Tremendous cost-saving efficiencies can result from optimizing inventory stocking levels using the best predictions of future demand. Familiarity with forecasting basics is an important part of being effective with the software tools designed to exploit this efficiency. This concise introduction (the first in a short series of blog posts) offers the busy professional a primer in the basic ideas you need to bring to bear on forecasting. How do you evaluate your forecasting efforts, and how reliable are the results?
A good forecast is “unbiased.” It correctly captures predictable structure in the demand history, including: trend (a regular increase or decrease in demand); seasonality (cyclical variation); special events (e.g. sales promotions) that could impact demand or have a cannibalization effect on other items; and other, macroeconomic events.
By “unbiased,” we mean that the estimated forecast is not projecting too high or too low; the actual demand is equally likely to be above or below predicted demand. Think of the forecast as your best guess of what could happen in the future. If that forecast is “unbiased,” the overall picture will show that measures of actual future demand will “bracket” the forecasts—distributed in balance above and below predictions by the equal odds.
You can think of this as if you are an artillery officer and your job is to destroy a target with your cannon. You aim your cannon (“the forecast”) and then shoot and watch the shells fall. If you aimed the cannon correctly (producing an “unbiased” forecast), those shells will “bracket” the target; some shells will fall in front and some shells fall behind, but some shells will hit the target. The falling shells can be thought of as the “actual demand” that will occur in the future. If you forecasted well (aimed your cannon well), then those actuals will bracket the forecasts, falling equally above and below the forecast.
Once you have obtained an “unbiased” forecast (in other words, you aimed your cannon correctly), the question is: how accurate was your forecast? Using the artillery example, how wide is the range around the target in which your shells are falling? You want to have as narrow a range as possible. A good forecast will be one with the minimal possible “spread” around the target.
However, just because the actuals are falling widely around the forecast does not mean you have a bad forecast. It may merely indicate that you have very “volatile” demand history. Again, using the artillery example, if you are starting to shoot in a hurricane, you should expect the shells to fall around the target with a wide error.
Your goal is to obtain as accurate a forecast as is possible with the data you have. If that data is very volatile (you’re shooting in a hurricane), then you should expect a large error. If your data is stable, then you should expect a small error and your actuals will fall close to the forecast—you’re shooting on a clear day!
So that you can understand both the usefulness of your forecasts and the degree of caution appropriate when applying them, you need to be able to review and measure how well your forecast is doing. How well is it estimating what actually occurs? SmartForecasts does this automatically by running its “sliding simulation” through the history. It simulates “forecasts” that could have occurred in the past. An older part of the history, without the most recent numbers, is isolated and used to build forecasts. Because these forecasts then “predict” what might happen in the more recent past—a period for which you already have actual demand data—the forecasts can be compared to the real recent history.
In this manner, SmartForecasts can empirically compute the actual forecast error—and those errors are needed to properly estimate safety stock. Safety stock is the amount of extra stock you need to carry in order to account for the anticipated error in your forecasts. In a subsequent essay, I’ll discuss how we use our estimated forecasts error (via the SmartForecasts sliding simulation) to correctly estimate safety stocks.
Nelson Hartunian, PhD, co-founded Smart Software, formerly served as President, and currently oversees it as Chairman of the Board. He has, at various times, headed software development, sales and customer service.
Related Posts
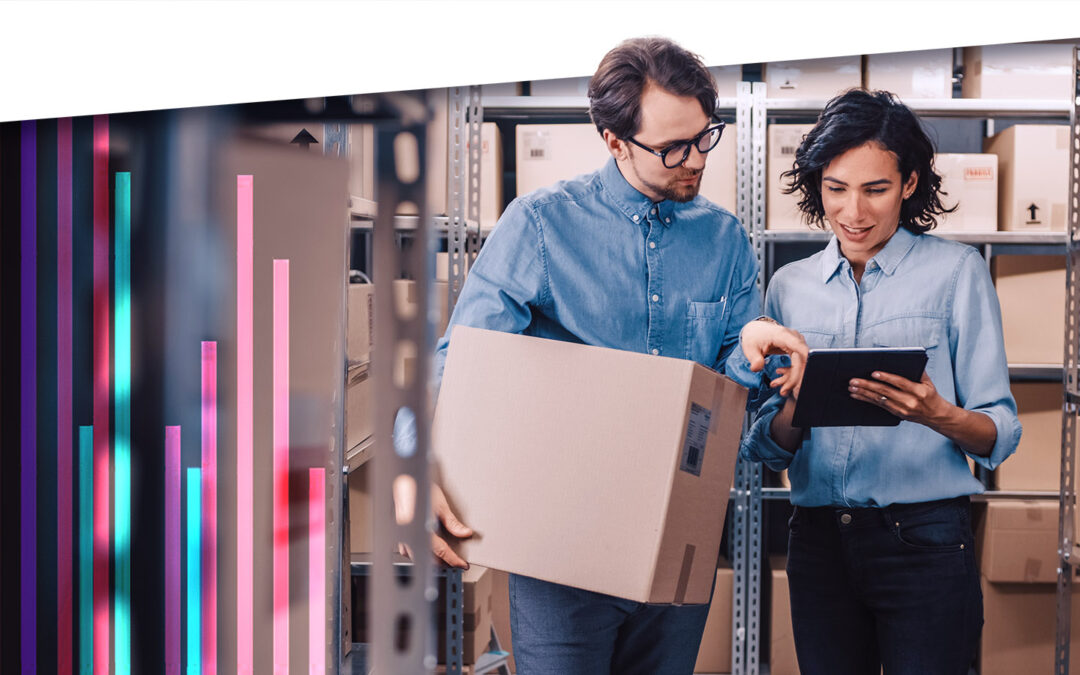
Forecast-Based Inventory Management for Better Planning
Forecast-based inventory management, or MRP (Material Requirements Planning) logic, is a forward-planning method that helps businesses meet demand without overstocking or understocking. By anticipating demand and adjusting inventory levels, it maintains a balance between meeting customer needs and minimizing excess inventory costs. This approach optimizes operations, reduces waste, and enhances customer satisfaction.
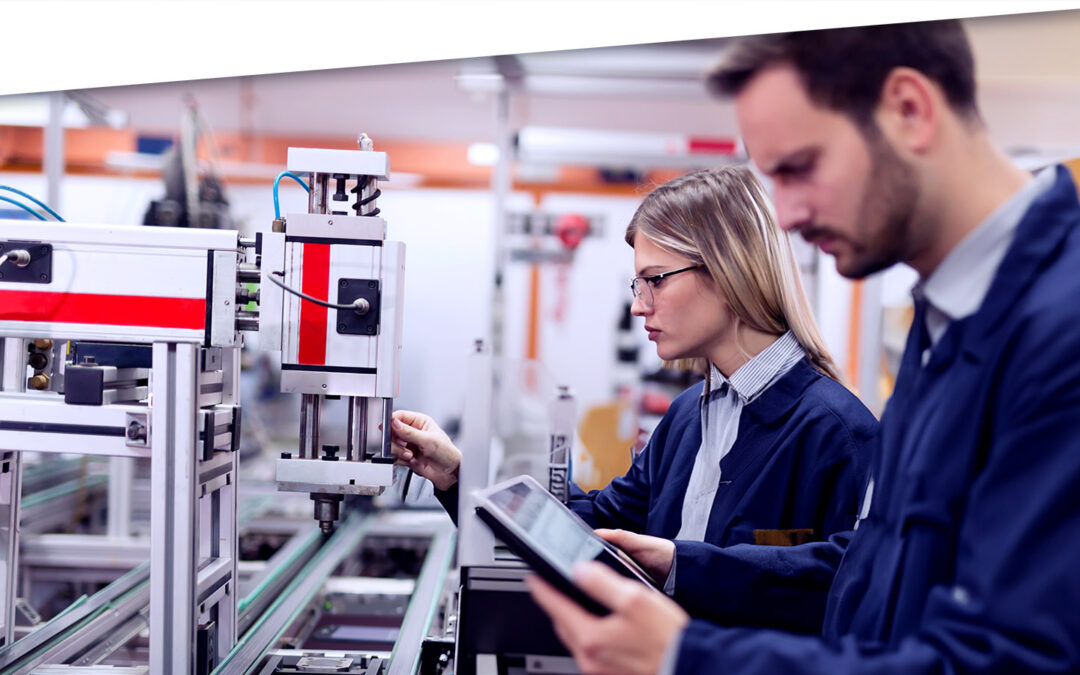
Leveraging Epicor Kinetic Planning BOMs with Smart IP&O to Forecast Accurately
In this blog, we explore how leveraging Epicor Kinetic Planning BOMs with Smart IP&O can transform your approach to forecasting in a highly configurable manufacturing environment. Discover how Smart, a cutting-edge AI-driven demand planning and inventory optimization solution, can simplify the complexities of predicting finished goods demand, especially when dealing with interchangeable components. Learn how Planning BOMs and advanced forecasting techniques enable businesses to anticipate customer needs more accurately, ensuring operational efficiency and staying ahead in a competitive market.
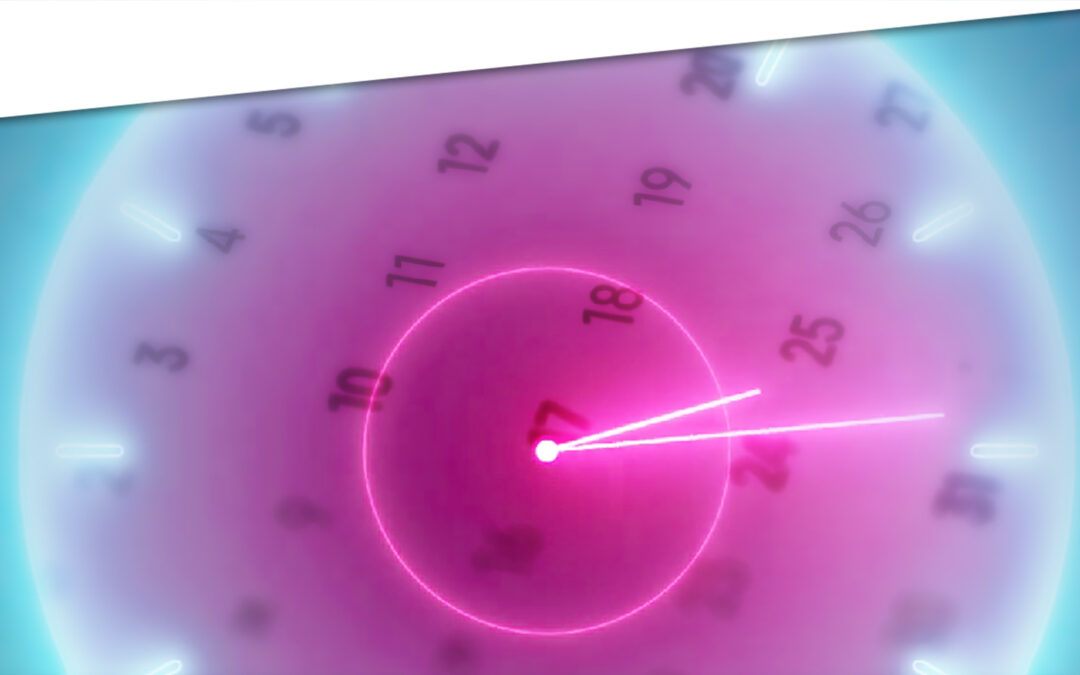
Daily Demand Scenarios
In this Videoblog, we will explain how time series forecasting has emerged as a pivotal tool, particularly at the daily level, which Smart Software has been pioneering since its inception over forty years ago. The evolution of business practices from annual to more refined temporal increments like monthly and now daily data analysis illustrates a significant shift in operational strategies.