Managing inventory requires executives to balance competing goals: high product availability versus low investment in inventory. Executives strike this balance by stating availability targets and budget constraints. Then supply chain professionals translate these “commander’s intentions” into detailed specifications about reorder points and order quantities.
A High-Stakes Race Between Supply and Demand
Let’s focus on reorder points (also known as mins). They work as follows. As on-hand inventory decreases in response to demand, it eventually drops down to or below a trigger value, the reorder point or min. At that point, it’s like a gun goes off to start a race between supply and demand. A replenishment order is sent to restock the item, but there is a replenishment lead time, so the restocking is not instantaneous. While your system waits for resupply, demand continues to whittle away at the stock on hand. It is bad news if demand wins the race, because then you won’t be in position to provide what somebody is demanding. Then they either get it from a competitor or get back-ordered and unhappy: either way, stocking out is a bad outcome for you and your customer.
The risk of stocking is controlled by your staff’s choice of reorder points. If they are set too high, stock-outs are rare but inventory is bloated. Set them too low and stock-outs abound. So how should reorder points be set?
Avoiding Foolish Follow-Through
Several factors govern stock-out risk. Each item in your inventory has its own demand history and lead time. Together with your chosen availability targets, these factors determine the best choice of reorder point. But the relationships are statistical and require good analysis to work out. Inventory Optimization Software can compute the proper reorder point for each of tens of thousands of items. But instead of relying on proper analysis, many companies fall back on simple rules of thumb or just “doing what we always do”.
In place of using the right math, companies often rely on rules of thumb that serve them poorly. Here are some examples in order of most common to least common.
1) Multiples of Average Demand
Setting reorder points at some (arbitrary) multiple of average demand starts to rely on actual facts. But it ignores the key demand attribute that drives stock-out risk: demand variability. Two items with the same average demand but very different levels of variability will require very different reorder points to insure the same low risk of stock-out. (See Figure 1)
2) Gut feel
Some companies have self-styled supply chain gurus. Even if they actually are Jedi masters, it’s impossible to keep up with tens of thousands of items whose reorder points should be reviewed frequently. And if the logic that drives decision making is buried in a hard to use spreadsheet that only they know how to use, the company risks not being able to execute the inventory plan without that one individual –a risky proposition.
3) Average Demand + some multiple of Demand Variability
This approach is taught in many “Inventory 101” courses. But it implicitly assumes some facts about demand that are very often not true: that demand has a Normal (“bell-shaped”) distribution and that demand in one period does not relate to demand in the previous time period(s). Assumptions of independence and reliance on normal distribution models just don’t cut it.
4) Nursery rhymes
Not at all the norm, hence being last on the list, but we heard of one company that used one simple rule for all items: “If it’s down to four, order more”. It’s crazy to believe that one rule applies to all items at all times. But at least it rhymes.
Your people can do better than to rely on any of these approaches. Do you know whether your company is using any one of them?
Getting It Right
The right way to set reorder points uses the tools of probability theory. The details depend on whether you are selling finished goods or spare parts. Spare parts are usually more difficult to manage because they have quirky demand patterns: high intermittency (lots of zero demands), high skewness (lots of small demands but with some whoppers too), and auto-correlation (“feast or famine” behavior). Modern Reorder Point Software takes these quirks into account to set reorder points that insure the desired level of item availability. Importantly, they also let your people see explicit trade-off curves, so they can strike the balance you want — at the item by location level – between stock-out risk and inventory investment.
Inventory is a major item on the balance sheet and needs high-level attention. At many manufacturers, service parts can represent up to half of revenue. Modern software lets the C-Suite move beyond, incomplete math and other inadequate approaches to managing inventory.
Figure 1: Two equally important items with the same average demand get assigned the same stocking policy that determines the Min (reorder point) as 2 x average lead time demand. Despite the “same” stocking policy service performance varies significantly with the stable Item A experiencing overstocks and the volatile Item B experiencing stock outs.
Related Posts
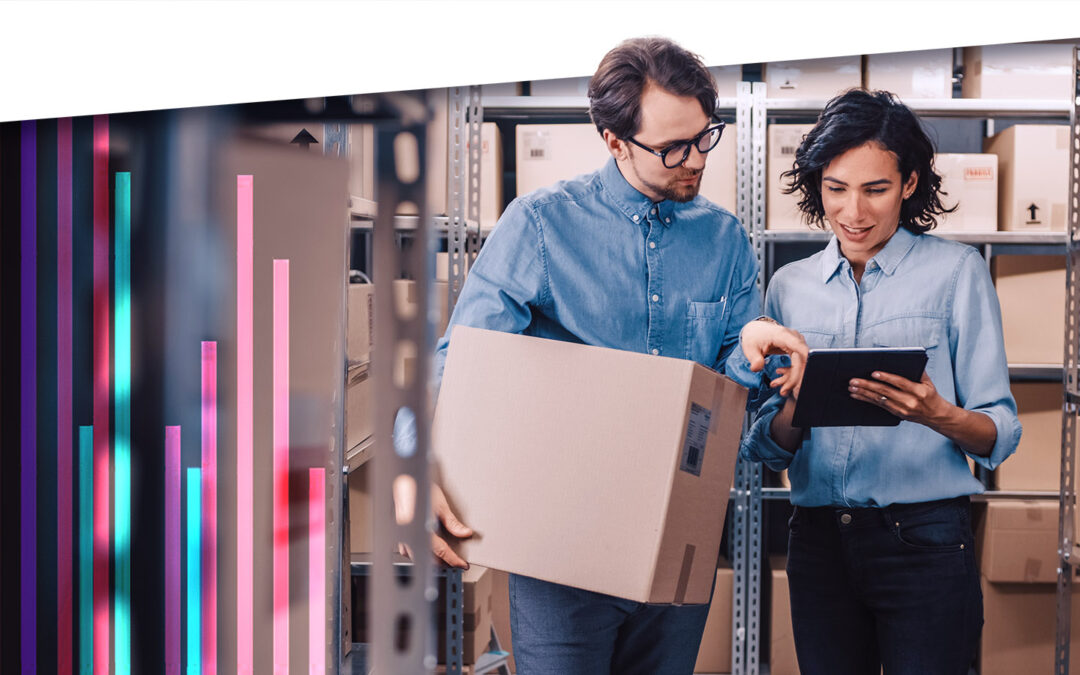
Forecast-Based Inventory Management for Better Planning
Forecast-based inventory management, or MRP (Material Requirements Planning) logic, is a forward-planning method that helps businesses meet demand without overstocking or understocking. By anticipating demand and adjusting inventory levels, it maintains a balance between meeting customer needs and minimizing excess inventory costs. This approach optimizes operations, reduces waste, and enhances customer satisfaction.

Future-Proofing Utilities: Advanced Analytics for Supply Chain Optimization
Utilities in the electrical, natural gas, urban water, and telecommunications fields are all asset-intensive and reliant on physical infrastructure that must be properly maintained, updated, and upgraded over time. Maximizing asset uptime and the reliability of physical infrastructure demands effective inventory management, spare parts forecasting, and supplier management. A utility that executes these processes effectively will outperform its peers, provide better returns for its investors and higher service levels for its customers, while reducing its environmental impact.
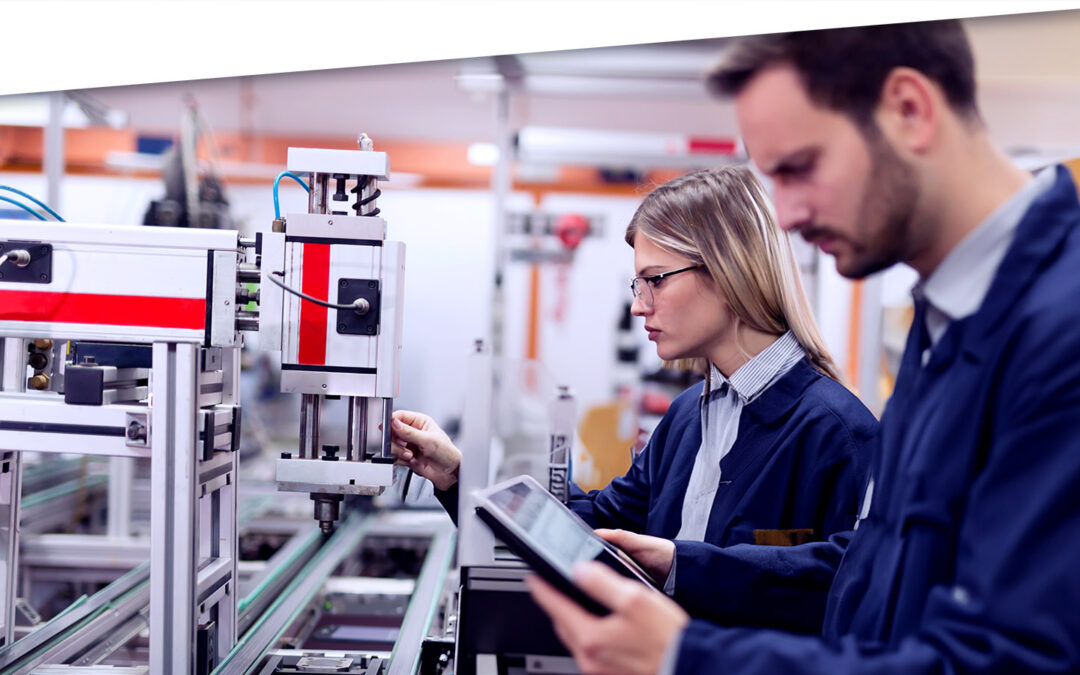
Leveraging Epicor Kinetic Planning BOMs with Smart IP&O to Forecast Accurately
In this blog, we explore how leveraging Epicor Kinetic Planning BOMs with Smart IP&O can transform your approach to forecasting in a highly configurable manufacturing environment. Discover how Smart, a cutting-edge AI-driven demand planning and inventory optimization solution, can simplify the complexities of predicting finished goods demand, especially when dealing with interchangeable components. Learn how Planning BOMs and advanced forecasting techniques enable businesses to anticipate customer needs more accurately, ensuring operational efficiency and staying ahead in a competitive market.