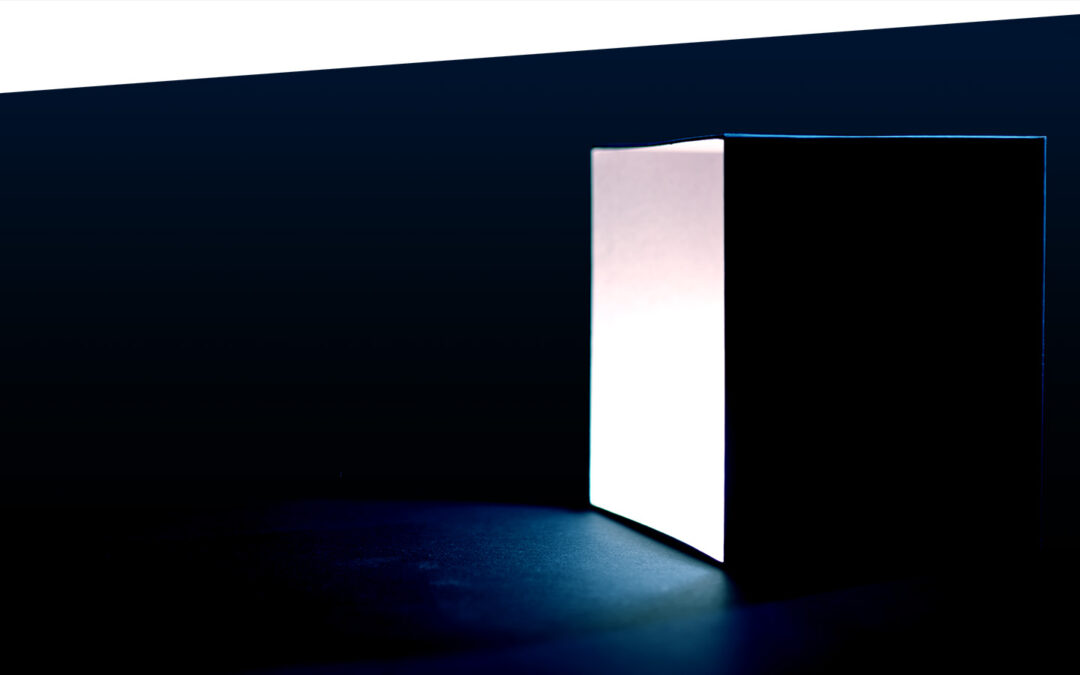
Is your demand planning and forecasting process a black box?
There’s one thing I’m reminded of almost every day at Smart Software that puzzle me: most companies do not understand how forecasts are created, and stocking policies are determined. It’s an organizational black box. Here is an example from a recent sales call:
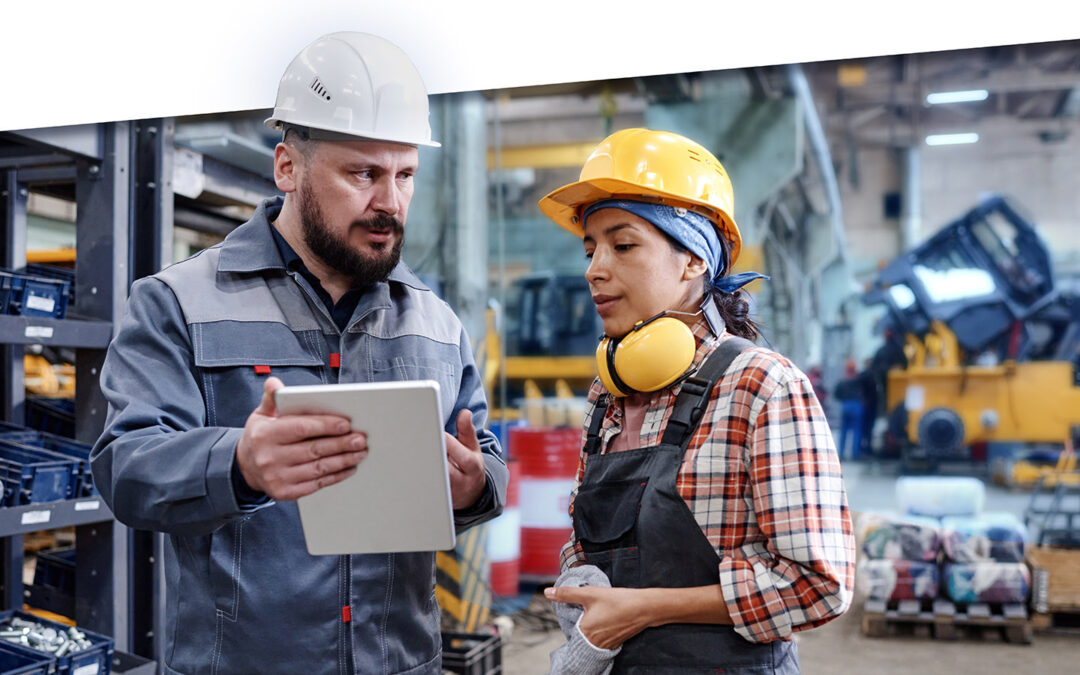
Spare Parts, Replacement Parts, Rotables, and Aftermarket Parts
Those new to the parts planning game are often confused by the many variations in the names of parts. This blog points out distinctions that do or do not have operational significance for someone managing a fleet of spare parts and how those differences impact inventory planning.
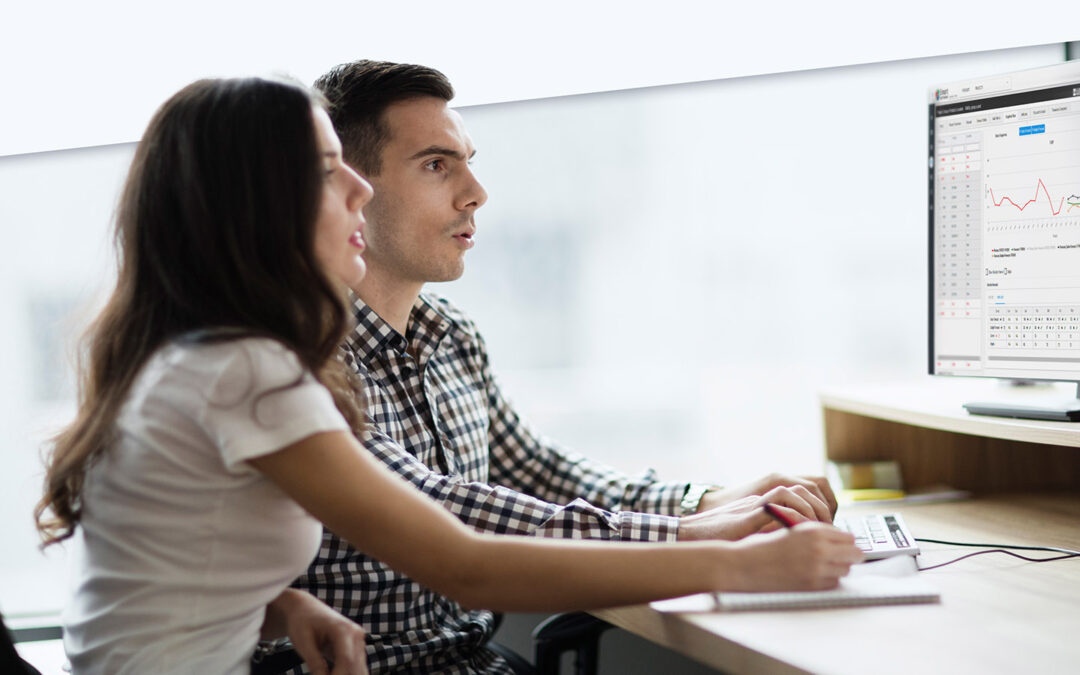
Fifteen questions that reveal how forecasts are computed in your company
In a recent LinkedIn post, I detailed four questions that, when answered, will reveal how forecasts are being used in your business. In this article, we’ve listed questions you can ask that will reveal how forecasts are created.
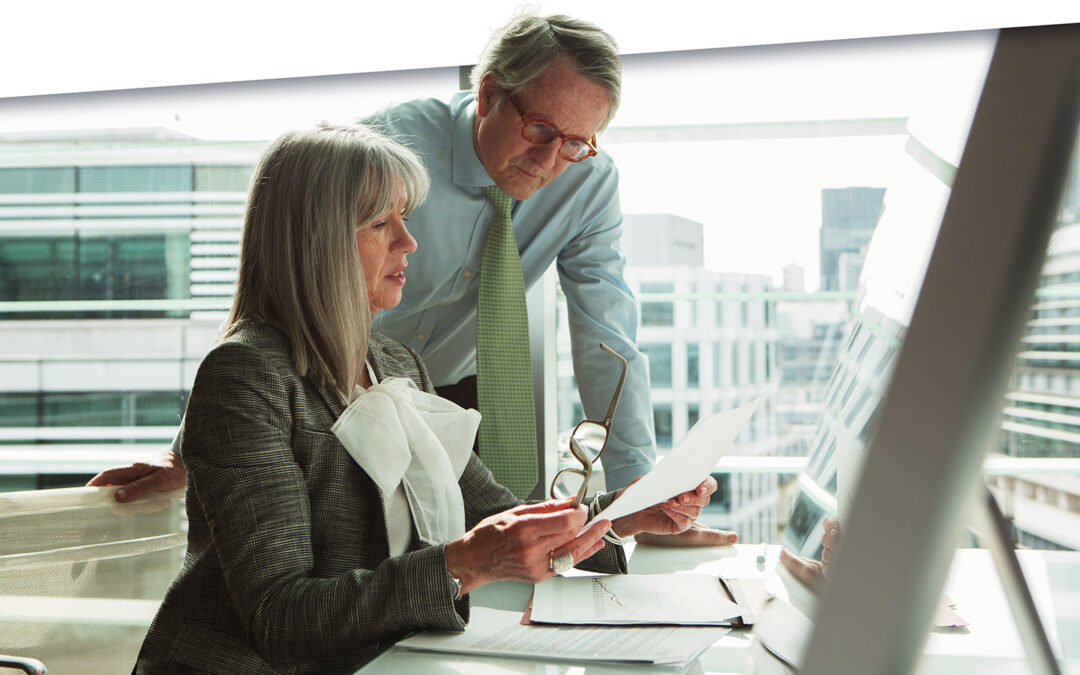
The top 3 reasons why your spreadsheet won’t work for optimizing reorder points on spare parts
We often encounter Excel-based reorder point planning methods. In this post, we’ve detailed an approach that a customer used prior to proceeding with Smart. We describe how their spreadsheet worked, the statistical approaches it relied on, the steps planners went through each planning cycle, and their stated motivations for using (and really liking) this internally developed spreadsheet.
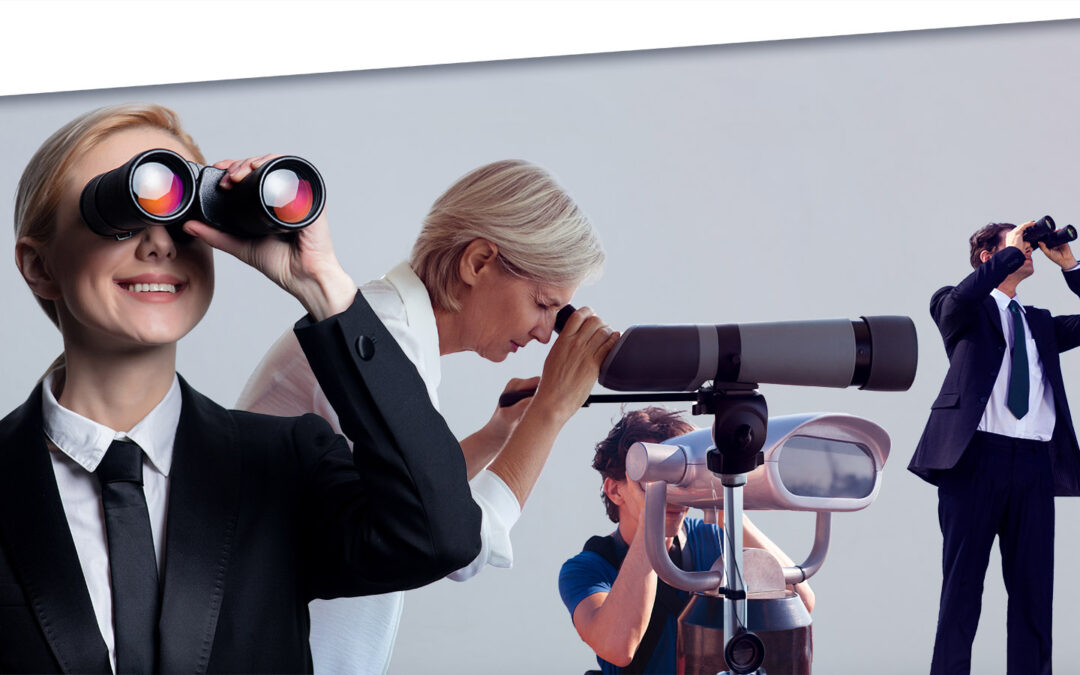
How to interpret and manipulate forecast results with different forecast methods
This blog explains how each forecasting model works using time plots of historical and forecast data. It outlines how to go about choosing which model to use. The examples below show the same history, in red, forecasted with each method, in dark green, compared to the Smart-chosen winning method, in light green.
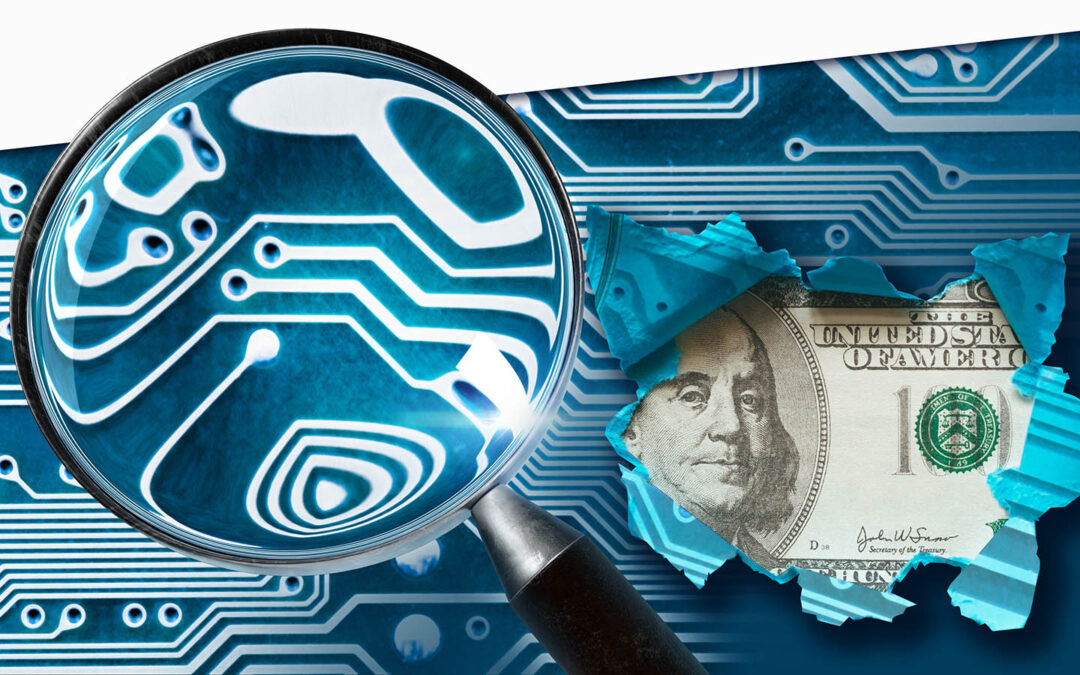
Uncover data facts and improve inventory performance
The best inventory planning processes rely on statistical analysis to uncover relevant facts about the data. When you have the facts and add your business knowledge, you can make more informed stocking decisions that will generate significant returns. You’ll also set proper expectations with internal and external stakeholders, ensuring there are fewer unwelcome surprises.
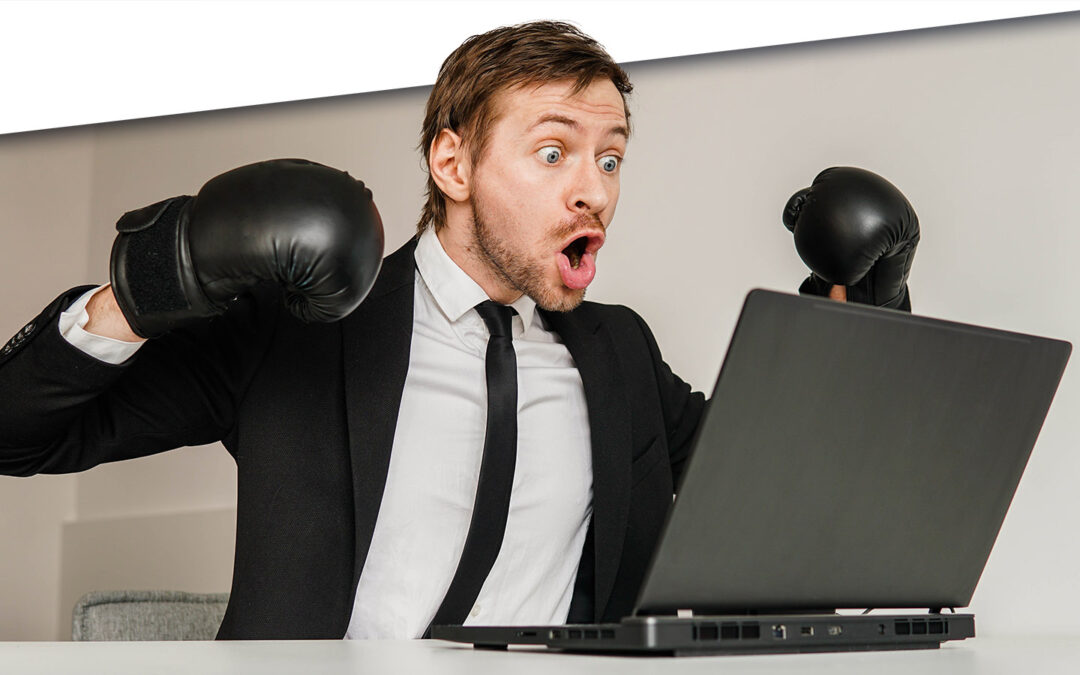
What to do when a statistical forecast doesn’t make sense
Sometimes a statistical forecast just doesn’t make sense. Every forecaster has been there. They may double-check that the data was input correctly or review the model settings but are still left scratching their head over why the forecast looks very unlike the demand history. When the occasional forecast doesn’t make sense, it can erode confidence in the entire statistical forecasting process.
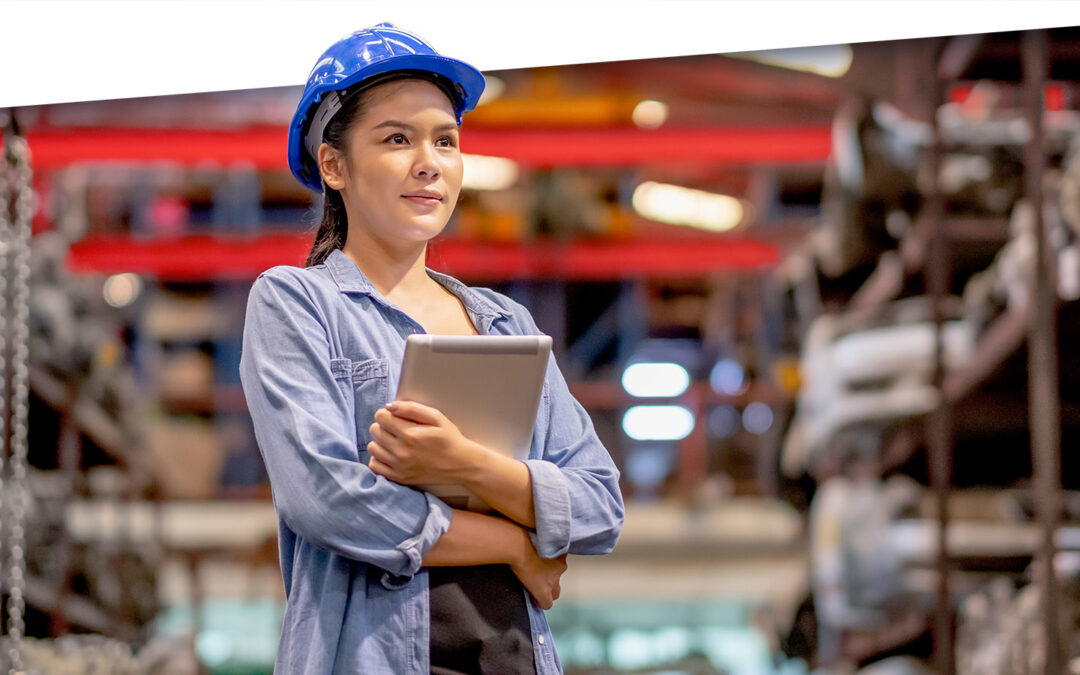
Spare Parts Planning Isn’t as Hard as You Think
When managing service parts, you don’t know what will break and when because part failures are random and sudden. As a result, demand patterns are most often extremely intermittent and lack significant trend or seasonal structure. The number of part-by-location combinations is often in the hundreds of thousands, so it’s not feasible to manually review demand for individual parts. Nevertheless, it is much more straightforward to implement a planning and forecasting system to support spare parts planning than you might think.
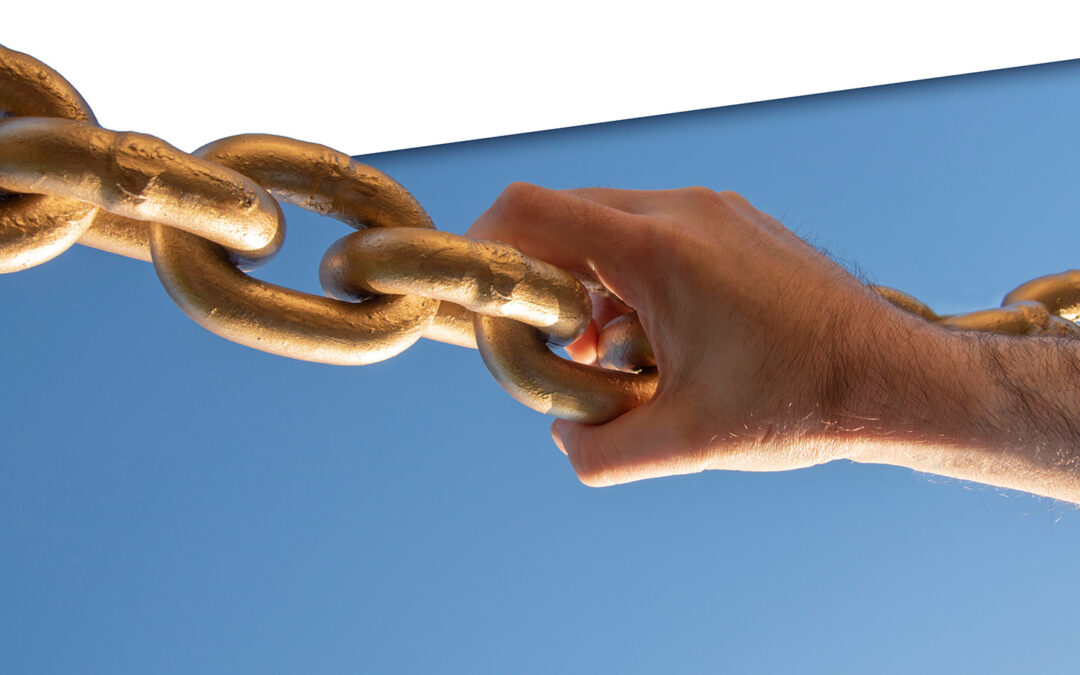
The Role of Trust in the Demand Forecasting Process Part 2: What do you Trust
Regardless of how much effort is poured into training forecasters and developing elaborate forecast support systems, decision-makers will either modify or discard the predictions if they do not trust them.”
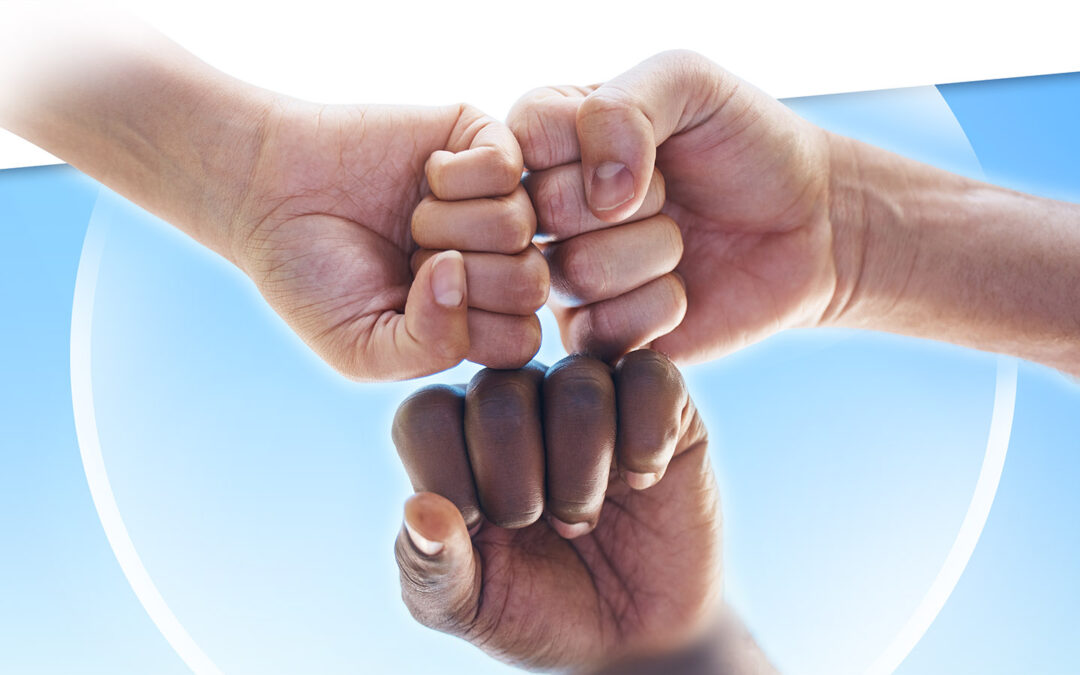
The Role of Trust in the Demand Forecasting Process Part 1: Who do you Trust
Trust is always a two-way street, but let’s stay on the demand forecaster’s side. What characteristics of and actions by forecasters and demand planners build trust in their work? Key to building trust among the users of forecasts are perceptions of forecaster and demand planner competence and objectivity.
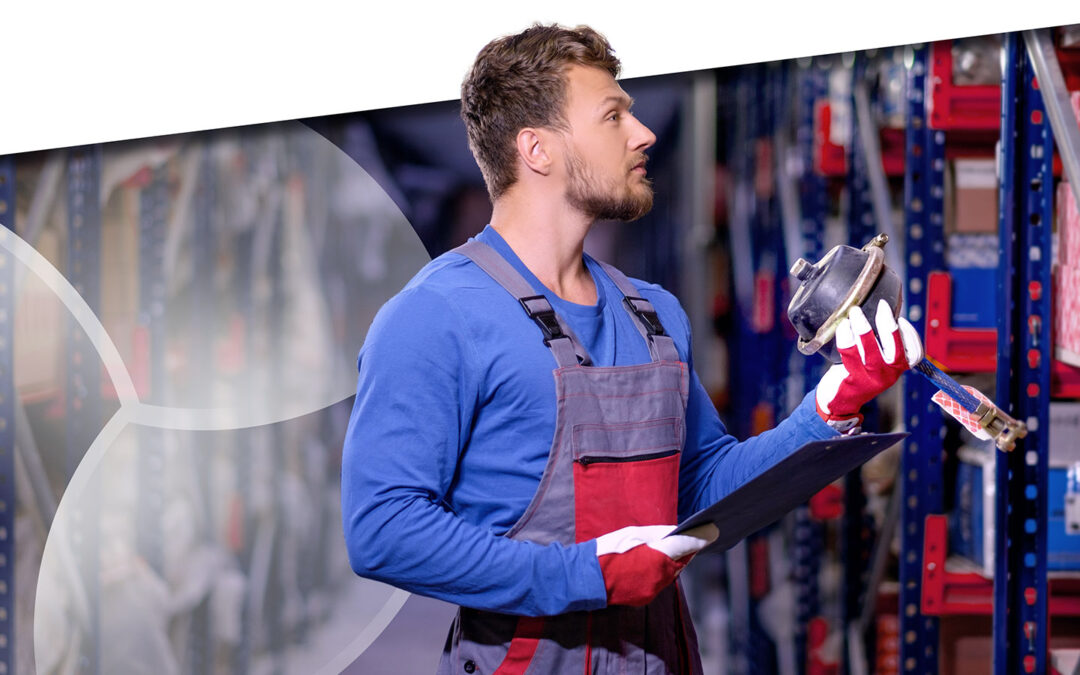
Service-Level-Driven Planning for Service Parts Businesses
Service-Level-Driven Service Parts Planning is a four-step process that extends beyond simplified forecasting and rule-of-thumb safety stocks. It provides service parts planners with data-driven, risk-adjusted decision support.
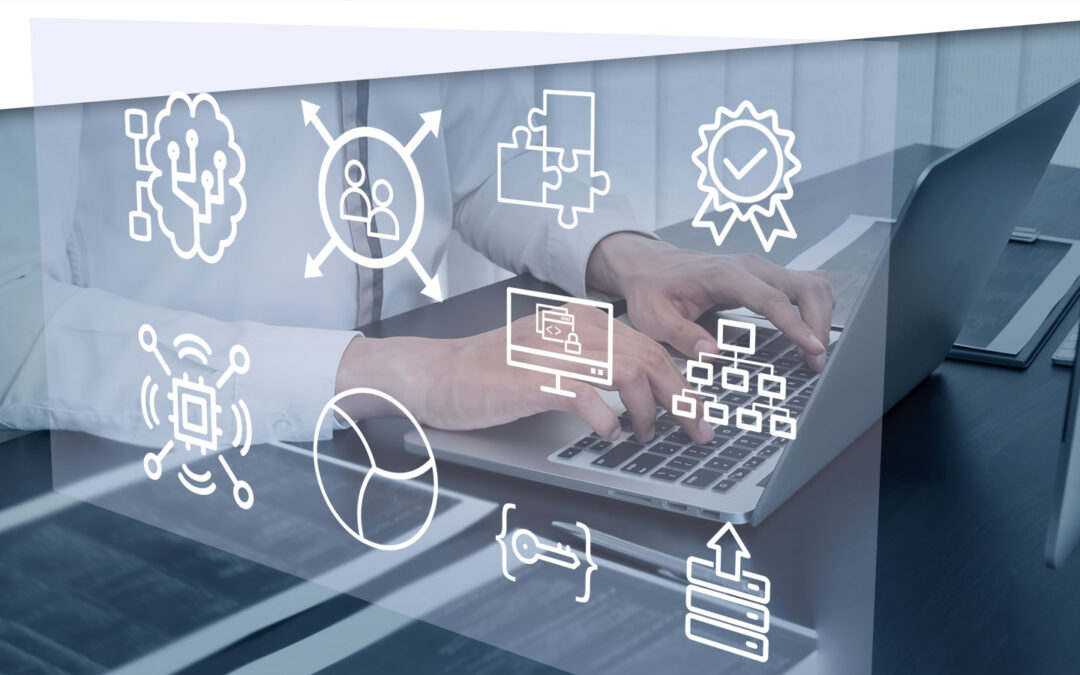
Implementing Demand Planning and Inventory Optimization Software with the Right Data
Data verification and validation are essential to the success of the implementation of software that performs statistical analysis of data, like Smart IP&O. This article describes the issue and serves as a practical guide to doing the job right, especially for the user of the new application.
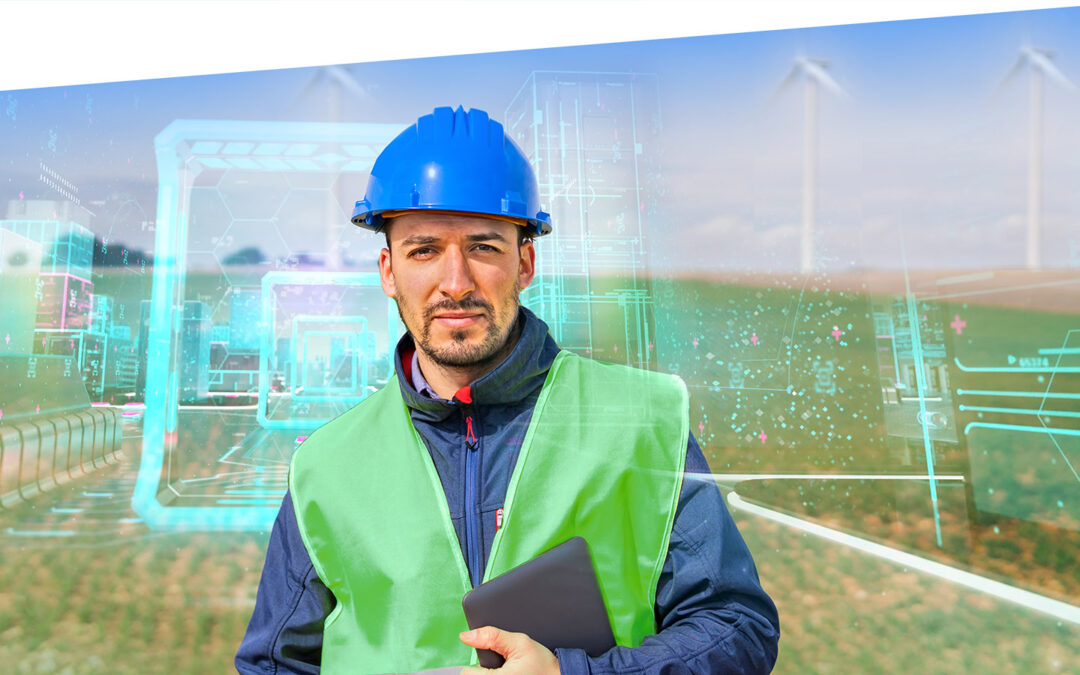
7 Digital Transformations for Utilities that will Boost MRO Performance
Utilities in the electrical, natural gas, urban water, and telecommunications fields are all asset-intensive and reliant on physical infrastructure that must be properly maintained, updated, and upgraded over time. Maximizing asset uptime and the reliability of physical infrastructure demands effective inventory management, spare parts forecasting, and supplier management. A utility that executes these processes effectively will outperform its peers, provide better returns for its investors and higher service levels for its customers, while reducing its environmental impact.
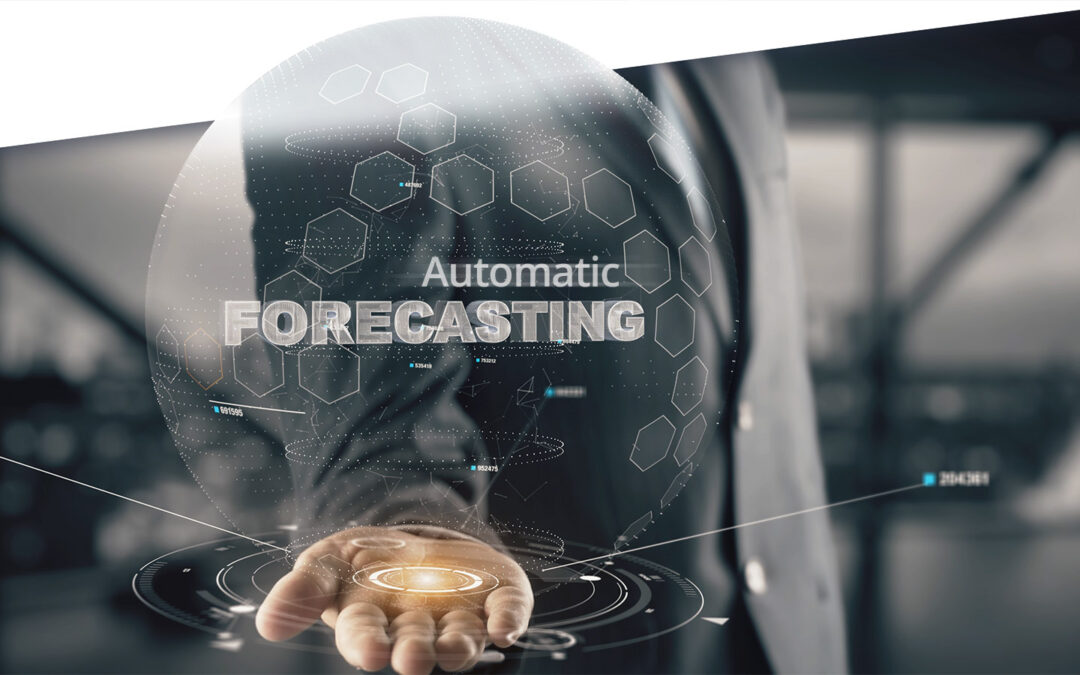
Statistical Forecasting: How Automatic method selection works in Smart IP&O
Smart IP&O offers automated statistical forecasting that selects the right forecast method that best forecasts the data. It does this for each time-series in the data set. This blog will help a laymen understand how the forecast methods are chosen automatically
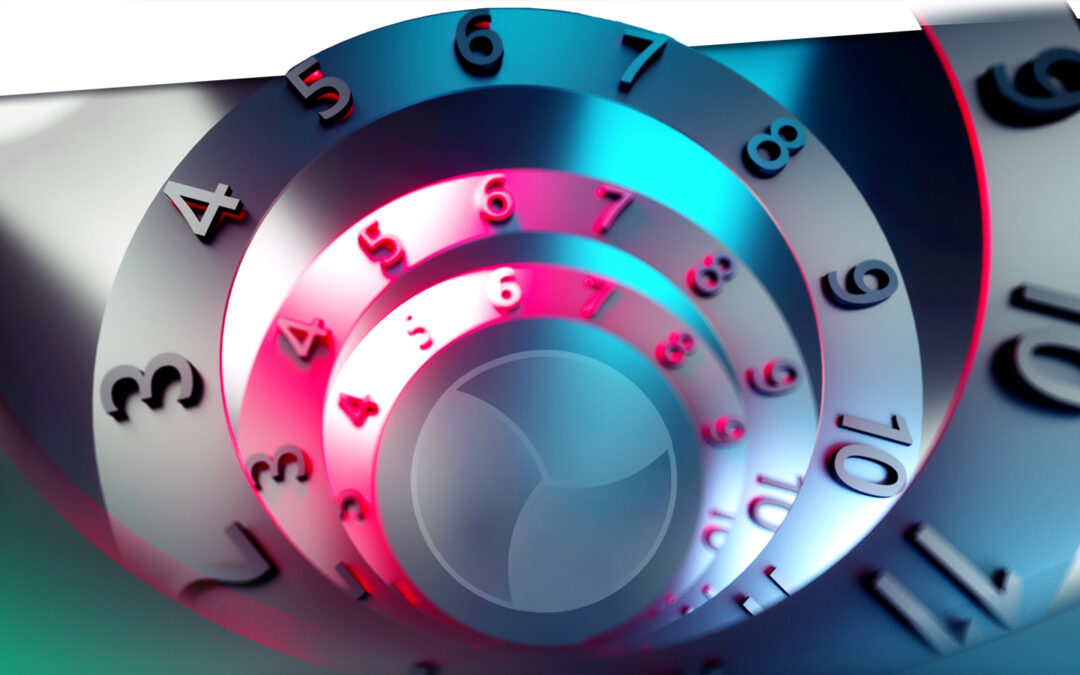
How much time should it take to compute statistical forecasts?
How long should it take for a demand forecast to be computed using statistical methods? This question is often asked by customers and prospects. The answer truly depends. Forecast results for a single item can be computed in the blink of an eye, in as little as a few hundredths of a second, but sometimes they may require as much as five seconds. To understand the differences, it’s important to understand that there is more involved than grinding through the forecast arithmetic itself. Here are six factors that influence the speed of your forecast engine.