“Trillions of records of millions of people…Finding the useful and right information, understanding its quality and producing reliable analyzed data in a timely and cost-effective manner are all critical issues.”
Smart Software Senior Vice President for Research Tom Willemain recently had the opportunity to talk with Dr. Mohsen Hamoudia, President of the International Institute of Forecasters (IIF), to discuss current issues with, and opportunities for, big data analytics. The IIF informs practitioners on trends and research developments in forecasting via print and online publications and the hosting of professional conferences.
Dr. Hamoudia begins, by way of introduction:
In all industries, data availability is exploding in volume, variety and velocity. Big data analytics is playing an important role in identifying the data that is most important to the business.
Let me take the example of the Information and Communication Technology (ICT) sector. We are seeing literally exponential growth in the amount of data available to telecoms, Over-the-top (OTT) independent content distributors, government, regulators and other organizations.
Around the world, we are witnessing petabytes of data: trillions of records of millions of people—all coming from multiple sources. Among these sources: internet connections, sales, customer contact centers, social media, mobile and land lines data. Finding the useful and right information, understanding its quality and producing reliable analyzed data in a timely and cost-effective manner are all critical issues. ICT companies are increasingly looking to find actionable insights in their data. How they can increase their customer base and loyalty programs? How can they improve the quality of service (QoS) and reduce customer turnover? With the right big data analytics platforms in place, they can be more competitive and efficient, improving operations, customer service and risk management. Forecasting and predicting customer trends and directions are key for telecoms.
Forecasting skills, including mathematics, statistics and econometrics, form one of the most important “blocks” of required skills in managing Big Data. Some forecasting activities naturally form part of the big data debate.
In retail industries, forecasting addresses daily demand across thousands of products. Financial forecasting, whether considering customer behavior or financial data series, generates massive on-line data sets. As pointed out by Robert Fildes, Distinguished Professor at Lancaster University, as yet the academic forecasting community is not thoroughly engaged—with only a few exceptions. Hal Varian of Google has looked at some of the work that David Hendry and Jennifer Castle, at Oxford University, have undertaken on searching large data sets for data congruent meaningful models. Stock and Watson have also developed their own approaches to large macro data sets. But despite the attempt, at last year’s symposium on forecasting in Seoul, to explore the theme of big data and its forecasting applications, there remain few convincing applications of using on-line data on real forecasting problems.
Q. One hears a great deal about “predictive analytics” these days, yet the phrase rarely is linked with forecasting. Do you agree that forecasting lies at the heart of predictive analytics? Have you an explanation for why the link has been broken? Have you ideas about how to re-inject forecasting into the conversation?
The results of forecasting (the “what”) are perhaps now perceived as less important than the “how”. Consequently, the trust that users give to traditional forecasting has declined. Who indeed is challenging the accuracy or relevance of forecasting by comparing, a posteriori, the reality vs. forecast—making a case for metholodiges’ effectiveness and therefor building credibility?
With the current perception of “predictive analytics”, there is probably more space in the public imagination allocated to the “how” side of things, and therefor a more credible story to tell to partners, investors or customers.
Q. It appears that there is almost no link between traditional forecasting and mobile technology (smart phones, tablet computers). Is this true, or are some companies migrating forecasting to mobile devices? Do you see a path forward in which traditional forecasting algorithms would routinely reside on mobile devices?
First of all, I am really delighted to invite your readers to have a look at our latest issue of Foresight. An excellent paper on the subject, “Forecasting In the Pocket: Mobile Devices Can Improve Collaboration”, explains that “the increasing popularity of PDAs, smartphones, tablet computers and other mobile devices opens up new opportunities for communication and collaboration on business forecasts.” The authors tell us “mobile forecasting (m-forecasting) applications may streamline approaches to collaboration between retailers and suppliers, thus contributing to the provision and exchange of product information, especially since forecasts are strongly tied to local context knowledge.”
For example, on the ICT & OTT side, a large number of predictive projects, such as those of Google+ and Facebook, are happening thanks to the inclusion of the “user location” data in the OTT IT systems. In my opinion, and what I see in some sectors like retail and logistics, is that traditional forecasting and mobile forecasting (m-forecasting) are complementary. This latter could be seen as a bottom-up forecasting approach that will or will not confirm the top-down forecasting results.
Q. Some people argue that big data will facilitate the replacement of forecasting with “sense and react” systems. Practically speaking, how would you explain “sense and react”, and are there application areas where you think it is or is not likely to take hold?
It seems to me that “sense and react” is fully oriented to the short-term perspective. Forecasting extends this by addressing needs for a variable horizon: short-term and medium-term.
As a side effect of ATAWAD (Anytime, Anywhere, Any Device), the decision-making criteria are, more than ever, “short term”. Big data is a “weak signals” sensing system, which enable the near-real-time detection of business opportunities that would go unnoticed with traditional IT systems. There are not really preferred or non-priority applications for this, the question is more on the “when” side.
Big data is relevant when looking below the surface in difficult economic times, but I am less sure whether it is worth the effort in “normal” economic period. To conclude on this point: I will be happy to see an example on how accurate are forecasts which are based on “sense and react” versus forecast based on traditional models.
Q. I’m asking some big questions. To what extent do you see the IIF community shaping these discussions and outcomes? How can readers join in the dialogue?
We are expecting an increasing availability, and increasing usage, of huge amount of data in many industries—such as energy, transportation, health care, finance, telecommunications and tourism.
Many of the IIF’s members are engaged in different aspects of the big data “movement.” The IIF is doing some work in the forecasting activities that naturally form part of the big data debate. More generally, the IIF is actively participating in, and providing a forum for, the discussion of forecasting in the wider world.
The theme of our last International Symposium on Forecasting (ISF) held in Seoul was “Forecasting with Big Data” and a few presentations were related to health care and telecommunications. A relevant workshop has just been run by the European Central Bank (ECB). If these models are capitalized on, they have the potential to impact the economic policy of Europe quite quickly.
Readers can join in the dialogue by contributing papers to the IIF’s publications (The International Journal of Forecasting, Foresight and The Oracle). Foresight, for one, is an invaluable voice in bringing academics and practitioners together in an ongoing discussion.
Readers also can present papers at the annual conference (the aforementioned ISF). They also can suggest and organize specific workshops for specific applications of big data, like the one that was just organized by the ECB in Frankfurt. Another opportunity is to invite IIF’s members to attend any meeting related to forecasting with big data. All these opportunities form good platforms for networking and working together.
Mohsen Hamoudia, PhD, is the President of the International Institute of Forecasters. He also serves as Head of Strategy for Large Projects (Paris) for Orange Business Services (the former France Telecom).
Thomas Willemain, PhD, co-founded Smart Software and currently serves as Senior Vice President for Research. Dr. Willemain also serves as Professor Emeritus of Industrial and Systems Engineering at Rensselear Polytechnic Institute, and as a member of the research staff at the Center for Computing Sciences, Institute for Defense Analyses.
Related Posts
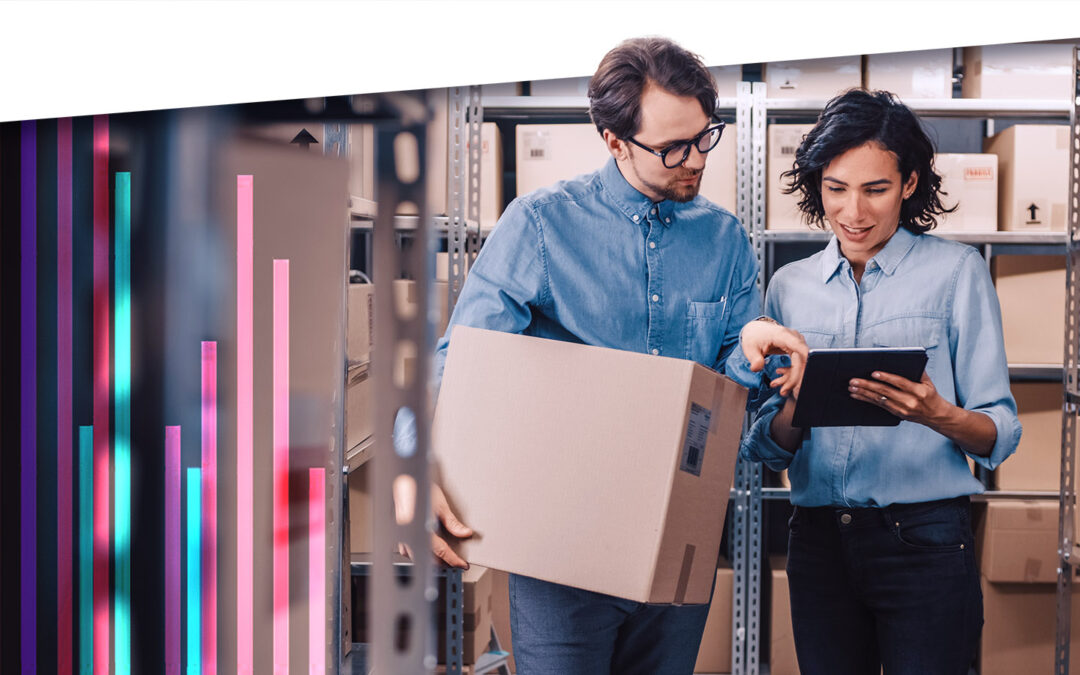
Forecast-Based Inventory Management for Better Planning
Forecast-based inventory management, or MRP (Material Requirements Planning) logic, is a forward-planning method that helps businesses meet demand without overstocking or understocking. By anticipating demand and adjusting inventory levels, it maintains a balance between meeting customer needs and minimizing excess inventory costs. This approach optimizes operations, reduces waste, and enhances customer satisfaction.

Future-Proofing Utilities: Advanced Analytics for Supply Chain Optimization
Utilities in the electrical, natural gas, urban water, and telecommunications fields are all asset-intensive and reliant on physical infrastructure that must be properly maintained, updated, and upgraded over time. Maximizing asset uptime and the reliability of physical infrastructure demands effective inventory management, spare parts forecasting, and supplier management. A utility that executes these processes effectively will outperform its peers, provide better returns for its investors and higher service levels for its customers, while reducing its environmental impact.
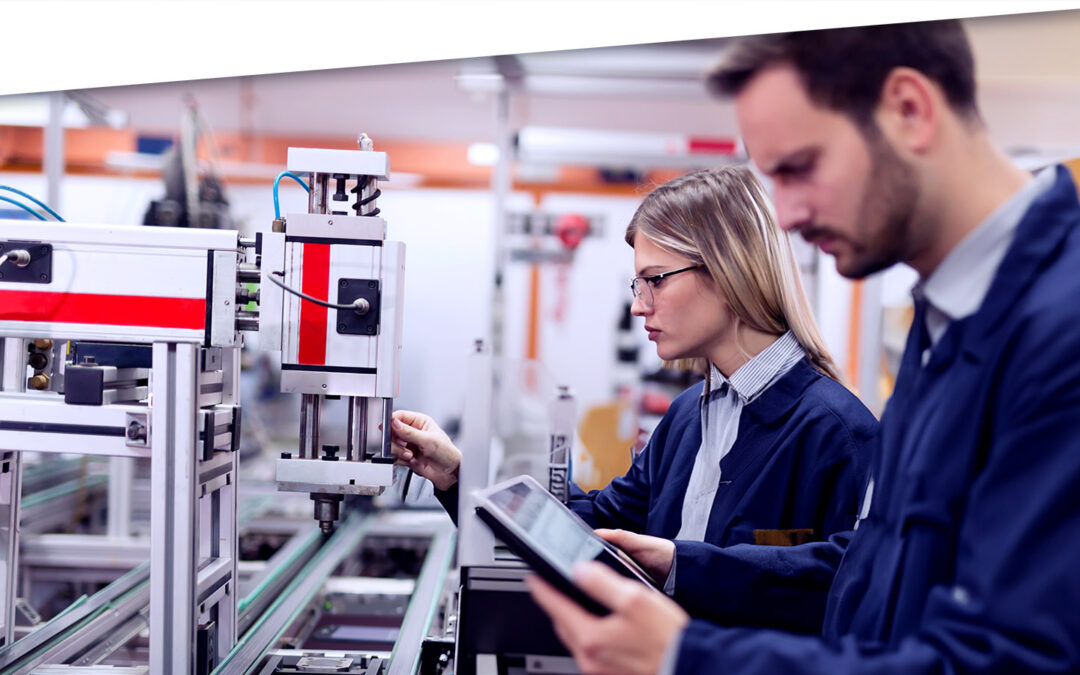
Leveraging Epicor Kinetic Planning BOMs with Smart IP&O to Forecast Accurately
In this blog, we explore how leveraging Epicor Kinetic Planning BOMs with Smart IP&O can transform your approach to forecasting in a highly configurable manufacturing environment. Discover how Smart, a cutting-edge AI-driven demand planning and inventory optimization solution, can simplify the complexities of predicting finished goods demand, especially when dealing with interchangeable components. Learn how Planning BOMs and advanced forecasting techniques enable businesses to anticipate customer needs more accurately, ensuring operational efficiency and staying ahead in a competitive market.