When implementing inventory optimization, the pressure is on to get results. As the Major League Baseball season starts it’s final stretch towards the playoffs, we thought this analogy might help enforce an important point about the pace of deploying a new inventory optimization process.
The Situation
It’s the bottom of the ninth and your team is down by a run. You step up to the plate batting lead-off. With the roar of the crowd and excitement in the air, you are tempted to swing hard for that elusive home run. As you dig into the batter’s box, you weigh the options and decide on a better approach: stay patient and do whatever you can to get one base. You don’t try to hit a home run and focus on making good contact with the ball. You know that in this situation, a single will do. By getting on base you help build team confidence. Most importantly, you put the team in a better position to win the game than taking an aggressive but risky swing of the bat.
3 Reasons for a Progressive Approach to Inventory Optimization
When the pressure is on to optimize inventory, you might want to move fast much like the hitter who wants to hit that home run. And in some cases, swinging for the fences might be the recommended approach. More often than not, a progressive approach to inventory optimization is more effective. Here are three reasons why:
- It builds user confidence and creates momentum
- Early success buys necessary time to get full management buy-in
- Corporate roll out of a new process requires progressive evidence of success
Industry Example
A case in point is a multinational company offering next day PC and electronic device repair services. They never know what will come in for repair, but need to set inventory policy to meet their next day service commitment for thousands of parts, while keeping inventory to a minimum. They’ve chosen our inventory optimization software to help and are in the process of implementing. The planning team has taken this progressive approach, working brand by brand to set optimal reorder points and order quantities using Smart’s probability forecasting engine. They selected one brand to illustrate the process and show results: starting with 26 items, they filtered out 14 parts with little or no demand history. Assessing the remaining 12, they showed how to reduce inventory by $51,000 while increasing service levels. While the $51,000 reduction was just a small proportion of the overall benefit, it was easily understood, and presenting it has helped gain support to build out their inventory optimization implementation, brand by brand across the company.
Confidence is Key
User confidence is key and this comes with mastering the use of the inventory optimization software and being able to present results. So, too, is management confidence. We have encountered situations where optimization scenarios have clearly shown opportunities for large inventory savings, but staff were reluctant to seize them. Why? Because they would be reducing inventory, and that felt risky. Again, selecting a subset of items, working through the optimization process, and gaining the top-down management support to make the change made all the difference. And they will substantially reduce their inventory as a result.
Inventory Optimization success comes with a motivated team, the right technology, and solid execution of a good plan. A progressive approach to implementation reduces risk, validates the plan, and provides the foundation for an effective inventory planning and optimization program.
Download Smart Inventory Optimization product sheet here: https://smartcorp.com/inventory-optimization/
Related Posts
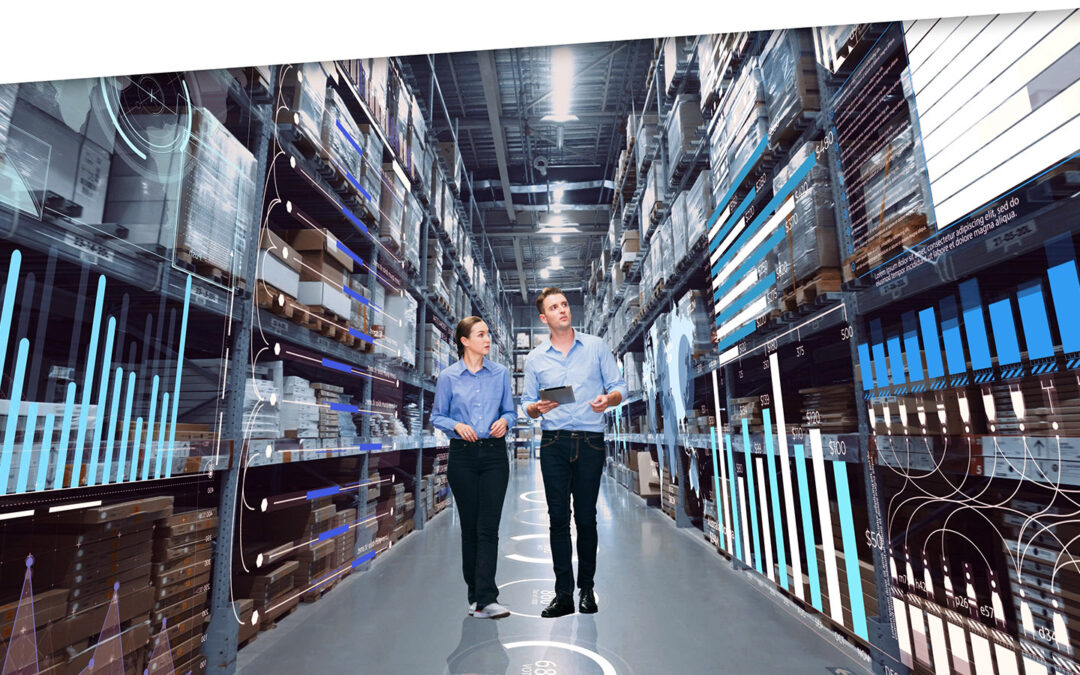
Make AI-Driven Inventory Optimization an Ally for Your Organization
In this blog, we will explore how organizations can achieve exceptional efficiency and accuracy with AI-driven inventory optimization. Traditional inventory management methods often fall short due to their reactive nature and reliance on manual processes. Maintaining optimal inventory levels is fundamental for meeting customer demand while minimizing costs. The introduction of AI-driven inventory optimization can significantly reduce the burden of manual processes, providing relief to supply chain managers from tedious tasks.
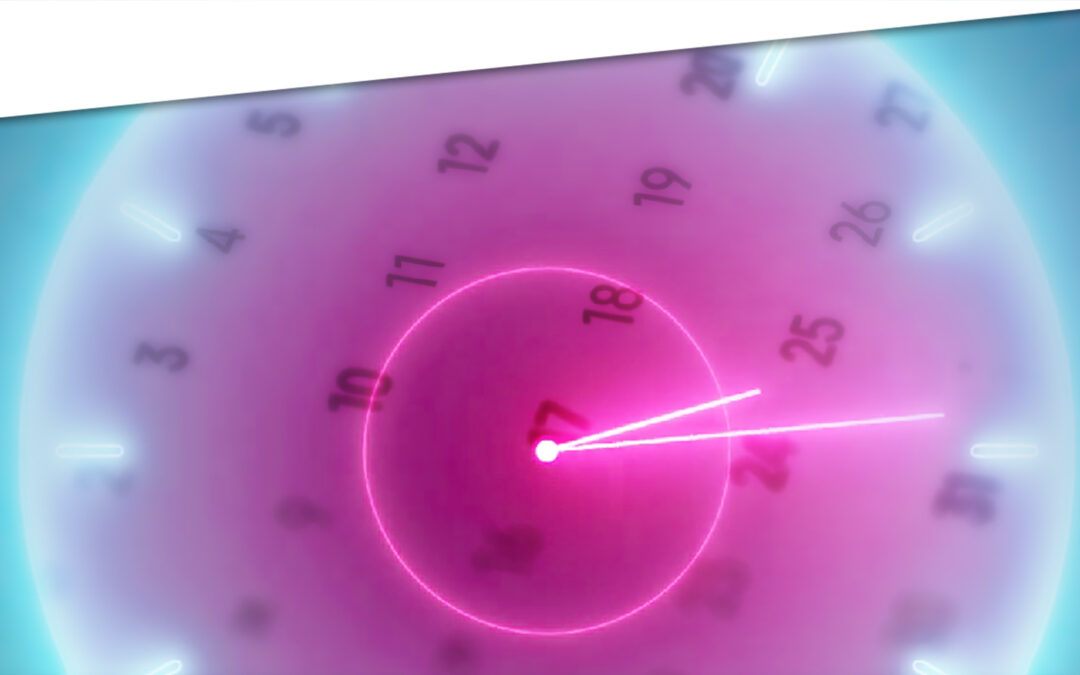
Daily Demand Scenarios
In this Videoblog, we will explain how time series forecasting has emerged as a pivotal tool, particularly at the daily level, which Smart Software has been pioneering since its inception over forty years ago. The evolution of business practices from annual to more refined temporal increments like monthly and now daily data analysis illustrates a significant shift in operational strategies.
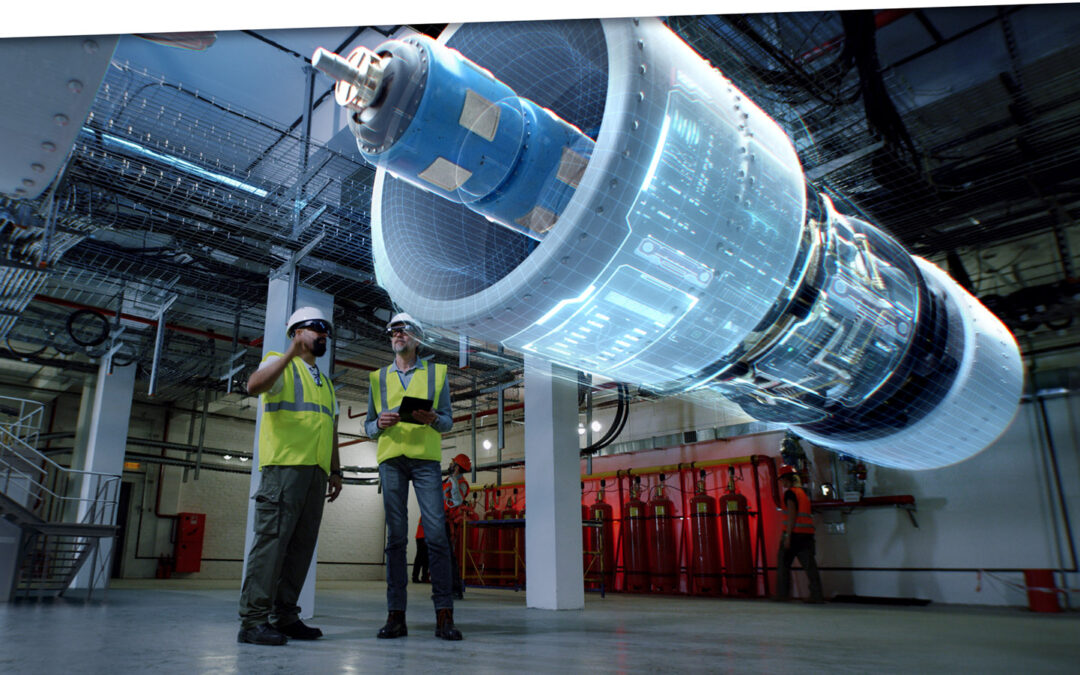
Constructive Play with Digital Twins
Those of you who track hot topics will be familiar with the term “digital twin.” Those who have been too busy with work may want to read on and catch up. While there are several definitions of digital twin, here’s one that works well: A digital twin is a dynamic virtual copy of a physical asset, process, system, or environment that looks like and behaves identically to its real-world counterpart. A digital twin ingests data and replicates processes so you can predict possible performance outcomes and issues that the real-world product might undergo.