A new metric we call the “Attention Index” will help forecasters identify situations where “data behaving badly” can distort automatic statistical forecasts (see adjacent poem). It quickly identifies those items most likely to require forecast overrides—providing a more efficient way to put business experience and other human intelligence to work maximizing the accuracy of forecasts. How does it work?
Classical forecasting methods, such as the various flavors of exponential smoothing and moving averages, insist on a leap of faith. They require that we trust present conditions to persist into the future. If present conditions do persist, then it is sensible to use these extrapolative methods—methods which quantify the current level, trend, seasonality and “noise” of a time series and project them into the future.
But if they do not persist, extrapolative methods can get us into trouble. What had been going up might suddenly be going down. What used to be centered around one level might suddenly jump to another. Or something really odd might happen that is entirely out of pattern. In these surprising circumstances, forecast accuracy deteriorates, inventory calculations go wrong and general unhappiness ensues.
One way to cope with this problem is to rely on more complex forecasting models that account for external factors that drive the variable being forecasted. For instance, sales promotions attempt to disrupt buying patterns and move them in a positive direction, so including promotion activity in the forecasting process can improve sales forecasting. Sometimes macroeconomic indicators, such as housing starts or inflation rates, can be used to improve forecast accuracy. But more complex models require more data and more expertise, and they may not be useful for some problems—such as managing parts or subsystems, rather than finished goods.
If one is stuck using simple extrapolative methods, it is useful to have a way to flag items that will be difficult to forecast. This is the Attention Index. As the name suggests, items to be forecast with a high Attention Index require special handling—at least a review, and usually some sort of forecast adjustment.
The Attention Index detects three types of problems:
An outlier in the demand history of an item.
An abrupt change in the level of an item.
An abrupt change in the trend of an item.
Using software like SmartForecasts™, the forecaster can deal with an outlier by replacing it with a more typical value.
An abrupt change in level or trend can be dealt with by omitting, from the forecasting calculations, all data from before the “rupture” in the demand pattern—assuming that the item has switched into a new regime that renders the older data irrelevant.
While no index is perfect, the Attention Index does a good job of focusing attention on the most problematic demand histories. This is demonstrated in the two figures below, which were produced with data from the M3 Competition, well known in the forecasting world. Figure 1 shows the 20 items (out of the contest’s 3,003) with the highest Attention Index scores; all of these have grotesque outliers and ruptures. Figure 2 shows the 20 items with the lowest Attention Index scores; most (but not all) of the items with low scores have relatively benign patterns.
If you have thousands of items to forecast, the new Attention Index will be very useful for focusing your attention on those items most likely to be problematic.
Thomas Willemain, PhD, co-founded Smart Software and currently serves as Senior Vice President for Research. Dr. Willemain also serves as Professor Emeritus of Industrial and Systems Engineering at Rensselaer Polytechnic Institute and as a member of the research staff at the Center for Computing Sciences, Institute for Defense Analyses.
Related Posts
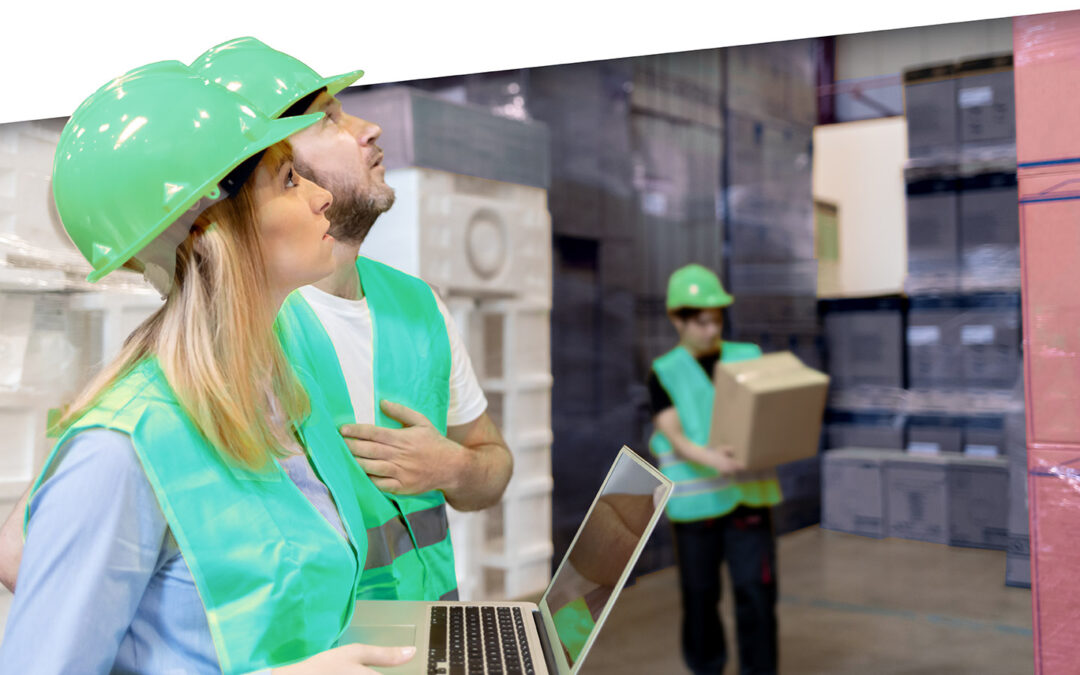
Looking for Trouble in Your Inventory Data
In this video blog, the spotlight is on a critical aspect of inventory management: the analysis and interpretation of inventory data. The focus is specifically on a dataset from a public transit agency detailing spare parts for buses.
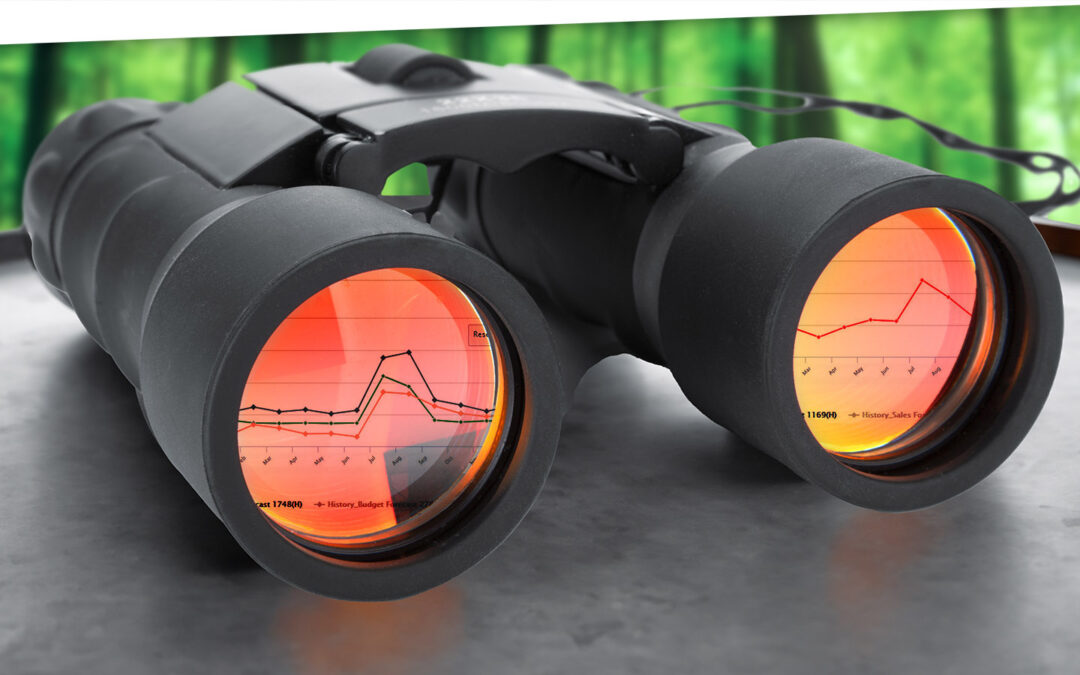
Can Randomness be an Ally in the Forecasting Battle?
When we try to understand the complex world of logistics, randomness plays a pivotal role. This introduces an interesting paradox: In a reality where precision and certainty are prized, could the unpredictable nature of supply and demand actually serve as a strategic ally?
The quest for accurate forecasts is not just an academic exercise; it’s a critical component of operational success across numerous industries. For demand planners who must anticipate product demand, the ramifications of getting it right—or wrong—are critical. Hence, recognizing and harnessing the power of randomness isn’t merely a theoretical exercise; it’s a necessity for resilience and adaptability in an ever-changing environment.
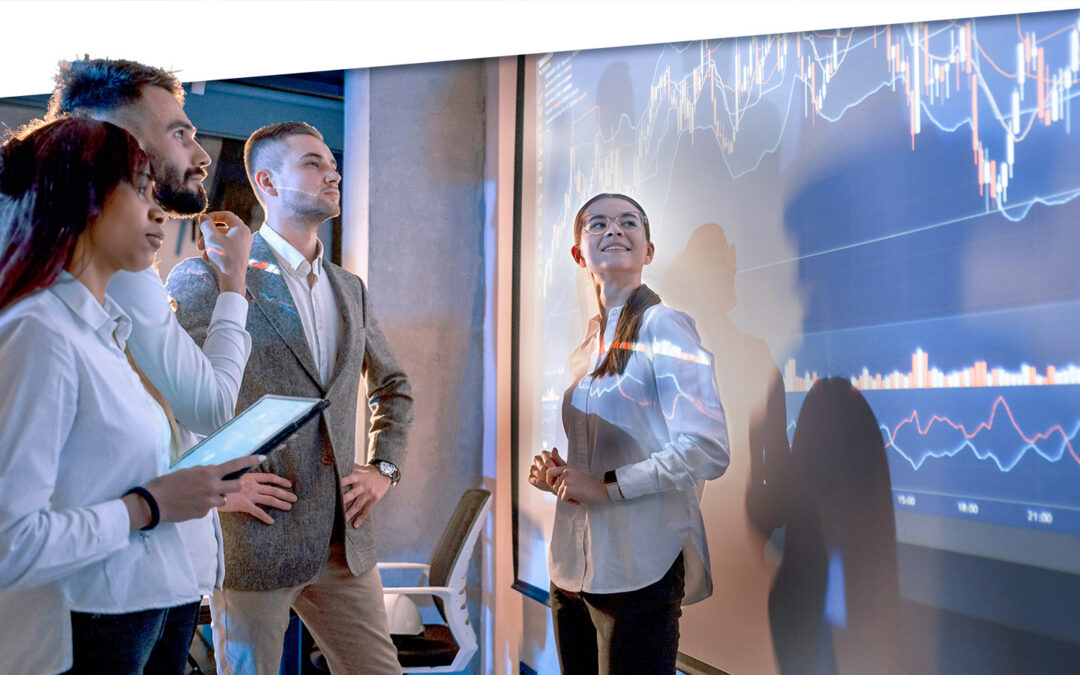
The Objectives in Forecasting
A forecast is a prediction about the value of a time series variable at some time in the future. For instance, one might want to estimate next month’s sales or demand for a product item. A time series is a sequence of numbers recorded at equally spaced time intervals; for example, unit sales recorded every month. The objectives you pursue when you forecast depend on the nature of your job and your business. Every forecast is uncertain; in fact, there is a range of possible values for any variable you forecast. Values near the middle of this range have a higher likelihood of actually occurring, while values at the extremes of the range are less likely to occur.