Demand planning, inventory planning and demand forecasting.
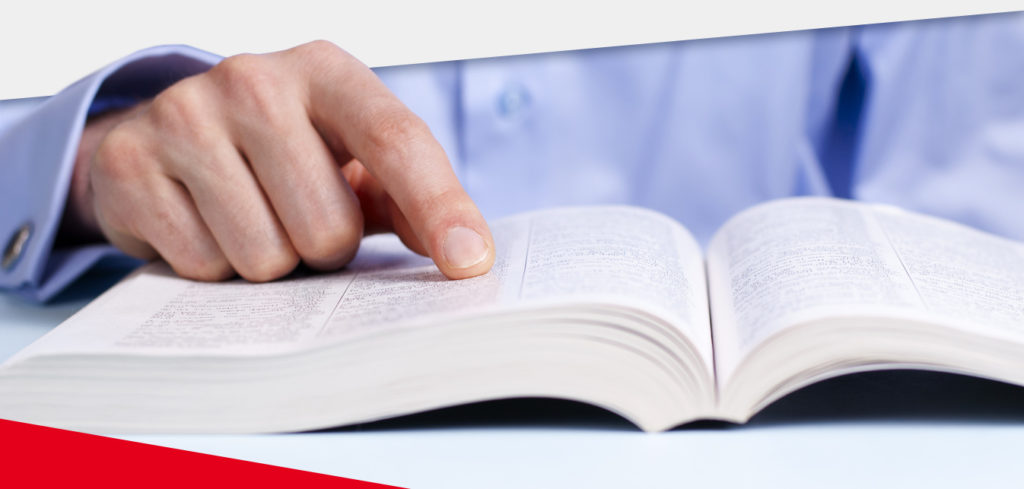
Dictionary
We find that practitioners are not always sure about the meanings of these words, which can impede effective business communication, particularly when implementing demand planning and forecasting software. Sometimes two companies (supplier and customer, supply chain software vendor and customer, etc.) use the same word but apply different meanings to it. This post aims to clarify some basic definitions.
A
accuracy
How close the forecast is to the actual. Forecast accuracy should be expressed as “forecast error” and determined as follows: Forecast – Actual. To determine the percentage error use: (Forecast – Actual)/Actual. It is not recommended to use the forecast as the denominator (i.e. (forecast – actual)/forecast)) when computing accuracy since forecast accuracy can never be worse than 100% and the planner won’t properly communicate the true error associated with the demand forecasting process.
averaging period
The number of historical data points used in moving average computations. See also moving average.
B
bootstrapping
A statistical forecasting technique based on sampling at random (with replacement) from historical values. These resampled data values are combined to form realistic demand scenarios that resemble the actual historical demand patterns without exactly repeating them.
Brown’s method
An exponential smoothing forecasting method appropriate for data with trend but without seasonality. Also known as DOUBLE exponential smoothing
C
coefficient of variation
A measure of the relative variability of the data values stored in a variable, computed as 100 times the standard deviation divided by the mean.
correlation
A measure of the degree of linearrelationship between two variables, ranging from -1 to +1, with 0 indicating no linear relationship.Large values may suggest a leading indicatorrelationship useful in regression analysis.
crosscorrelation
The correlation between oneseries and another one that is shifted forward or backward in time. See correlation.
cross-sectional data
Data organized by administrative units rather than by time periods(for example, company sales measured over all sales territories during one time period).
cumulative forecast
The forecast of the total of all the data values over the forecast horizon. Use this number when your goal is to estimate percentiles of the cumulative demand. The cumulative forecast differs from the forecast sum when the service level is not 50%. See forecast sum.
D
data cycle
The frequency with which data are collected. If the data are collected in monthly “buckets,” the data cycle is 12. Quarterly data have a data cycle of 4. Accounting months have a data cycle of 13. If you have a web site and you want to track usage by the hour, you may want to use a data cycle of 24.
decomposition
A statistical procedure that decomposes a time series into trend, seasonal, and irregular components. In multiplicative decomposition, data = trend x seasonal x irregular. In additive decomposition, data = trend + seasonal+ irregular. See also seasonal adjustment.
d.f.
Degrees of freedom. Used in computing the statistical significance of an F-statistic in a regression analysis.
dependent variable
In regression analysis, the variable to be predicted from the predictor or independent variable(s). Also known as the forecast variable. In the equation Y = 1 + 2 X, the dependent variable is Y.
.DOUBLE exponential smoothing
A forecasting method appropriate for data with trend but without seasonality. Also known as Brown’s method.
dummy variable
A variable that takes on only the values of zero or one. This type of variable is often used in regression analysis to indicate the presence or absence of some factor, such as a price promotion or a snowstorm. These play a role similar to event variables in promo/event modeling.
Durbin-Watson statistic
In regression analysis, an indicator of the degree of autocorrelation in theresiduals. Values near 2 indicate no autocorrelation, values between 0 and 2 indicate positive autocorrelation, and values between 2 and 4 indicate negative autocorrelation. If the DW is not near 2, the regression equation may still be useful for forecasting purposes, but it is not the best summary you could make of the historical relationships among your variables; furthermore, the estimates of uncertainty will be inaccurate.
E
EOQ models
Economic Order Quantity models. A class of models designed to find efficient inventory control procedures. For instance, one type of EOQ model computes the best values for when to reorder and how much to reorder, so as to balance inventory carrying costs against the cost of losing sales due to stockouts.
error
Forecast – Actual. To determine the percentage error use: (Forecast – Actual)/Actual. It is not recommended to use the forecast as the denominator (i.e. (forecast – actual)/forecast)) when computing accuracy since forecast accuracy can never be worse than 100% and the planner won’t properly communicate the true error associated with the demand forecasting process.
event model
A forecasting model used in Promo forecasting that adjusts the data for the effects of unusual events, then applies exponential smoothing. Events include sales promotions, bad weather, power outages, and other unusual circumstances of short duration.
exponential smoothing
A class of forecasting methods that averages out the random variation in a data series. Exponential smoothing computes a weighted average of all the historical data in a series. It gets its name from the way it weights the data, giving each data point a fixed percentage of the weight assigned to its successor. SmartForecasts uses the following four exponential smoothing methods: single, double, Winters’ additive and Winters’ multiplicative.
F
fit or fitted value
In regression analysis, the statistical approximation to the actual value of the forecast or dependent variable.
forecast
A prediction about the future value of a variable.
forecast interval
In SmartForecasts, the range of values indicating the margin of error in the forecast.
F-statistic
In regression analysis, an indicator of the collective ability of all the predictor (independent) variables to predict the forecast (dependent) variable. The F-statistic is used to assess the chance that a high value of R-square is nothing more than a statistical fluke. It is possible to get a fairly high value of R-square just by accident, especially when you have a small number of data cases relative to the number of predictor variables. With more data, you might get a much lower R-square. The statistical significance of a given value of the F-statistic must be assessed manually using a table of critical values and the degrees of freedom reported by SmartForecasts. If the F-statistic is not statistically significant, then none of the predictors is useful. Examine the tstatistics for individual regression coefficients only if the F-statistic is significant. See also d.f.statistical significance, coefficient, and t-statistic.
H
hedge
A judgmentally specified deceleration of a statistical trend.
holdout analysis
A method for making realistic assessments of forecast uncertainty. This method uses older data values to forecast newer values, then compares the forecasted and actual values. This mimics reality, since the forecasts are assessed against data that were not involved in their creation. There are two versions. A simple holdout analysis compares the most recent actual data values against forecasts using all the previous data. A sliding simulation uses a portion of the historical data to forecast the next several values, then slides forward one period and repeats the process, and continues in this way until using all but the last data value to predict one step ahead.
I
independent variable
See predictor variable.
intermittent demand
Demand that is mostly zero,but takes random nonzero values at random times. Intermittent demand is characteristic of spare parts and high-priced capital goods. Also known as “slow moving demand,” “lumpy demand,” “irregular demand.” and “sporadic demand.”
interval forecast
A forecast expressed as a range, for example, there is a 90% chance that sales next month will be between 100 and 150 units. See point forecast.
inventory optimization
The process of computing the minimal inventory needed to provide a desired inventory service level.
irregular component
The random variation in a time series that remains after trend and seasonality have been accounted for. The irregular component measures the effect of other influences on the series, such as snowstorms, strikes, power failures, and other nonrecurring events. See decomposition.
L
lag
The number of time periods that old values are shifted forward to pair with current values when computing an autocorrelation.
.lead
The number of time periods that future values are shifted backward to pair with current values when computing an autocorrelation.
lead time
In inventory management, the time between placing and receiving an inventory replenishment order. The inventory manager may wish to insure that there are no stockouts during this period.
lead time demand
The total of the demands in each period of the lead time.
leading indicator
An item or variable whose changes anticipate changes in some other variable.For example, knowing current new car sales may let you better predict next quarter’s sales of automotive aftermarket accessories. A leadingindicator can be used as a predictor variable in regression analysis.
least squares
A method of estimating regression coefficients. This method selects values for theregression constant and coefficients so as tominimize the sum of squared residuals. See also regression and residual.
level
A feature of a time series indicating its longtermaverage value.
linear moving average A forecasting method appropriate for data with trend but without seasonality; also useful for removing seasonality from trending data. Sometimes referred to as double moving average.
M
mean
A measure of the typical level of a variable,computed as the arithmetic average of the data values stored in the variable.
measure
A numeric value to be forecasted. It might be unit sales, liters, dollars, and so on.
median
A measure of the typical level of a variable, determined by sorting the values stored in a variable from smallest to largest and identifying the middle value (if there are an odd number of data values) or the average of the two middle values (if there are an even number of data values).
moving average
A class of forecasting methods for averaging out the random variation in a data series. A moving average uses only the most recent to form the total, forecasts the total, then pro-rates the forecasts of the total down to the individual items.
N
noise
A term describing the net effect of any number of random factors that influence the value of a variable. The Decompose feature separates noise from the trend and seasonality in a variable.
outlier
A data value that is much larger or smaller than the other values of the same variable. An outlier could be caused by an error in data recording or an unusual circumstance.
P
P(2-tail)
In regression analysis, the probability that a regression coefficient would achieve its observed value or an even greater value solely by chance, if the true coefficient value were zero. A low value of P(2-tail) suggests that the coefficient is not zero. See statistically significant.
percentile
A number that equals or exceeds a specified percentage of all the values of a variable. For instance, the median is the 50th percentile, since 50% of the data values are less than or equal to the median.
point forecast
A forecast expressed as a single number, for example, sales next month will most likely be 125 units. See interval forecast.
predictor variable
In regression analysis, a variable used to forecast the dependent or forecastvariable. Also known as an independent variable. In the equation Y = 1 + 2X, the predictor variable is X.
Promotional forecasting
A special feature of SmartForecasts that combines automatic forecasting with adjustments for sales promotions and other special events that influence sales, such as blizzards and strikes.
Q
quartile
A number that divides the distribution of data values into quarters. The lower quartile is greater than 25% of the data values, the middle quartile (that is, the median) is greater than 50%, and the upper quartile is greater than 75%.
R
regression
A statistical analysis that creates an equation to convert the values of predictor or independent variables into estimates of the values of a forecast or dependent variable
residual
In regression analysis, the difference between the actual value of the forecast, or dependent variable, and its fitted value.
R-square
In regression analysis, a measure of goodness of fit between the observed and fitted values of the forecast or dependent variable. Rsquare values range from 0% to 100%, with 100% indicating a perfect fit. If the R-square is close to 0%, then your predictors are essentially worthless for forecasting purposes. See adjusted R-square
S
safety stock
An inventory buffer or investment that must be added to the most likely estimate of future demand (that is, the expected forecast) to protect against demand variablity
scatterplot
A graph of cross-sectional data, with the independent variable on the horizontal (X) axis and the dependent variable on the vertical (Y) axis. Also known as XY plot.
seasonal adjustment
A statistical procedure to cancel out a predictable pattern of monthly, quarterly, or other periodic variation in a data series. If the seasonality is multiplicative, seasonal adjustment divides the data by seasonal multipliers. If the seasonality is additive, seasonal adjustment subtracts seasonal add-factors from the data. The Decompose command performs seasonal adjustment to leave only the influences of the trend and irregular components. See decomposition
seasonal component
The repetitive cyclical influence on a time series. The Decompose command estimates the trend, seasonal, and irregular components.
seasonality
A feature of a time series characterized by repetitive cycles of high and low values; often found in quarterly data (cycles of period 4) and monthly data (cycles of period 12).
Series
A sequence of measurements or observations on a variable.
service level
The probability, expressed as a percentage, of meeting total customer demand for a particular product item during a future period out of available inventory (for example, inventory nextperiod will be sufficient to maintain a 95% or 99% customer service level). Also known as inventory service level.
service level forecasts
Forecasts of a high percentile of the distribution of demand. Usually, forecasts aim for the average or expected valueHowever, in inventory control applications, it is important to plan around the upper end of the demand distribution; this helps to minimize the occurrence of stockouts or backorders.
SIMPLE moving average
A forecasting method appropriate for data with neither trend nor seasonality; also useful for removing seasonality from data without trend.
simulation
A method of understanding the randomness in a variable by creating scenarios or possible futures. Used to estimate the distribution of lead time demand when forecasting intermittent data.
SINGLE
exponential smoothing A forecasting method appropriate for data with neither trend nor seasonality.
SKU
Abbreviation for Stock Keeping Unit, the most disaggregated level of inventory tracked in a manufacturing company.
smoothed data value
In forecasting method using exponential smoothing or moving averages, the statistical approximation to the actual value of the forecast variable.
smoothing weight
The percentage weight given to the most recent data value in exponential smoothing computations. A lower weight implies more smoothing.
sparcity
The percentage of variables containing historical data. If sparcity is eliminated when connecting a database to SmartForecasts, only rows with forecastable historical data are imported into the SmartForecasts’ data table.
standard deviation
A measure of the variability of the data values stored in a variable (computed using n-1 in the denominator).
standard error
In regression analysis, a measure of the sampling uncertainty in the value of a regression coefficient. If you were to analyze a different set of data on the same subject, you would inevitably get somewhat different regression coefficients. The standard errors show how different the regression coefficients might be. With different data, you could expect to get regression coefficients that are one or even two or more standard errors different from those computed from your present dataset.
standard error of estimate
In regression analysis, a measure of the typical size of the discrepancies between the actual data values and the values predicted by the regression equation, that is, the residuals. Also known as the root mean square error.
statistically significant
A result that is beyond the typical range of chance variability. For example, if you flip 100 fair coins, getting 53 heads and 47 tails is not a statistically significant deviation from the 50:50 ideal, since this small a discrepancy is easily explained by chance. In contrast, a 90:10 split would be statistically significant, since this large a discrepancy would almost never arise by chance if the coins were fair. In regressionanalysis, SmartForecasts tests the statistical significance of the regression coefficients against the null hypothesis that they are zero. See also P(2-tail)
T
timeplot
A graph of data values plotted against time. It is a good idea to examine a timeplot before forecasting to look for trends, seasonality, and unusual values (outliers). Also known as a line graph in Excel.
time series
An ordered succession of numbers representing the values of a particular variable over a given period of time (for example, monthly sales figures for l999). Synonymous with data series. Contrast with cross-sectional data.
time table
A table that contains textual attributes
transformation
Application of a mathematical function to a variable. For instance, log(X) is a transformation of X. Transformations are used to make a skewed distribution more symmetric or to make a nonlinear relationship more linear. The Scatterplot command can transform either or both of the X and Y variables to create a better straight line fit and then save the transformed variables in the data table. The Define command can also create and save new, transformed variables.
trend
A feature of a time series marked by more or less steady increases or decreases in the level of the series
trend component
The slow, long-term change in the level of a time series. The Decompose command estimates the trend, seasonal, and irregular components
trend percent
The average unit change per period divided by average units per period multiplied by It is available as a statistic in the describe function and for numerical filtering to identify variables with positive, negative, or zero trend and to compare average trends of one series with another.
t-statistic
In regression analysis, the ratio of a coefficient value to its standard error. SmartForecasts uses the t-statistic to compute the value of P(2-tail) when testing the hypothesis that the coefficient value would be zero except for chance variation. Roughly speaking, values of the t-statistic beyond ± 2 suggest that the corresponding predictor variable is useful for predicting the forecast variable. See P(2-tail) and statistically significant
W
weight
A parameter in an exponential smoothing method that determines the balance of influence between recent and older data values. Higher values for weights give more influence to more recent data values. This permits faster adjustment to changing conditions at the cost of less smoothing of noise when conditions are stable.
WINTERS’ exponential smoothing
A forecasting method appropriate for data with both trend and seasonality; both additive and multiplicative forms of Winters’ method are available in SmartForecasts.
Article Archives
Smart Software is excited to announce that we are joining Epicor, a global leader of industry-specific enterprise software. The acquisition brings together two companies tightly aligned in helping organizations get to the right insights at the right time and take action to maximize business performance.
In joining Epicor, Smart Software customers will benefit from significant scale, development, and investment in our inventory planning and optimization solutions, over time giving you even more capabilities and product options. In acquiring Smart Software, Epicor is complementing and strengthening its portfolio of best-in-class ERP solutions, helping makers, movers, and sellers worldwide streamline and simplify their supply chains to gain a competitive advantage. As your strategic business partner, our top priority as we integrate the organizations in the coming months is to continue to provide you with the highest level of service and support you expect.
For more information on the news, please visit the Epicor Newsroom
Smart Software is pleased to announce the award of US Patent 11,656,887. “System And Method to Simulate Demand and Optimize Control Parameters for a Technology Platform.”
The patent directs “technical solutions for analyzing historical demand data of resources in a technology platform to facilitate management of an automated process in the platform.” One important application is optimization of parts inventories.
Smart Software named a Microsoft Co-sell-ready partner as a leading demand planning and inventory optimization solutions provider. Microsoft customers leverage Smart’s web-native platform for Inventory Planning and Optimization (Smart IP&O) to develop consensus forecasts, manage demand, and optimize stocking policies.
We are so grateful to announce Smart Software’s 40th anniversary. Forty years of Innovation for Demand Forecasting, Inventory Planning, and Supply Chain Analytics. Smart, Hartunian, and Willemain was incorporated in June 1981 by Charles Smart, Thomas Willemain, and Nelson Hartunian, our visionary founders.
Supply and Demand Chain Executive 2020 Online Magazine features an article about inventory optimization at BC Transit, entitled “Optimizing Parts Management at BC Transit.” Eric Nelson, Director for Supply Services at BC Transit explains how Smart IP&O has helped ensure that they have the right part in the right place at the right time to equip their entire service network with 35 repair locations.
Don’t Be a Victim of your Forecast Models – Special Section in Foresight Magazine. In this feature section, Tom Willemain provokes a good deal of thought about the role of statistical models in supply chain forecasting, a field that he believes lags far behind finance in embracing algorithms over gut instinct. Download Special Section on Forecast Modeling in Spring 2019 Issue of Foresight Magazine
Right-Sizing Inventory with Demand Forecasting,” interview with Chief Material Officer at Metro-North Railroad.
Supply & Demand Chain Executive — June 26
See “Four Steps to Service Level-Driven Inventory Planning” in SupplyChain Management Review.
SupplyChain Management Review — April 12, 2015
Minnesota’s Metro Transit adopts service-level driven method of inventory planning from Smart Software.
Mass Transit Magazine — February 2015
Video Interview with SupplyChainBrain, “Service Level-Driven Demand Planning”
SupplyChainBrain — June 30, 2014
Conquering Supply Chain Variability at Rev-A-Shelf,” in Supply & Demand Chain Executive Online
Supply & Demand Chain Executive — April 9, 2014
Now You See It, Now You Don’t Inventory,” New technologies to address intermittent demand, in Aviation Week Online
Aviation Week — April 7, 2014
Webinar Replay: Agile Demand Forecasting, the Rev-A-Shelf Experience.
SmartSoftware Inc. — January 2014
Feature Article: MCI’s Parts Planning Excels with Smart Software” in BusRide Maintenance Magazine
BusRide Maintenance Magazine — September 2013
Webinar replay: CFO View of Demand Forecasting, with Bud Schultz, NKK Switches. Originally broadcast 9/26/2013.
SmartSoftware Inc. — September 2013
Featured Review: “Smart Software for Service-level Driven Forecasting” by Technology Evaluation Centers.
Technology Evaluation Centers — May 2013
Feature Article: “Forecasting – It’s More than Inventory Management,” in Supply & Demand Chain Executive.
Supply & Demand Chain Executive — February 2013
Feature Article: “Small Operational Changes Bring Big Cost-Cutting Results for Transit Agencies” in APTA Passenger Transport Magazine
APTA Passenger Transport Magazine — June 2012
Feature Article: “Meeting the Service Parts Inventory Planning Challenge” in BUSride Maintenance Magazine
BUSride Maintenance Magazine — April 2012
SmartForecasts Review: “Smarter Inventory Management: Forecasting public transportation needs with the complete software package” in APICS Magazine
APICS magazine — January/February 2012
Transit Talk Blog: “How to Discover Hidden Cost Savings in Your Transit Operations”
Mass Transit Magazine — September, 2011
Cover Story: A Northeast Commuter Railroad Struggles to Optimize Its Spare Parts Inventory
SupplyChainBrain — May/June 2011
“Prestolite Charges up Its Inventory Management”
When retirements drained its planning expertise and a bad mix of too much inventory put a strain on cash flow, the company brought in a new forecasting tool to power its planning process. S&DCE Aug/Sep 2010
“How to Survive the Economic Crunch”
Current economic conditions are challenging everyone’s business. This is especially true for companies in the manufacturing and distribution sectors that have a large percentage of their supply chain resources tied up in inventory. With credit tight, these companies are looking for ways to preserve as much cash as possible. Because inventory is cash sitting on the shelves, a prime way to reduce costs and increase cash flows is to improve the forecasting and planning of inventory requirements. SDCExec.com May 2009
“Smooth Ride: Forecasting solution improves product deployment and reduces transportation costs”
See how Prevost Parts, a division of Canadian bus manufacturer Prevost Car, has used SmartForecasts to improve inventory allocation and distribution effectiveness in the North American aftermarket for its 25,000 motorcoach and urban bus parts. Learn about Prevost Parts’ evaluation criteria in selecting SmartForecasts over five other applications considered, and the importance of Smart’s unique intermittent demand forecasting capability in solving Prevost’s forecasting and safety stock estimation problems. APICS May 2005
“Prevost Parts increases replacement part availability and reduces inventory through improved demand forecasting” by Alex Daudelin.
Read an article about how Prevost Parts, the parts division of Canadian bus manufacturer Prevost Car, uses SmartForecasts to more effectively distribute replacement parts for the North American motorcoach and transit bus markets. Learn why the company selected SmartForecasts over SAP’s demand planning application and now expects both a 25% increase in forecast accuracy and close to a 20% reduction in inventory stocking levels. Geston Logistique March 2004
“The Right Tools” by Charles Smart, president of Smart Software.
Learn how the right statistical forecasting tools combined with collaborative inputs from management, salespeople and customers can greatly improve forecast accuracy and streamline a company’s demand planning process. APICS May 2003
“Forecasting-As Important as Ever” by Charles Smart, president of Smart Software.
Read why demand forecasting is still essential in this new manufacturing age. APICS Nov/Dec 2001
“A better crystal ball” by Charles Smart, president of Smart Software.
Read a Web-exclusive article about how forecasting applications are adapting to shorter product life cycles and build-to-order trends. manufacturingsystems.com Nov 2001
“Bootstrap Your Way to Better Forecasts” by Charles Smart, president of Smart Software.
Learn about Smart Software’s new forecasting method to address the multi-million dollar problem of planning intermittent, slow-moving demand. Midrange Enterprise Jan 2001
Contact Us Today for More Information
If you request a demo, one of our specialists will show you how Smart can help, using your own inventory data!