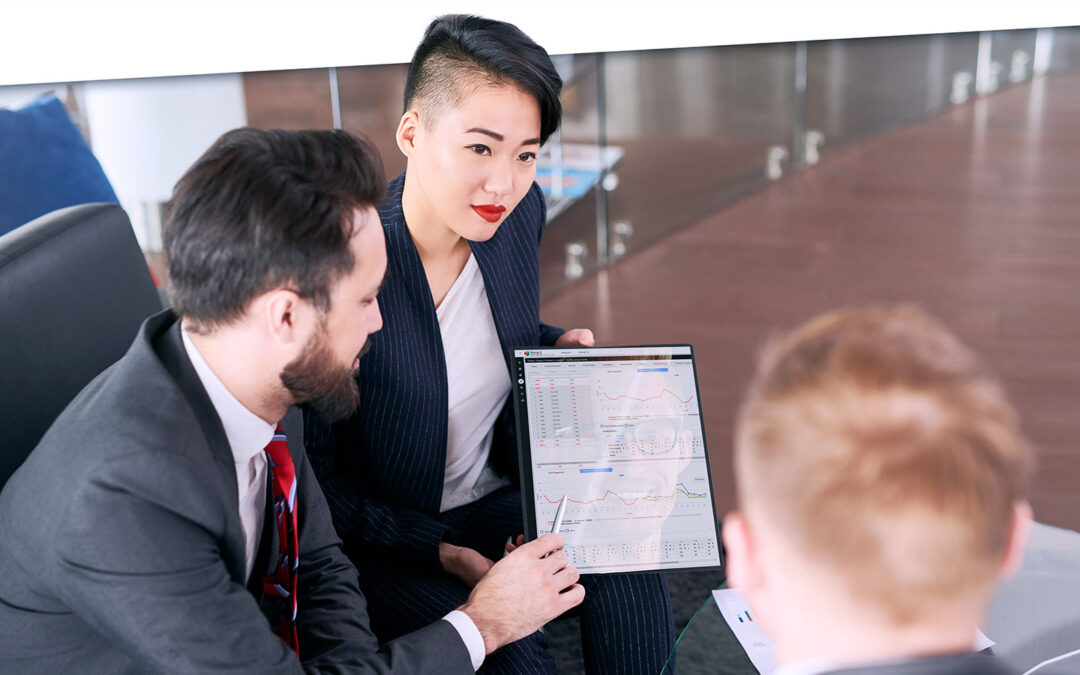
6 Observations About Successful Demand Forecasting Processes
Forecasting is both an art and a science, requiring a balance between professional judgment and objective statistical analysis. In this blog, we will explore how to generate accurate predictions by leveraging statistical methods, incorporating business knowledge, and enhancing credibility through refinement and graphical representation. Learn about aligning techniques with data nature and integrating them with other business processes, creating a comprehensive planning approach that acknowledges margin of error and forecast bias. Gain the principles and techniques for successful demand forecasting, empowering informed decision-making and optimized planning.
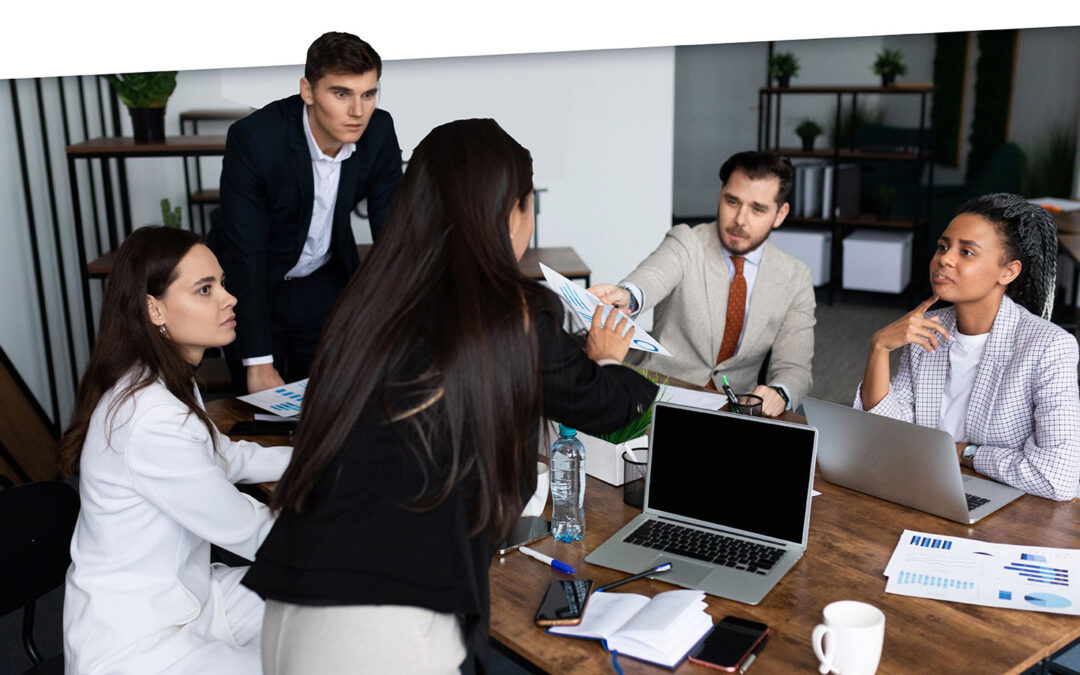
Don’t Blame Excess Stock on “Bad” Sales / Customer Forecasts
Sales forecasts are often inaccurate simply because the sales team is forced to give a number even though they don’t really know what their customer demand is going to be. Let the sales teams sell. Don’t bother playing the game of feigning acceptance of these forecasts when both sides (sales and supply chain) know it is often nothing more than a WAG.
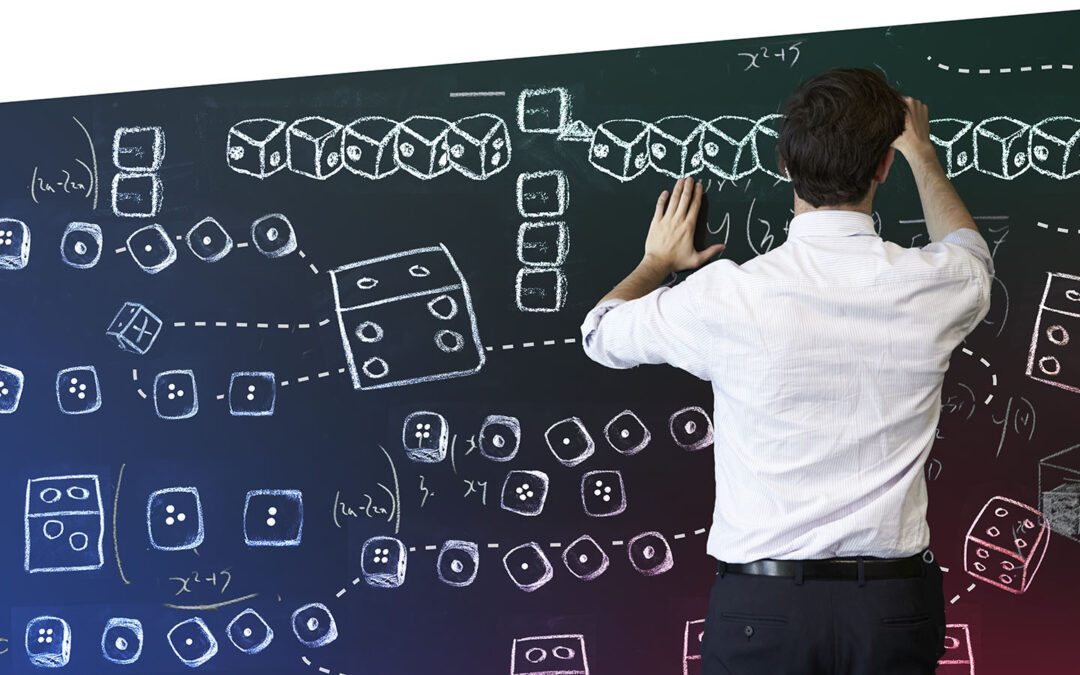
What makes a probabilistic forecast?
What’s all the hoopla around the term “probabilistic forecasting?” Is it just a more recent marketing term some software vendors and consultants have coined to feign innovation? Is there any real tangible difference compared to predecessor “best fit” techniques? Aren’t all forecasts probabilistic anyway?
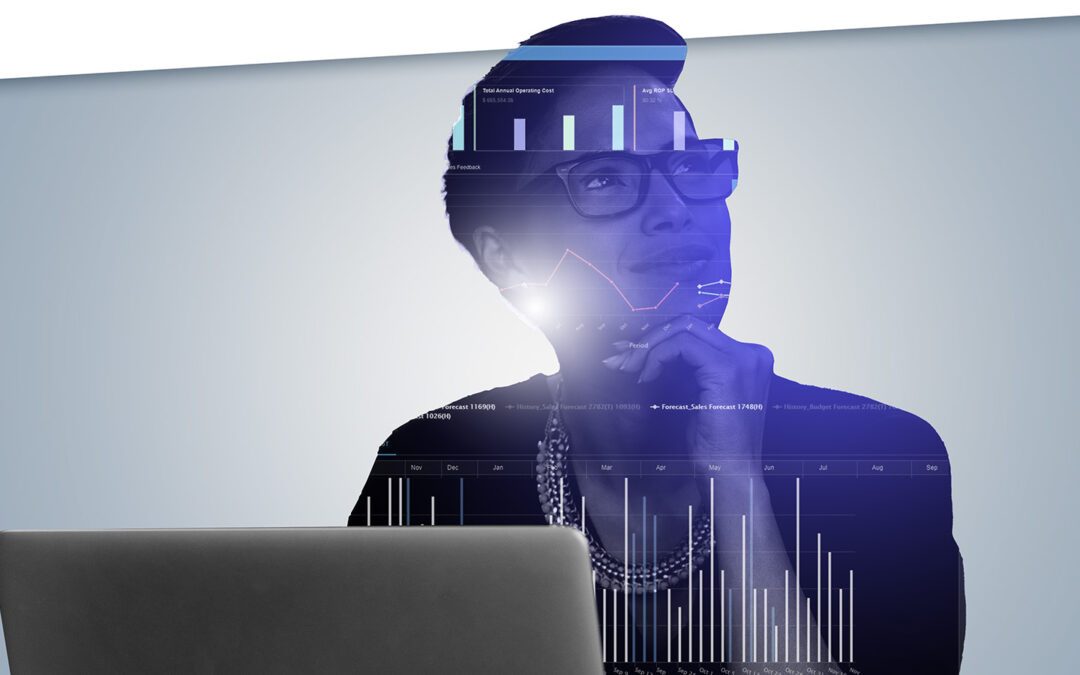
A Practical Guide to Growing a Professional Forecasting Process
Many companies looking to improve their forecasting process don’t know where to start. It can be confusing to contend with learning new statistical methods, making sure data is properly structured and updated, agreeing on who “owns” the forecast, defining what ownership means, and measuring accuracy. Having seen this over forty-plus years of practice, we wrote this blog to outline the core focus and to encourage you to keep it simple early on.
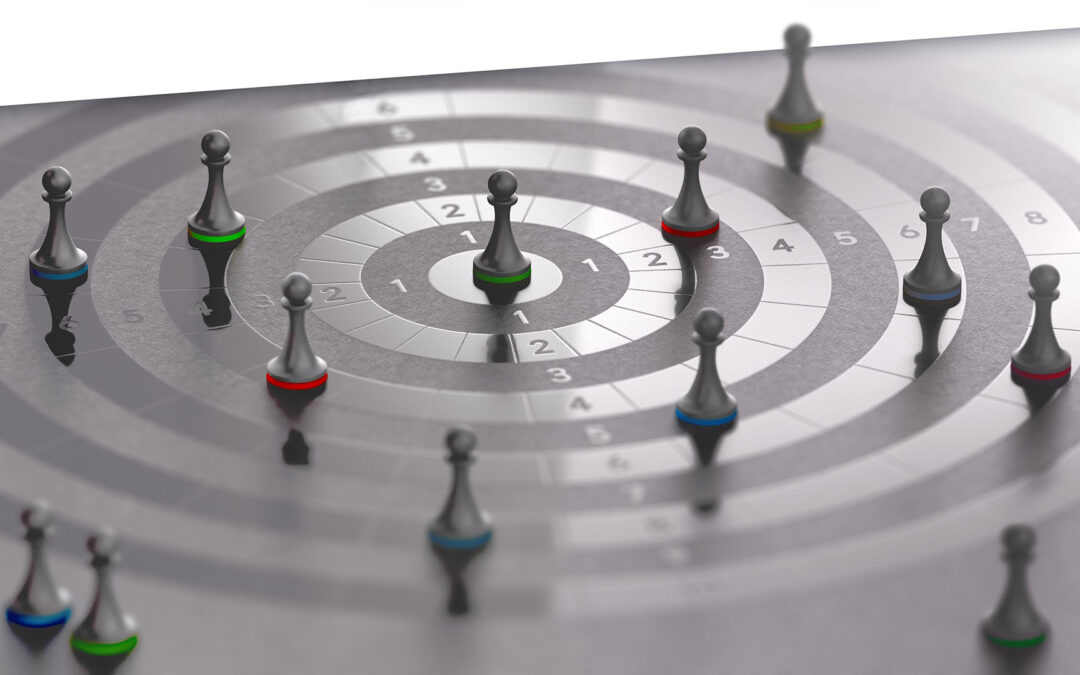
Everybody forecasts to drive inventory planning. It’s just a question of how.
Often companies will insist that they “don’t use forecasts” to plan inventory. They often use reorder point methods and are struggling to improve on-time delivery, inventory turns, and other KPIs. While they don’t think of what they are doing as explicitly forecasting, they certainly use estimates of future demand to develop reorder points such as min/max.
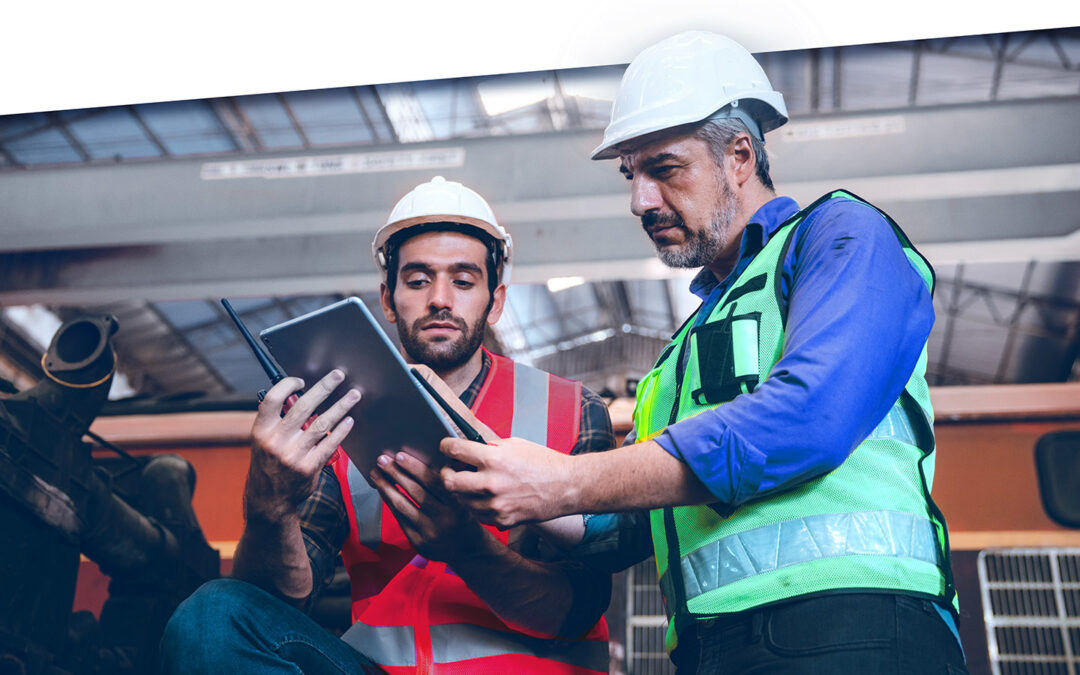
Prepare your spare parts planning for unexpected shocks
In today’s unpredictable business climate, we do have to worry about supply chain disruptions, long lead times, rising interest rates, and volatile demand. With all these challenges, it’s never been more vital for organizations to accurately forecast parts usage, stocking levels, and to optimize replenishment policies such as reorder points, safety stocks, and order quantities. In this blog, we’ll explore how companies can leverage innovative solutions like inventory optimization and parts forecasting software that utilize machine learning algorithms, probabilistic forecasting, and analytics to stay ahead of the curve and protect their supply chains from unexpected shocks.
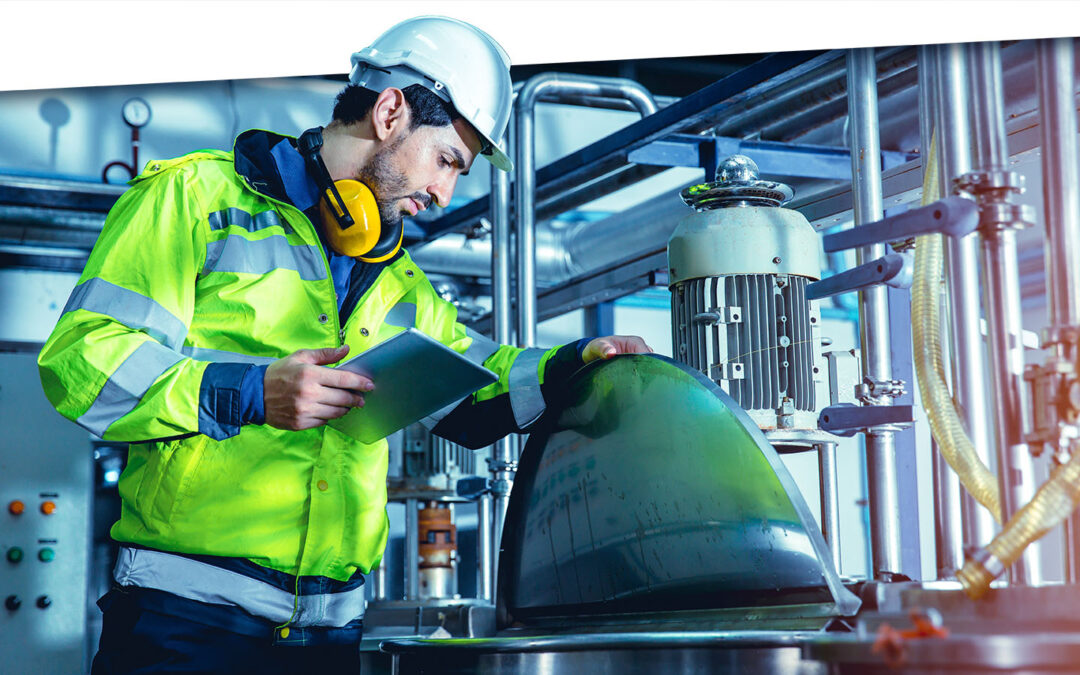
Why Spare Parts Tradeoff Curves are Mission-Critical for Parts Planning
When managing service parts, you don’t know what will break and when because part failures are random and sudden. As a result, demand patterns are most often extremely intermittent and lack significant trend or seasonal structure. The number of part-by-location combinations is often in the hundreds of thousands, so it’s not feasible to manually review demand for individual parts. Nevertheless, it is much more straightforward to implement a planning and forecasting system to support spare parts planning than you might think.
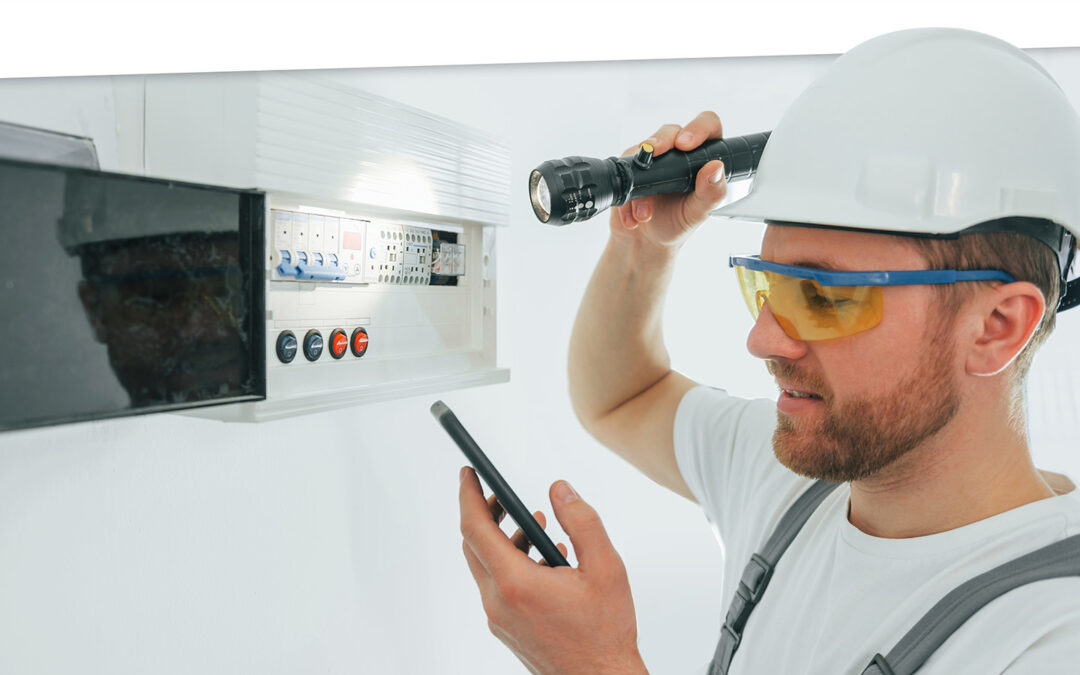
Electric Utilities’ Problems with Spare Parts
Every organization that runs equipment needs spare parts. All of them must cope with issues that are generic no matter what their business. Some of the problems, however, are industry specific. This post discusses one universal problem that manifested in a nuclear plant and one that is especially acute for any electric utility.
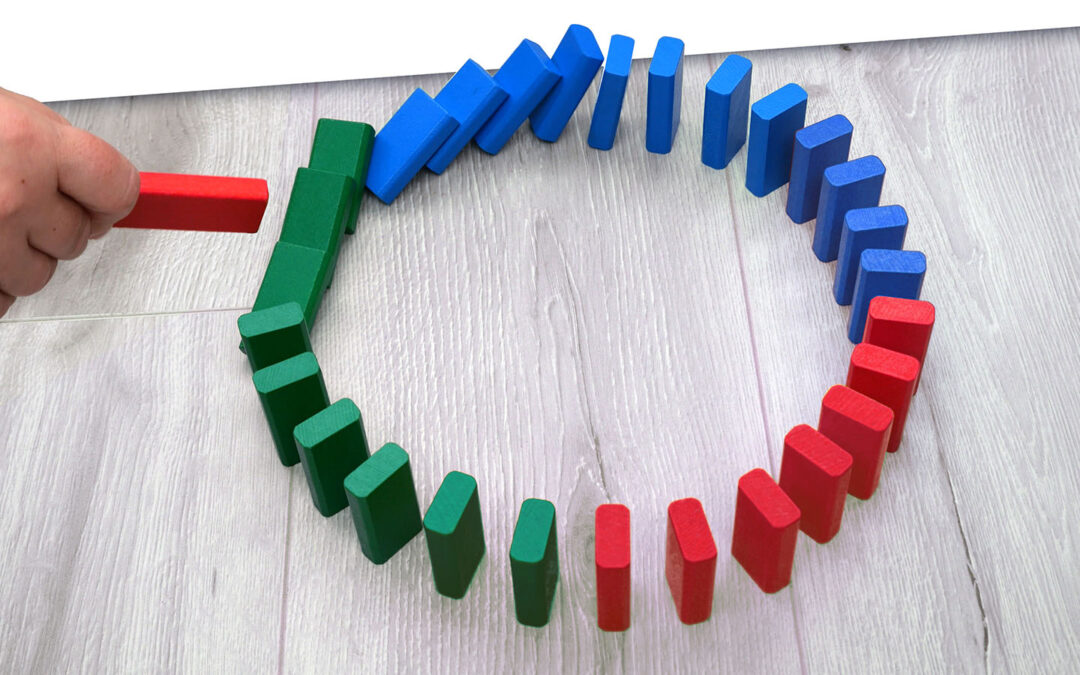
Correlation vs Causation: Is This Relevant to Your Job?
Outside of work, you may have heard the famous dictum “Correlation is not causation.” It may sound like a piece of theoretical fluff that, though involved in a recent Noble Prize in economics, isn’t relevant to your work as a demand planner. Is so, you may be only partially correct.
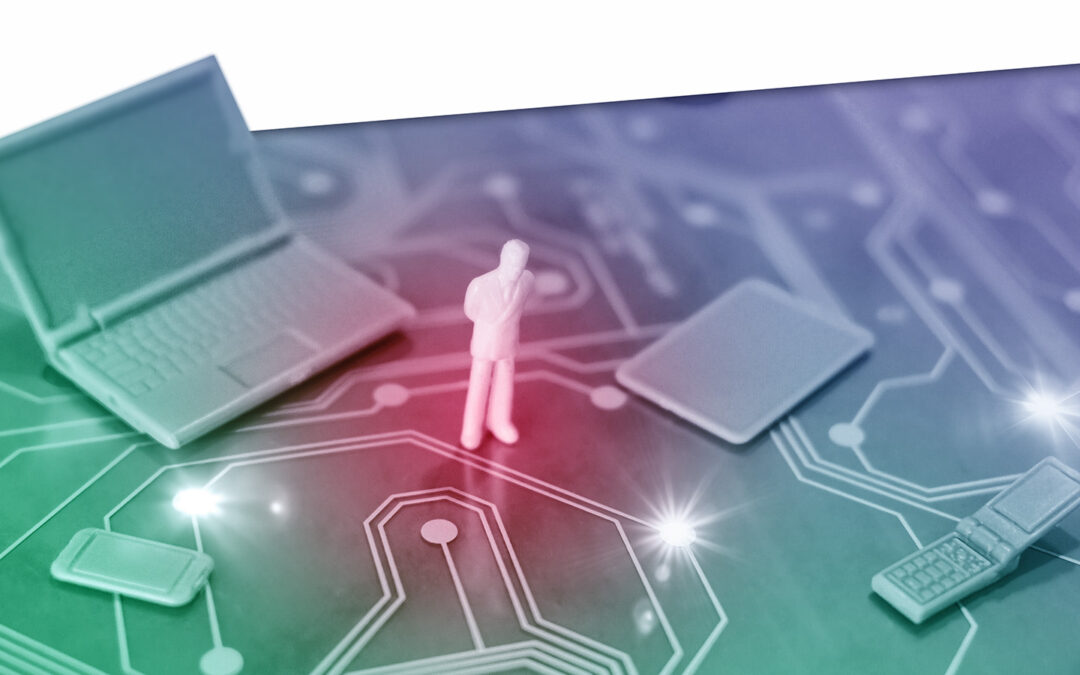
What data is needed to support Demand Planning Software Implementations
We recently met with the IT team at one of our customers to discuss data requirements and installation of our API based integration that would pull data from their on-premises installation of their ERP system. The IT manager and analyst both expressed significant concern about providing this data and seriously questioned why it needed to be provided at all.
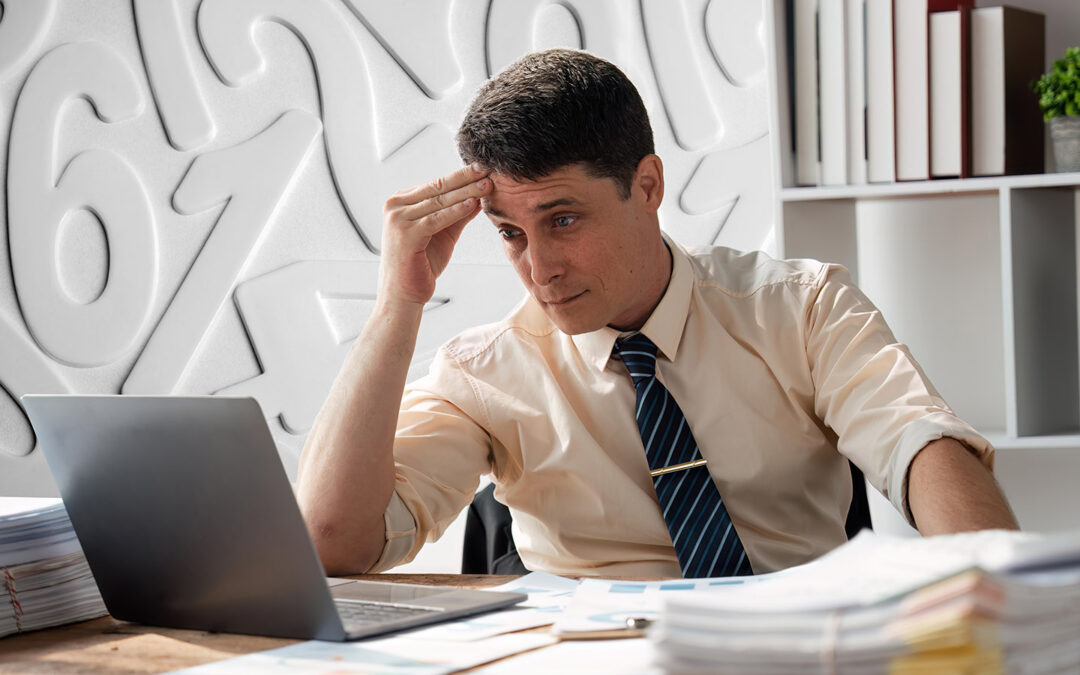
Types of forecasting problems we help solve
Generating accurate statistical forecasts isn’t an easy task. Planners need to keep historical data continually up to date, build and manage a database of forecasting models, know which forecast methods to use, keep track of forecast overrides, and report on forecast accuracy. Here are examples of forecasting problems that SmartForecasts can solve, along with the kinds of business data representative of each.
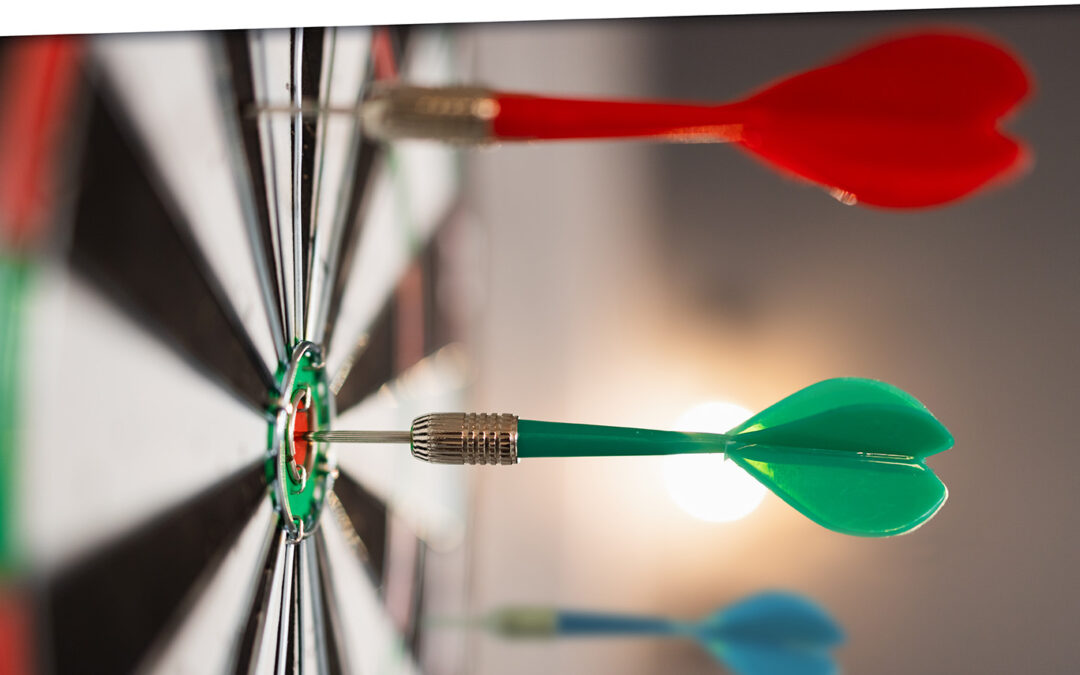
Three Ways to Estimate Forecast Accuracy
Forecast accuracy is a key metric by which to judge the quality of your demand planning process. Once you have forecasts, there are several ways to summarize their accuracy, usually designated by obscure three- or four-letter acronyms like MAPE, RMSE, and MAE.
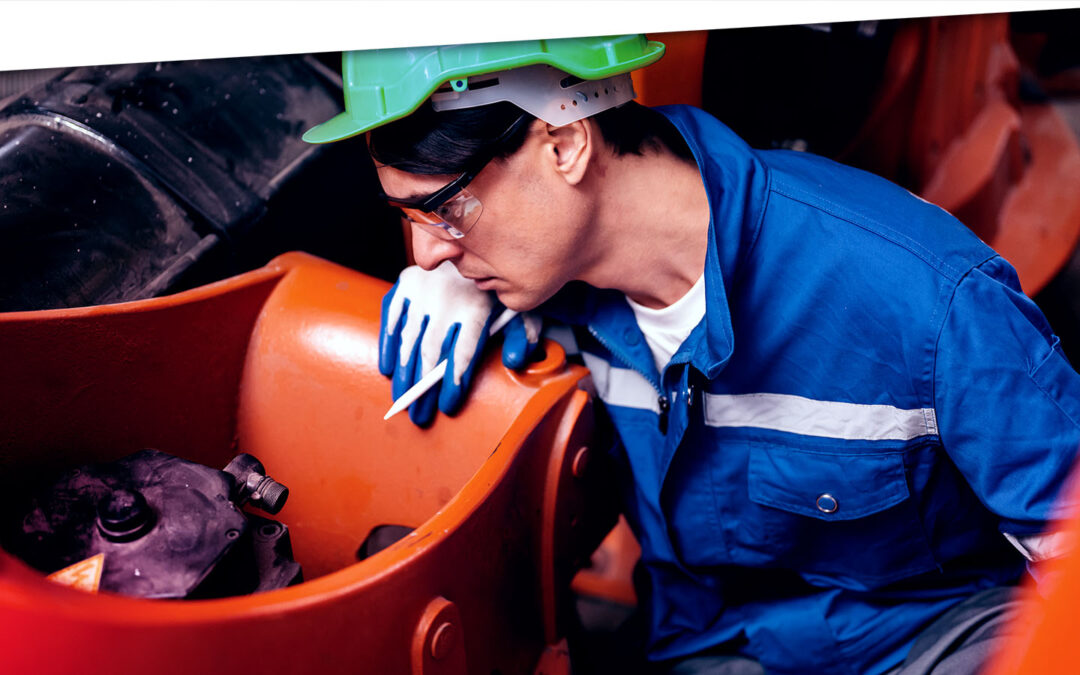
How to Forecast Spare Parts with Low Usage
What do you do when you are forecasting an intermittently demanded item, such as a spare part, with an average demand of less than one unit per month? Most of the time, the demand is zero, but the part is significant in a business sense; it can’t be ignored and must be forecasted to be sure you have adequate stock.
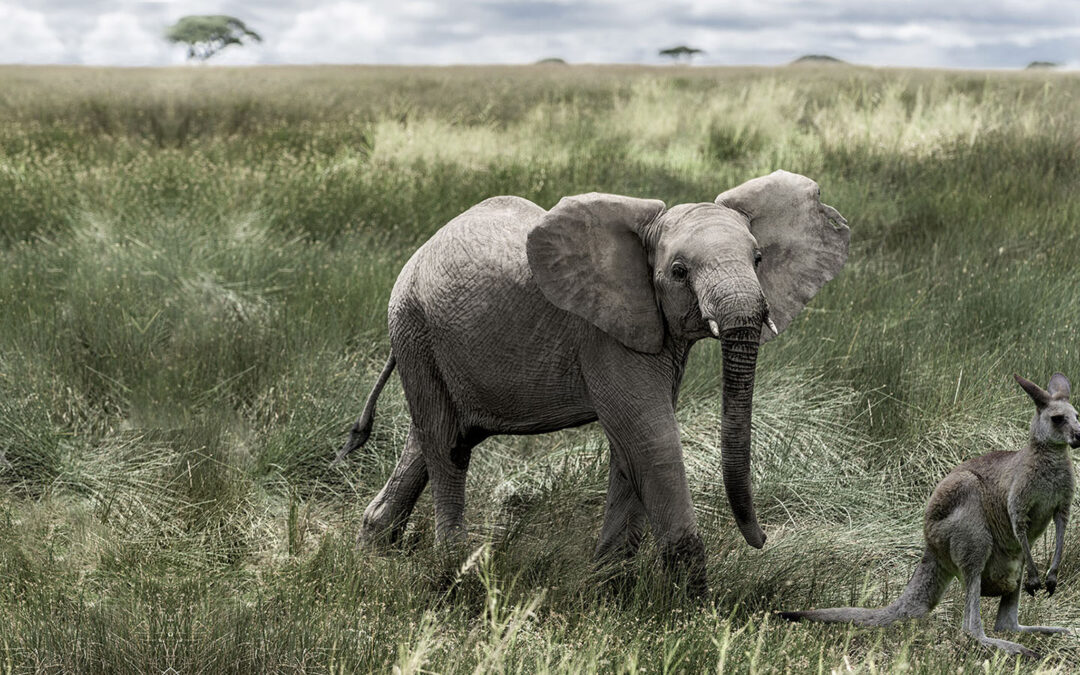
Elephants and Kangaroos ERP vs. Best of Breed Demand Planning
The largest ERP companies can’t develop high quality best-of-breed like solutions. They never had to, so they never evolved to innovate outside their core focus. However, as ERP systems have become commoditized, gaps in their functionality have become impossible to ignore.
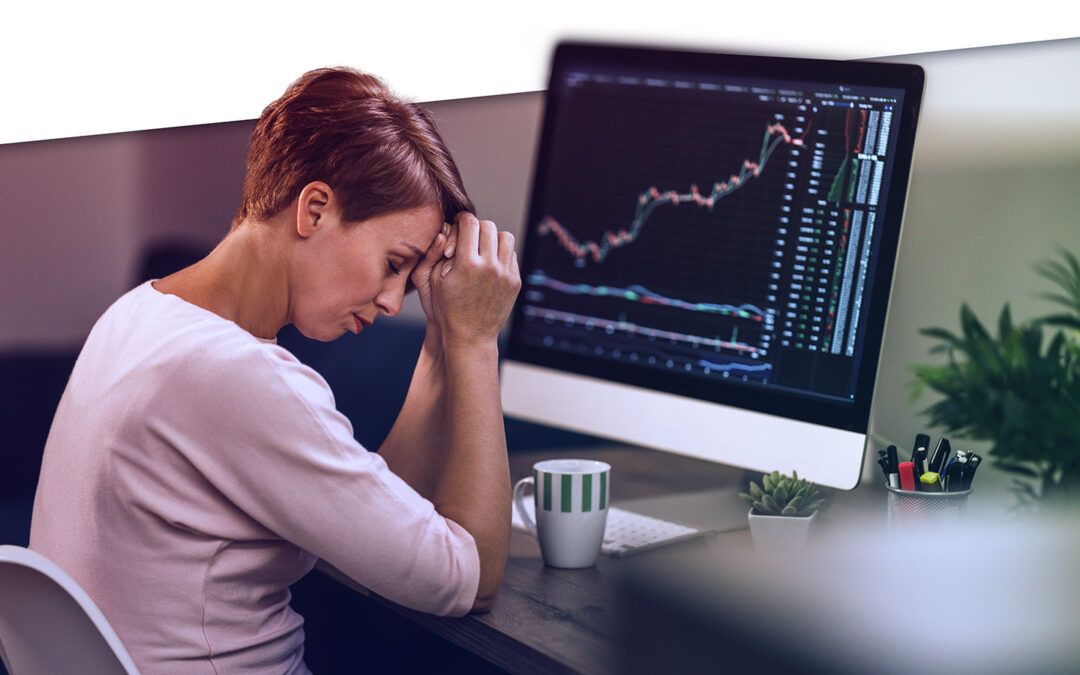
What Silicon Valley Bank Can Learn from Supply Chain Planning
If you had your head up lately, you may have noticed some additional madness off the basketball court: The failure of Silicon Valley Bank. Those of us in the supply chain world may have dismissed the bank failure as somebody else’s problem, but that sorry episode holds a big lesson for us, too: The importance of stress testing done right.