Quants and Financial Meltdowns
I spend much of my time developing new quantitative methods for statistical forecasting, demand forecasting and inventory optimization. For me, this is an engaging way to contribute to society. But I know that the most prudent way to do algorithm development is to stand a little to the side and cast a skeptical eye on my own work.
The need for this skepticism was highlighted for me recently as I read Scott Patterson’s book The Quants: How a New Breed of Math Whizzes Conquered Wall Street and Nearly Destroyed It (Crown Publishing, 2010). This book reviewed the “quants” whose complex financial models were largely responsible for the financial meltdown in 2007. As I read along and thought “What was wrong with these guys?” I began to wonder if we supply chain quants were guilty of some of the same sins.
Models versus Instincts
Generally, the supply chain field has lagged behind finance in terms of the use of statistical models. My university colleagues and I are chipping away at that, but we have a long way to go. Some supply chains are quite technically sophisticated, but many, perhaps more, are essentially managed as much by gut instinct as by the numbers. Is this avoidance of analytics safer than relying on models?
What makes gut instinct dangerous is that it is so amorphous. Everyone who works long in a job develops instincts, but longevity is not the same as wisdom. It is possible to learn all the wrong lessons over a long career. It is also possible to miss the chance to learn the right lessons because certain informative scenarios may never arise in one person’s career. It is also possible to have good days and bad days; even gurus can mess up. Gut instinct is also anti-productive, since all decisions have to pass through that one gut, which becomes an enterprise chokepoint. And Golden Guts eventually reach their Golden Years and take their Golden Watch and go off into a Golden Sunset; at that point, whatever expertise had been present has walked out the door.
In contrast, models have certain advantages. Relative to gut instinct, models are:
- Explicit: The theory of the supply chain operation is exposed for all to see.
- Adaptive: Because the theory is visible, it can be reviewed, critiqued, tested against data, and evolved.
- Consistent: Models may be more or less true, but they are not subject to day-to-day variability.
- Comprehensive: At least potentially, models can accumulate a wide range of empirical experience, including scenarios never encountered during any one person’s career.
- Instructive: Models are collections of relationships among variables. If the model’s “guts” are made visible, users can learn about those relationships.
Model Error
Nevertheless, despite all their virtues, models can also be wrong. In fact, that is a given. A constructive way to live with this is encoded in the famous aphorism by Dr. George Box, one of the best modelers of the last half century: “All models are wrong. Some are useful.”
The finance quants’ models were wrong by being oversimplified. They started with a quasi-religious belief in the efficiency of markets and developed statistical models that made certain assumptions that were more likely to be true of the physical world than the financial world. Among these were Normal distributions of changes in asset prices and independence of events across various corners of the market. They also assumed human rationality.
It should be a bit alarming that the Normal distribution and independence assumptions also underlie many of the models in supply chain software. In fact, there are alternative models of supply chain dynamics that do not require these simplifying assumptions, so this is an unnecessary risk being run by many, perhaps most, of the users of supply chain software.
But even with more robust and realistic model assumptions, there is no denying that model error is a constant risk. So, can you be victimized by your models? Of course you can.
Self-Protection: Look at the Data
Every supply chain professional who uses models, then, is subject to model risk. But unlike with decisions based on gut feel, decisions based on model calculations can be exposed and compared to real-world outcomes. Repeated checking is the best way to protect against model error, because it not only tests whether the model is realistic but also signals when it is time to update the model.
As noted above, a model is a set of functional relationships between key variables. Those relationships have parameters that tune the model to the current operating context. For instance, supply chain models often rely, in part, on estimates of demand volatility. Historical demand data are used to calculate numerical values for these parameters. If demand volatility changes, the model becomes obsolete and likely to produce inapt recommendations. Therefore, good practice demands frequent updates to model parameters.
Even when parameter values are current, there may still be trouble due to incorrect functional relationships. For example, consider the relationship between the mean and standard deviation of demand for spare parts. Generally speaking, the greater the average demand, the greater the demand volatility as measured by the standard deviation.
Now consider simplified “old school” models that describe spare part demand as a Poisson process. The Poisson process is widely useful and relatively simple, so it often shows up in Statistics 101 classes. Because of their relative simplicity, Poisson models are the white rats of supply chain analytics for spare parts, i.e., people do computer experiments and theory development based on the behavior of Poisson models of demand. For Poisson models, the standard deviation of demand equals the square root of the mean. However, when we look at our customers’ actual demand data, we discover that the actual relationship between the mean and standard deviation of demand is better described by a more general power-law relationship. Thus, the simple model may use accurate estimates of mean and standard deviation but still not accurately reflect their relationship. This in turn leads to incorrect recommendations about reorder points for spare parts. Checking real data is the best antidote to cavalier assumption-making.
What to Do Next
I do not sense that today’s supply chain models are on the brink of creating the kind of meltdown we saw in the start of the Great Recession. But those of us who are supply chain quants need to show more professional maturity than our financial colleagues. We need to not fall in love with our models, and we need to alert our customers to correct model hygiene.
So, model users, wash your hands frequently as we begin flu season, and wash your models thoroughly through hard data to be sure that the models you rely on are both up-to-date and grounded in reality. Both those steps will protect you from being victimized by your models and let you exploit their advantages over management by gut feel.
Appendix: Technical Tips
Supply chain analytics provide various types of outputs. In the realm of forecasting and demand planning, the obvious empirical check is to compare forecasts against the actual demand values that eventually reveal themselves. This same “forecast then check” approach can also be used in the generation of forecasts. In the realm of inventory management, the models can build on forecasts to recommend policy choices, such as reorder points and order quantities or Min and Max values. There is a smart way to confirm the accuracy of recommendations of reorder points and Min’s. See our blog The Right Forecast Accuracy Metric for Inventory Planning
Related Posts
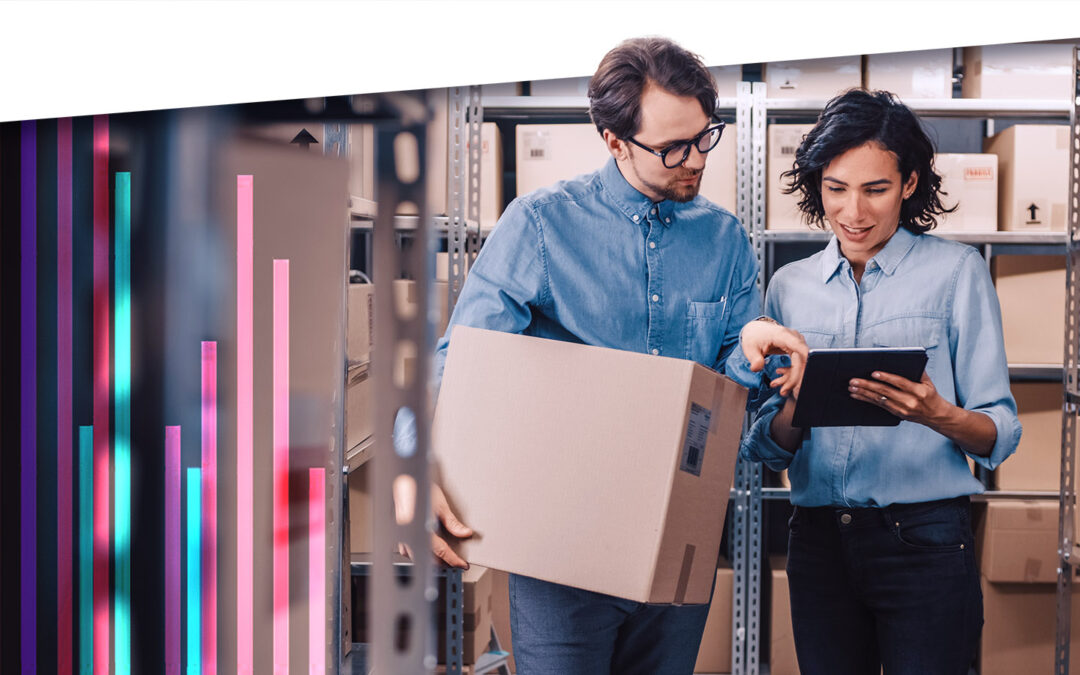
Forecast-Based Inventory Management for Better Planning
Forecast-based inventory management, or MRP (Material Requirements Planning) logic, is a forward-planning method that helps businesses meet demand without overstocking or understocking. By anticipating demand and adjusting inventory levels, it maintains a balance between meeting customer needs and minimizing excess inventory costs. This approach optimizes operations, reduces waste, and enhances customer satisfaction.
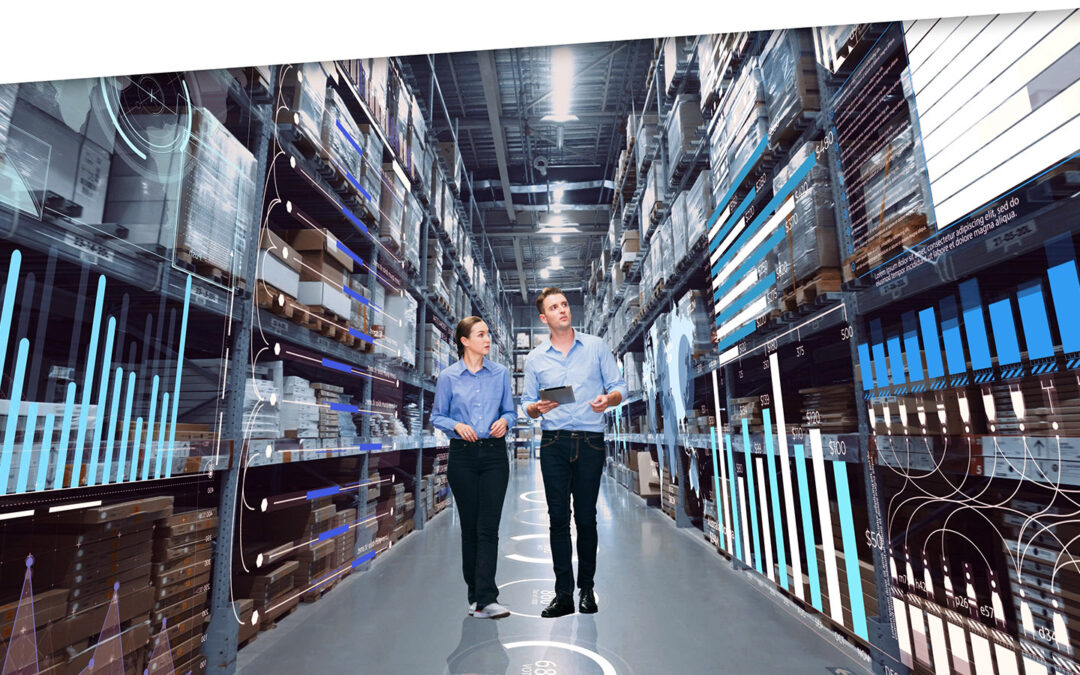
Make AI-Driven Inventory Optimization an Ally for Your Organization
In this blog, we will explore how organizations can achieve exceptional efficiency and accuracy with AI-driven inventory optimization. Traditional inventory management methods often fall short due to their reactive nature and reliance on manual processes. Maintaining optimal inventory levels is fundamental for meeting customer demand while minimizing costs. The introduction of AI-driven inventory optimization can significantly reduce the burden of manual processes, providing relief to supply chain managers from tedious tasks.
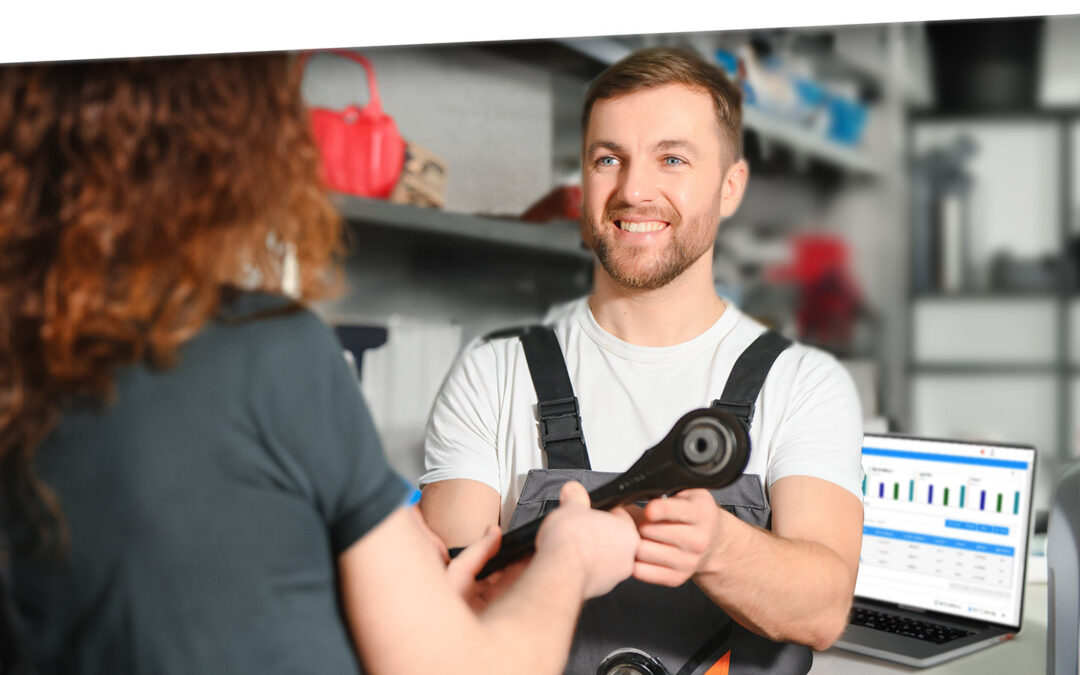
The Importance of Clear Service Level Definitions in Inventory Management
Inventory optimization software that supports what-if analysis will expose the tradeoff of stockouts vs. excess costs of varying service level targets. But first it is important to identify how “service levels” is interpreted, measured, and reported. This will avoid miscommunication and the false sense of security that can develop when less stringent definitions are used. Clearly defining how service level is calculated puts all stakeholders on the same page. This facilitates better decision-making.