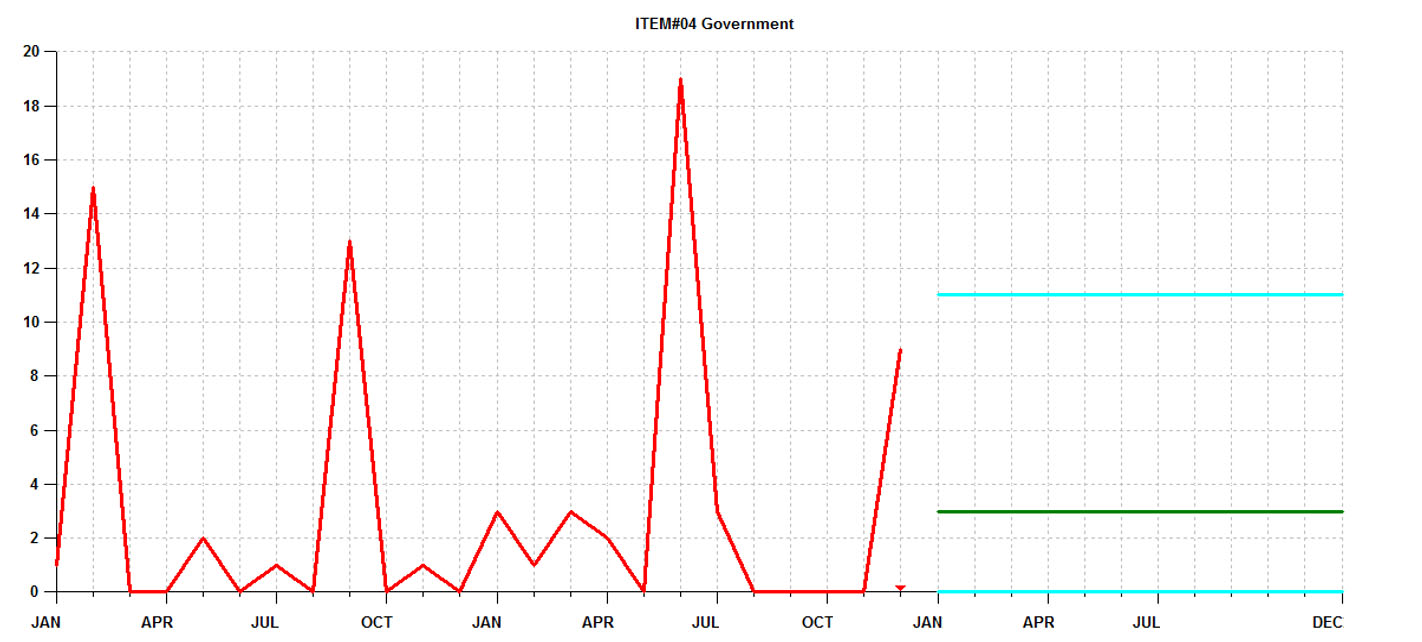
Forecasts of intermittent demand can’t predict the spikes and require safety stock buffers to protect against stockouts.
Second, P21’s safety stock methods allow you to set a target service level but the underlying logic mistakenly assumes that the demand is normally distributed. With intermittent demand, the demand isn’t “normal” and therefore the estimate of safety stock will be wrong. Here is what wrong means: when setting a service level of, for example 98%, the expectation is that 98% of the time the stock on hand will fill 100% of what the customer needs from the shelf. Using a normal distribution to compute safety stocks will result in large deviations between the targeted service level and actual service level achieved. It is not uncommon to see situations where the actual service level misses the target by 10% or more (i.e., targeted 95% but only achieved 85%).
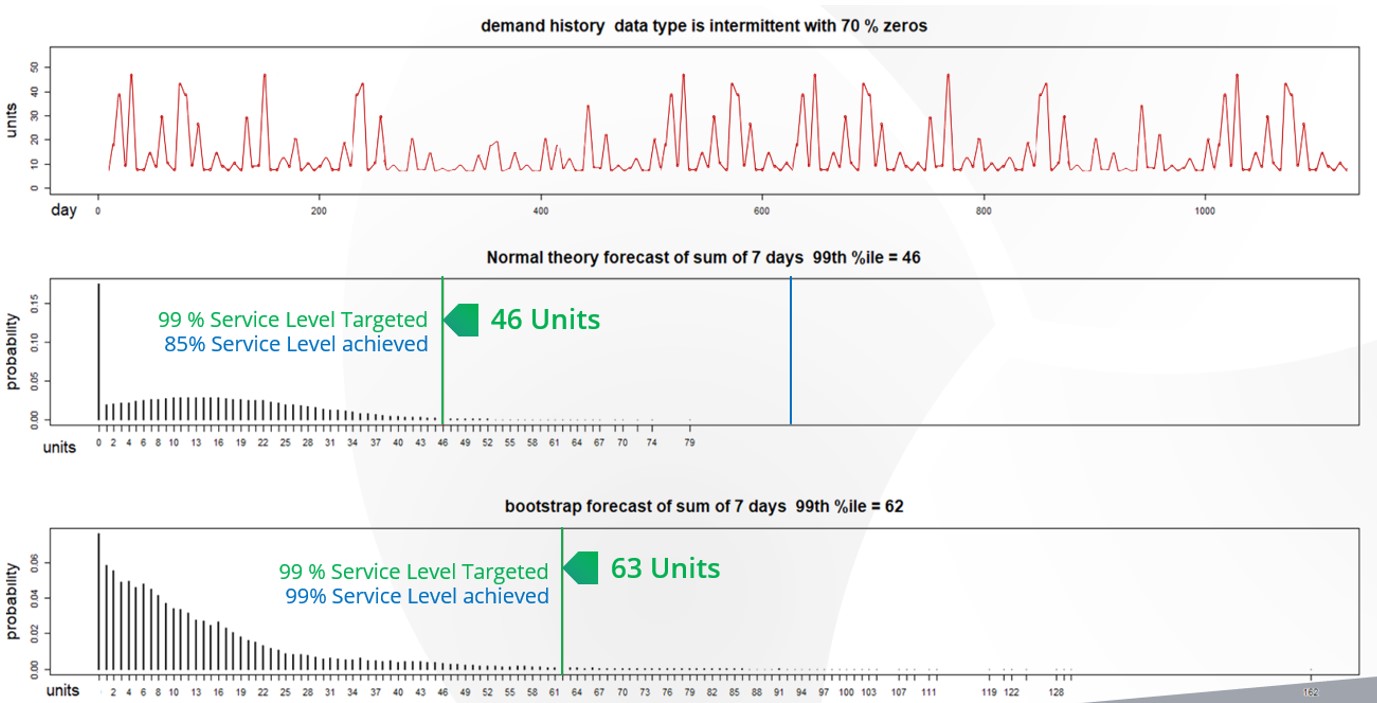
In this figure you can see the demand history of an intermittently demanded part and two distributions based on this demand history. The first distribution was generated using the same “normal distribution: logic employed by P21. The second is a simulated distribution based on Smart Software’s probabilistic forecasting. The “normal” P21 distribution recommends that 46 units is needed to achieve the 99% service level but when compared to actuals far more inventory was needed. Smart accurately predicted that 63 units was required to achieve the service level.
This blog explains how you can test your system’s service level accuracy.
Reliance on Spreadsheets & Reactive Planning
P21 customers tell us that they rely heavily on the use of spreadsheets to manage stocking policies and forecasting. Spreadsheets aren’t purpose-built for forecasting and inventory optimization. Users will often bake in user-defined rule of thumb methods that often do more harm than good. Once calculated, users must input the information back into P21 via manual file imports or even manual entry. The time consuming nature of the process leads companies to infrequently compute their inventory policies – Many months and on occasion years go by in between mass updates leading to a “set it and forget it” reactive approach, where the only time a buyer/planner reviews inventory policy is at the time of order. When policies are reviewed after the order point is already breached, it is too late. When the order point is deemed too high, manual interrogation is required to review history, calculate forecasts, assess buffer positions, and to recalibrate. The sheer volume of orders means that buyers will just release orders rather than take the painstaking time to review everything, leading to significant excess stock. If the reorder point is too low, it’s already too late. An expedite is now required driving up costs and even then, you’ll still lose sales if the customer goes elsewhere.
Limited What If Planning
Since features for modifying reorder points and order quantities are baked into P21 it is not possible to make wholesale changes across groups of items and assess predicted outcomes before deciding to commit. This forces users to adopt a “wait and see” process when it comes to modifying parameters. Planners will make a change and then monitor actuals until they are confident the change improved things. Managing this at scale—many planners are dealing with tens of thousands of items—is extremely time consuming and the end result is infrequent recalibration of inventory policy. This also contributes to reactive planning whereby planners will only review settings after a problem has occurred.
Epicor is Smarter
Epicor has partnered with Smart Software and offers Smart IP&O as a cross platform add-on to Prophet 21 complete with a bidirectional API-based integration. This enables Epicor customers to leverage built-for-purpose best of breed forecasting and inventory optimization applications. With Epicor Smart IP&O you can generate forecasts that capture trend and seasonality without having to first apply manual configurations. You will be able to automatically recalibrate policies every planning cycle using field proven, cutting-edge statistical and probabilistic models that were engineered to accurately plan for intermittent demand. Safety stocks will accurately account for demand and supply variability, business conditions, and priorities. You can leverage service level driven planning so you have just enough stock or turn on optimization methods that prescribe the most profitable stocking policies and service levels that consider the real cost of carrying inventory. You can build consensus demand forecasts that blend business knowledge with statistics, better assess customer and sales forecasts, and confidently upload forecasts and stocking policies to Epicor with a few mouse-clicks.
Smart IP&O customers routinely realize 7 figure annual returns from reduced expedites, increased sales, and less excess stock, all the while gaining a competitive edge by differentiating themselves on improved customer service. To see a recorded webinar hosted by the Epicor Users Group that profiles Smart’s Demand Planning and Inventory Optimization platform, please register here: https://smartcorp.com/epicor-smart-inventory-planning-optimization/