In order to reap the efficiency benefits of forecasting, you need the most accurate forecasts—forecasts built on the most appropriate historical data. Most discussions of this issue tend to focus on the merits of using demand vs. shipment history—and I’ll comment on this later. But first, let’s talk about the use of net vs. gross data.
Net vs. Gross History
Many planners are inclined to use net sales data to create their forecasts. Systems that track sales capture transactions as they occur and aggregate results into weekly or monthly periodic totals. In some cases, sales records account for returned purchases as negative sales and compute a net total. These net figures, which often mask real sales patterns, are fed into the forecasting system. The historical data used actually presents a false sense of what the customer wanted, and when they wanted it. This will carry forward into the forecast, with less than optimal results.
Suppose your sales data is aggregated into monthly totals. If monthly net figures are used, and returns occur in the same month that they are purchased, no problem. The net activity for the month reflects actual demand. But what if the product is returned three or four months after it was purchased, as often occurs? The net figures are underestimate interest in the product for the month when the return is counted, and (it would seem) overestimate demand for the month of the initial sale. Reflecting product demand at the wrong time in this way interferes with the forecasting system’s ability to properly identify the pattern (or lack thereof) in the data.
Since you fill orders you as you get them, you are hardly going to decline to ship because you think they might return the product in a few months. Customers wouldn’t stand for that. So of course you want to know that such demand is likely to occur. If the orders are going to come, you need stock in your inventory to meet the demand, regardless of later returns. Using net figures is not the best practice in these situations.
In most cases, data used in preparing forecasts needs to be based on gross figures. Returns can be forecast as a separate variable and used as a source of incoming supply. This will result in fewer unnecessary orders of new inventory. Ultimately, this approach better captures the true patterns in the data—the heart of forecasting.
Shipment vs. Demand Data
A second important distinction regarding historical data may seem counter-intuitive. Forecasters are nearly always encouraged to use demand data because it is assumed to better reflect what the customer wanted and when. However, when you have the choice, it can sometimes be smarter to use shipment data.
One guiding principle is to consider the accuracy of the data. In most businesses, the shipment data accurately reflects exactly what was shipped, while the demand data is often plagued with inaccuracies. Here are a few examples:
Customers may know, for example, that you will be unable to fill their order. In that case, they may refrain from placing the order, holding off until they believe you have stock on hand to meet their needs. On the other hand, anxious customers may file multiple electronic orders, concerned that some miscommunication could prevent a vital order from being processed. When assured that one order has been accepted, they may cancel the additional orders. Then there is the case of a customer that has experienced stockouts with you in the past. They may increase the size of their orders to maximize the chance that inventory will be allocated to them.
If such practices plague your order history, it may be more prudent to use shipping data. You can then use SmartForecasts’ “adjust history” feature to fine tune the shipment data—so it better reflects demand. You can certainly do the same on inaccurate demand data but often this will require much more effort than simply correcting for obvious cases where shipments are low one month and high the next due to a stock-out situation.
Before making a decision on whether to use shipment or demand data, it is important to understand how orders and returns are accounted for in your system. Talk to your customer service reps and ask them about how they would account for these situations. Don’t take your IT department’s word for it. Exercise your judgment to get the best results.
Making the right data choices will go a long way toward achieving valuable supply chain efficiencies. Take the time to read Michael Gilliland’s post on this topic at the Institute of Business Forecasting and Planning’s blog, at demand-planning.com.
Gregory Hartunian serves as President of Smart Software and as a member of the Board of Directors. He previously held the position of Vice President of Sales.
Related Posts
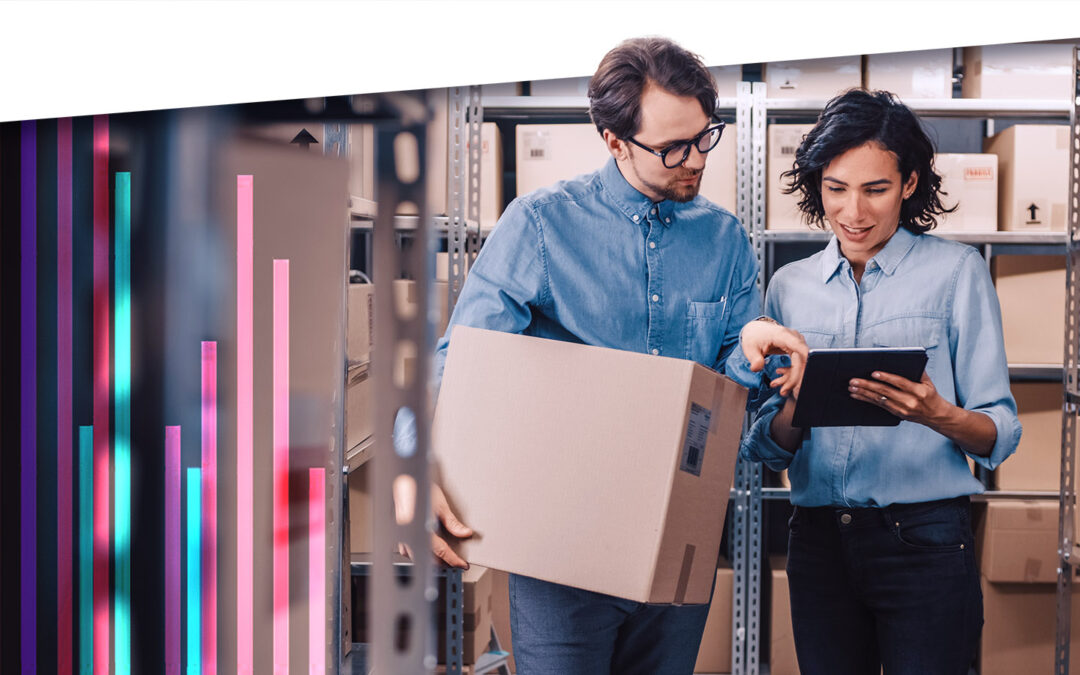
Forecast-Based Inventory Management for Better Planning
Forecast-based inventory management, or MRP (Material Requirements Planning) logic, is a forward-planning method that helps businesses meet demand without overstocking or understocking. By anticipating demand and adjusting inventory levels, it maintains a balance between meeting customer needs and minimizing excess inventory costs. This approach optimizes operations, reduces waste, and enhances customer satisfaction.
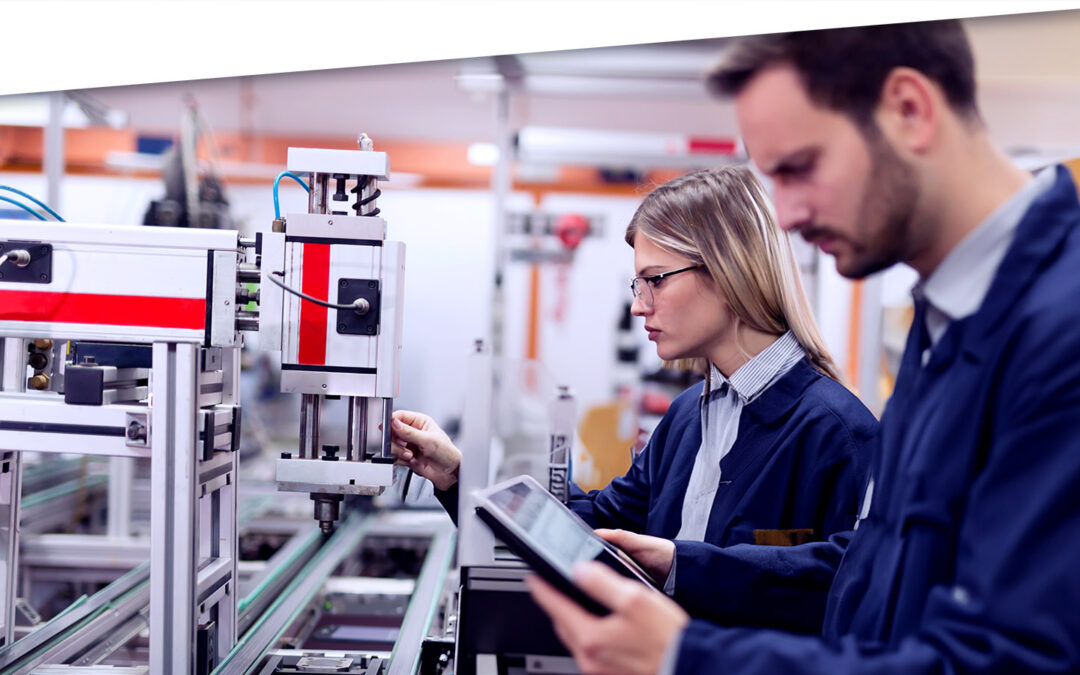
Leveraging Epicor Kinetic Planning BOMs with Smart IP&O to Forecast Accurately
In this blog, we explore how leveraging Epicor Kinetic Planning BOMs with Smart IP&O can transform your approach to forecasting in a highly configurable manufacturing environment. Discover how Smart, a cutting-edge AI-driven demand planning and inventory optimization solution, can simplify the complexities of predicting finished goods demand, especially when dealing with interchangeable components. Learn how Planning BOMs and advanced forecasting techniques enable businesses to anticipate customer needs more accurately, ensuring operational efficiency and staying ahead in a competitive market.
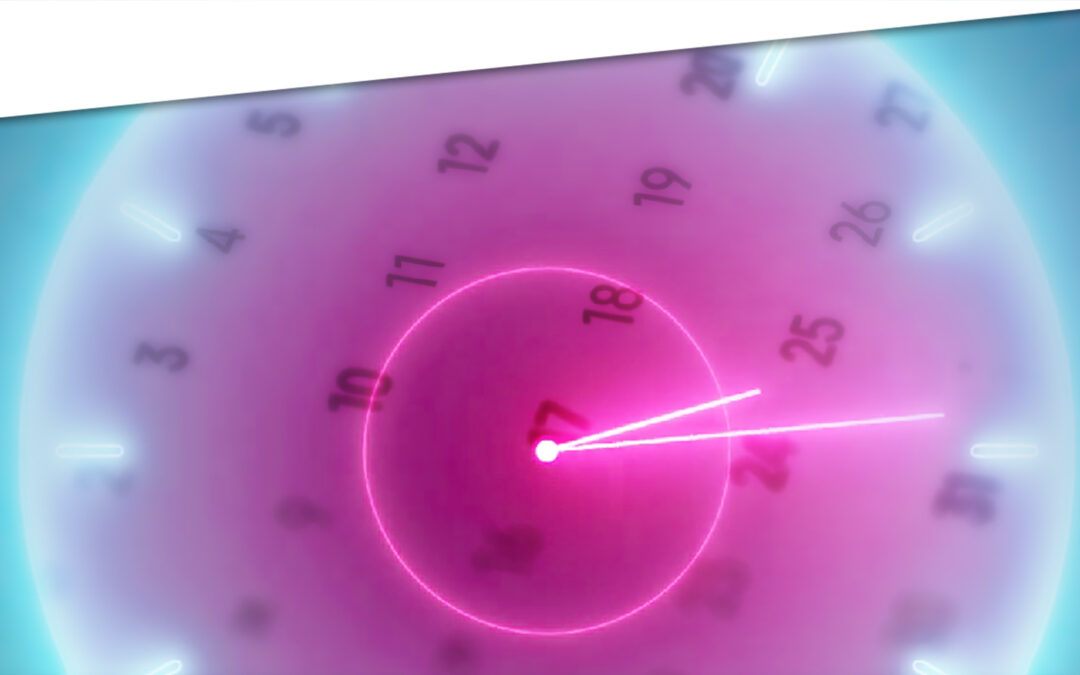
Daily Demand Scenarios
In this Videoblog, we will explain how time series forecasting has emerged as a pivotal tool, particularly at the daily level, which Smart Software has been pioneering since its inception over forty years ago. The evolution of business practices from annual to more refined temporal increments like monthly and now daily data analysis illustrates a significant shift in operational strategies.