No, not that kind of regime change: Nothing here about cruise missiles and stealth bombers. And no, we’re not talking about the other kind of regime change that hits closer to home: Shuffling the C-Suite at your company.
“Regime change” has a third meaning that is relevant to your profession as a demand planner or inventory manager. To researchers in economics and finance, regime change means sudden shifts in the very character of a time series of random observations. The random time series in question here is the sequence of daily (or weekly or monthly) demand counts for your products and inventory items.
Most forecasting software uses statistical algorithms to process historical demand. It may add additional steps, such as incorporating field intelligence from sales people, but everything starts with the demand history of whatever item you must manage.
The question raised by regime change is, which data do you use? The simple answer is “All of it”, because that leads to the most accurate forecasts — but only if your data world is stable. If your data world is turbulent, then using all the data means you are basing forecasts on bye-gone conditions. In turn, inputting obsolete data into your forecasting algorithms inevitably leads to reduced forecast accuracy.
Note that dealing with regime change is not the same as dealing with outliers. Outliers are usually one-off exceptions caused by transient events, such as a kink in your supply chain caused by a huge blizzard choking off all transit paths. In contrast, regime change persists over a longer period and is therefore capable of doing more damage to your forecasts. Here’s an analogy: Outliers are about weather, and regime change is about climate.
The most drastic forms of regime change are existential. Figure 1 shows an example of an existential change: There was no demand at all for a long time, then suddenly there was demand. If you had no demand for an item because it didn’t exist but you retain zero demand values in your database, and then the item goes live and you do have sales, the transition from nothing to something is an extreme regime change. Including all those zero demand values from before “Day One” is sure to bias statistical forecasts down below where they should be. The same thing happens if you kill off a product but keep recording zero demand: Including all those recent zeros degrades your demand forecasts.
In principle, careful record keeping should eliminate these problems. You should record only meaningful zero values. If you have a new item, start recording when it goes live. If you no longer have any demand for an item and expect none, purge it from your database, or at least forecast zero demand.
Unfortunately, there is a difference between principle and practice. We see many instances in which the data records for both new and dormant items are not properly kept, with “fake zeros” confounded with “real zeros”. This problem is not necessarily the result of incompetence: Usually, it is a byproduct of the scale of the problem, with too few people trying to keep track of too many items.
These existential regime changes are relatively easy to deal with compared to more subtle forms, which appear to afflict more items. Figure 2 shows two examples of regime changes in a pattern of ongoing sales. There are any number of factors that can change the demand for an item: salesforce performance, marketing and advertising efforts, competitor and supplier actions, new customers arising or old customers disappearing, etc. If demand for an item has been chugging along at a steady 1 unit per day but suddenly doubles (or vice versa), that’s a regime change. In the new world order, demand is 2 units/day and forecasts should reflect that. Instead, statistical forecasting algorithms will forecast too little demand if fed all the data, including that from before the regime change.
How do you protect yourself from regime change? The answer is the same for the cruelest dictator or the most innocent demand planner: Intelligence. And because threats are many, the intelligence is best automated. Modern software systems have the capability to screen tens of thousands of items for signs of regime change. Then the software can call your attention to the problematic items and prompt you to designate which recent data to use in calculations. Or the software can automatically detect and correct for regime change, working quickly at a scale that would easily defeat any busy person working “by hand”.
Related Posts
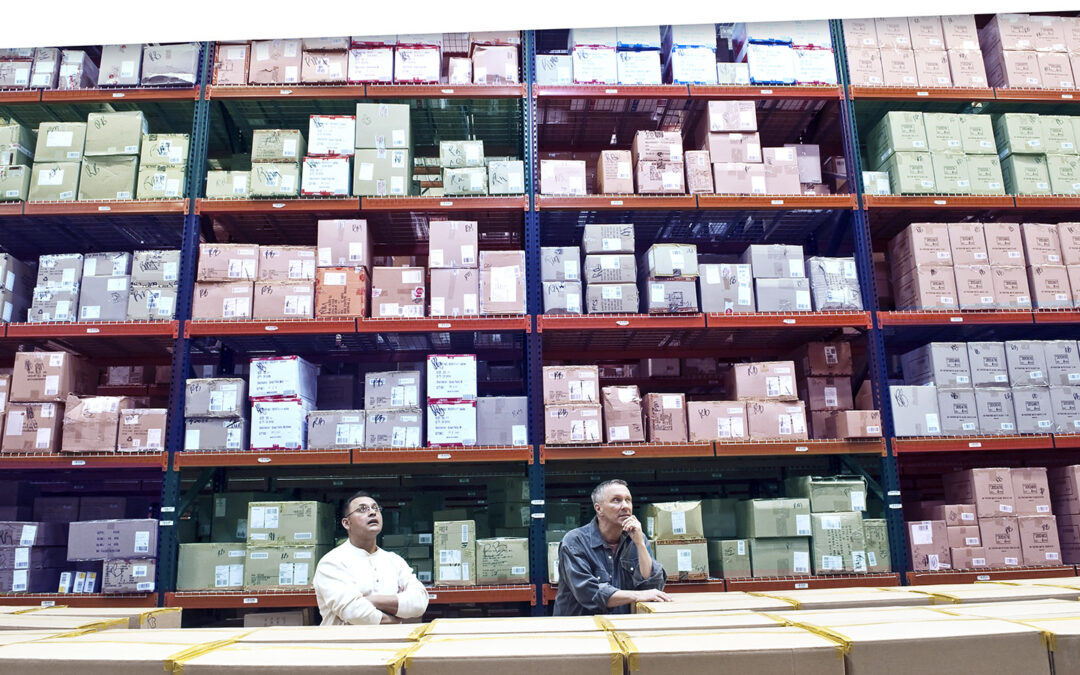
Irregular Operations
This blog is about “irregular operations.” Smart Software is in the process of adapting our products to help you cope with your own irregular ops. This is a preview.
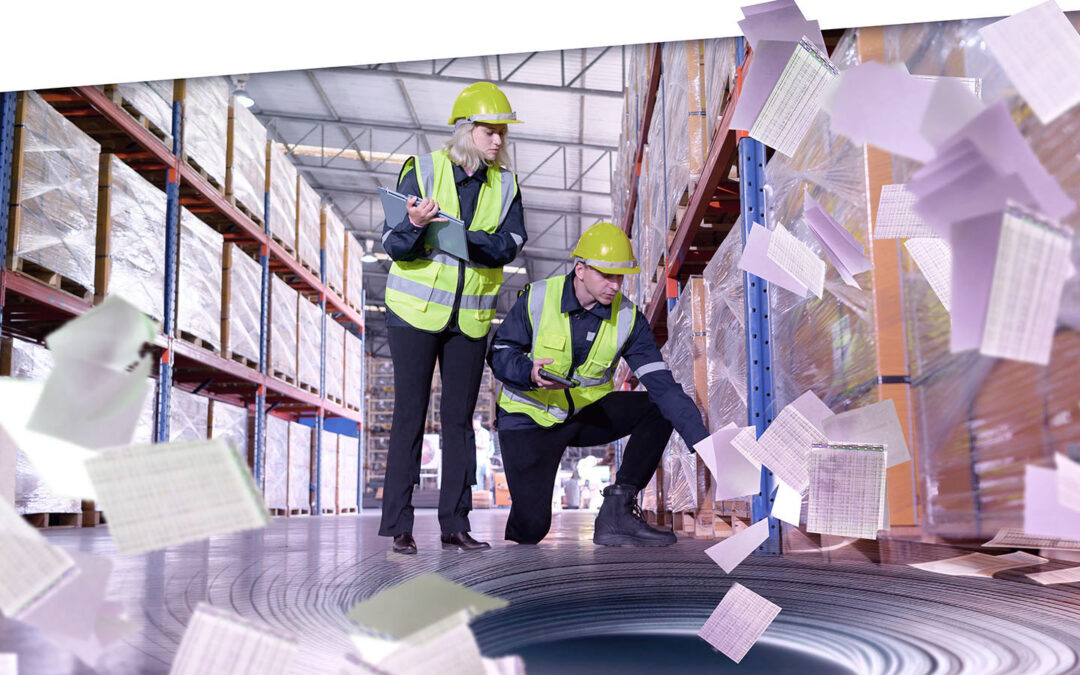
The Cost of Spreadsheet Planning
Companies that depend on spreadsheets for demand planning, forecasting, and inventory management are often constrained by the spreadsheet’s inherent limitations. This post examines the drawbacks of traditional inventory management approaches caused by spreadsheets and their associated costs, contrasting these with the significant benefits gained from embracing state-of-the-art planning technologies.
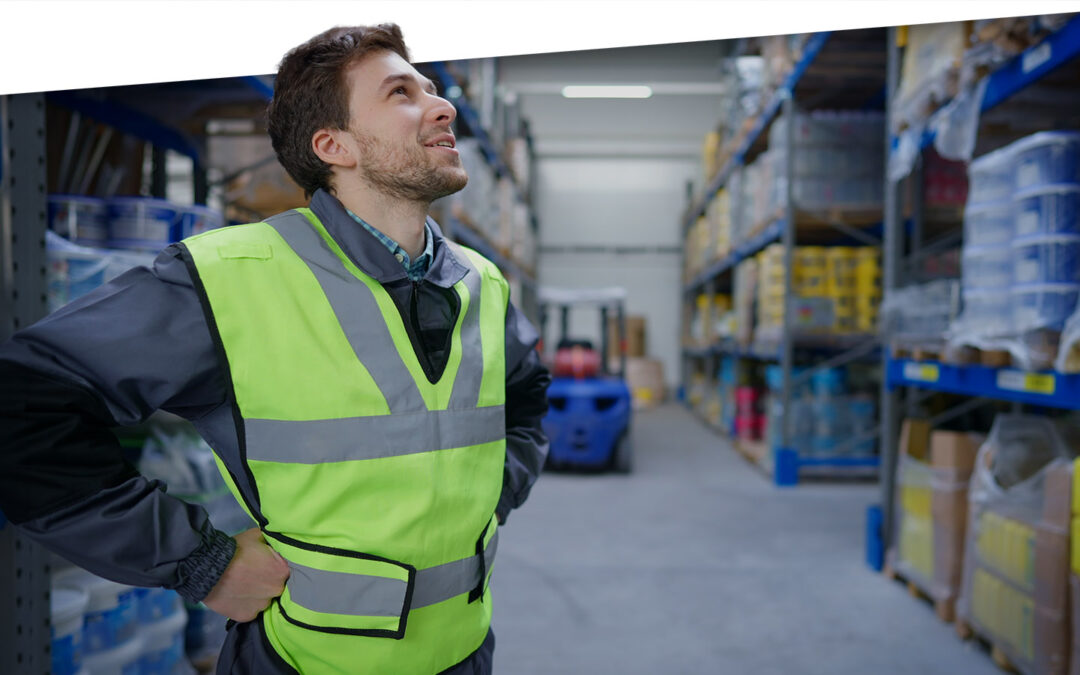
Finding Your Spot on the Inventory Tradeoff Curve
This video blog holds essential insights for those working with the complexities of inventory management. The session focuses on striking the right balance within the inventory tradeoff curve, inviting viewers to understand the deep-seated importance of this equilibrium.