Mastering Automatic Forecasting for Time Series Data
In this blog, we will analyze the automatic forecasting for time series demand projections, focusing on key techniques, challenges, and best practices. There are multiple methods to predict future demand for an item, and this becomes complex when dealing with thousands of items, each requiring a different forecasting technique due to their unique demand patterns. Some items have stable demand, others trend upwards or downwards, and some exhibit seasonality. Selecting the right method for each item can be overwhelming. Here, we’ll explore how automatic forecasting simplifies this process.
Automatic forecasting becomes fundamental in managing large-scale demand projections. With thousands of items, manually selecting a forecasting method for each is impractical. Automatic forecasting uses software to make these decisions, ensuring accuracy and efficiency in the forecasting process. It’s importance lies in its ability to handle complex, large-scale forecasting needs efficiently. It eliminates the need for manual selection, saving time and reducing errors. This approach is particularly beneficial in environments with diverse demand patterns, where each item may require a different forecasting method.
Key Considerations for Effective Forecasting
- Challenges of Manual Forecasting:
- Infeasibility: Manually choosing forecasting methods for thousands of items is unmanageable.
- Inconsistency: Human error can lead to inconsistent and inaccurate forecasts.
- Criteria for Method Selection:
- Error Measurement: The primary criterion for selecting a forecasting method is the typical forecast error, defined as the difference between predicted and actual values. This error is averaged over the forecast horizon (e.g., monthly forecasts over a year).
- Holdout Analysis: This technique simulates the process of waiting for a year to elapse by hiding some historical data, making forecasts, and then revealing the hidden data to compute errors. This helps in choosing the best method in real-time.
- Forecasting Tournament:
- Method Comparison: Different methods compete to forecast each item, with the method producing the lowest average error winning.
- Parameter Tuning: Each method is tested with various parameters to find the optimal settings. For example, simple exponential smoothing may be tried with different weighting factors.
The Algorithms Behind Effective Automatic Forecasting
Automatic forecasting is highly computational but feasible with modern technology. The process involves:
- Data Segmentation: Dividing historical data into segments helps manage and leverage different aspects of historical data for more accurate forecasting. For instance, for a product with seasonal demand, data might be segmented by seasons to capture season-specific trends and patterns. This segmentation allows forecasters to make and test forecasts more effectively.
- Repeated Simulations: Using sliding simulations involves repeatedly testing and refining forecasts over different periods. This method validates the accuracy of forecasting methods by applying them to different segments of data. An example is the sliding window method, where a fixed-size window moves across the time series data, generating forecasts for each position to evaluate performance.
- Parameter Optimization: Parameter optimization involves trying multiple variants of each forecasting method to find the best-performing one. By adjusting parameters, such as the smoothing factor in exponential smoothing methods or the number of past observations in ARIMA models, forecasters can fine-tune models to improve performance.
For instance, in our software, we allow various forecasting methods to compete for the best performance on a given item. Knowledge of Automatic forecasting immediately carries over to Simple Moving Average, linear moving average, Single Exponential Smoothing, Double Exponential Smoothing, Winters’ Exponential Smoothing, and Promo forecasting. This competition ensures that the most suitable method is selected based on empirical evidence, not subjective judgment. The tournament winner is the closest method to predicting new data values from old. Accuracy is measured by average absolute error (that is, the average error, ignoring any minus signs). The average is computed over a set of forecasts, each using a portion of the data, in a process known as sliding simulation, which we have explained previously in a previous blog.
Methods used in Automatic forecasting
Normally, there are six extrapolative forecasting methods competing in the Automatic forecasting tournament:
- Simple moving average
- Linear moving average
- Single exponential smoothing
- Double exponential smoothing
- Additive version of Winters’ exponential smoothing
- Multiplicative version of Winters’ exponential smoothing
The latter two methods are appropriate for seasonal series; however, they are automatically excluded from the tournament if there are fewer than two full seasonal cycles of data (for example, fewer than 24 periods of monthly data or eight periods of quarterly data). These six classical, smoothing-based methods have proven themselves to be easy to understand, easy to compute and accurate. You can exclude any of these methods from the tournament if you have a preference for some of the competitors and not others.
Automatic forecasting for time series data is essential for managing large-scale demand projections efficiently and accurately. Businesses can achieve better forecast accuracy and streamline their planning processes by automating the selection of forecasting methods and utilizing techniques like holdout analysis and forecasting tournaments. Embracing these advanced forecasting techniques ensures that businesses stay ahead in dynamic market environments, making informed decisions based on reliable data projections.
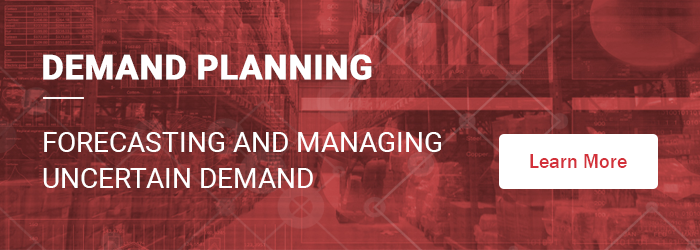
Smart Software VP of Research to Present at Business Analytics Conference, INFORMS 2022
Dr. Tom Willemain to lead INFORMS session “Dominating The Inventory Battlefield: Fighting Randomness With Randomness.”
Belmont, Mass., March 2022 – Smart Software, Inc., provider of industry-leading demand forecasting, planning, and inventory optimization solutions, today announced that Tom Willemain, Vice President for Research, will present at the INFORMS Business Analytics Conference, April 3-5, 2022, in Houston, TX.
Dr. Willemain will present a session on how next-generation analytics arms supply chain leaders in manufacturing, distribution, and MRO with tools to fight against randomness in demand and supply. During his session he will detail the following technologies:
(1) Regime change filtering to maintain data relevance against sudden shifts in the operating environment.
(2) Bootstrapping methods to generate large numbers of realistic demand and lead time scenarios to fuel models.
(3) Discrete event simulations to process the input scenarios and expose the links between management actions and key performance indicators.
(4) Stochastic optimization based on simulation experiments to tune each item for best results.
Without the analytics, inventory owners have two choices: sticking with rigid operating policies usually based on outdated and invalid rules of thumb or resorting to subjective, gut-feel guesswork that may not help and does not scale.
As the leading Business Analytics Conference, INFORMS provides the opportunity to interact with the world’s top forecasting researchers and practitioners. The attendance is large enough so that the best in the field are attracted, yet small enough that you can meet and discuss one-on-one. In addition, the conference features content from leading analytics professionals who share and showcase top analytics applications that save lives, save money, and solve problems.
About Dr. Thomas Willemain
Dr. Thomas Reed Willemain served as an Expert Statistical Consultant to the National Security Agency (NSA) at Ft. Meade, MD, and as a member of the Adjunct Research Staff at an affiliated think-tank, the Institute for Defense Analyses Center for Computing Sciences (IDA/CCS). He is Professor Emeritus of Industrial and Systems Engineering at Rensselaer Polytechnic Institute, having previously held faculty positions at Harvard’s Kennedy School of Government and Massachusetts Institute of Technology. He is also co-founder and Senior Vice President/Research at Smart Software, Inc. He is a member of the Association of Former Intelligence Officers, the Military Operations Research Society, the American Statistical Association, and several other professional organizations. Willemain received the BSE degree (summa cum laude, Phi Beta Kappa) from Princeton University and the MS and Ph.D. degrees from Massachusetts Institute of Technology. His other books include: Statistical Methods for Planners, Emergency Medical Systems Analysis (with R. C. Larson), and 80 articles in peer-reviewed journals on statistics, operations research, health care, and other topics. For more information, email: TomW@SmartCorp.com or visit www.TomWillemain.com.
About Smart Software, Inc.
Founded in 1981, Smart Software, Inc. is a leader in providing businesses with enterprise-wide demand forecasting, planning, and inventory optimization solutions. Smart Software’s demand forecasting and inventory optimization solutions have helped thousands of users worldwide, including customers at mid-market enterprises and Fortune 500 companies, such as Disney, Arizona Public Service, and Ameren. Smart Inventory Planning & Optimization gives demand planners the tools to handle sales seasonality, promotions, new and aging products, multi-dimensional hierarchies, and intermittently demanded service parts and capital goods items. It also provides inventory managers with accurate estimates of the optimal inventory and safety stock required to meet future orders and achieve desired service levels. Smart Software is headquartered in Belmont, Massachusetts, and can be found on the World Wide Web at www.smartcorp.com.
SmartForecasts and Smart IP&O have registered trademarks of Smart Software, Inc. All other trademarks are their respective owners’ property.
For more information, please contact Smart Software, Inc., Four Hill Road, Belmont, MA 02478.
Phone: 1-800-SMART-99 (800-762-7899); FAX: 1-617-489-2748; E-mail: info@smartcorp.com
Smart Software VP of Research to Present at Business Analytics Conference, INFORMS 2021
Dr. Tom Willemain to lead INFORMS session on Generation of Probabilistic Time-Series Scenarios
Belmont, Mass., March 2021 – Smart Software, Inc., provider of industry-leading demand forecasting, planning, and inventory optimization solutions, today announced that Tom Willemain, Vice President for Research, will present at the 2021 Virtual INFORMS Business Analytics Conference from April 12 – 14.
Dr. Willemain will present a session on Probabilistic Time-Series Scenarios, and how such scenarios are used, evaluated, and automatically generated using the statistical bootstrap. Frequently, OR models supporting business decisions are feed on massive numbers of probabilistic scenarios depicting future operating conditions. For example, with business operating at ever-lower levels of aggregation and ever-higher frequencies, demand planning and inventory optimization now use models fueled by scenarios representing the randomness of product demand at a daily scale. Dr. Willemain will discuss how even trivial decision tasks such as operator education benefit from large numbers of realistic training scenarios.
As the leading Business Analytics Conference, INFORMS provides the opportunity to interact with the world’s leading forecasting researchers and practitioners. The attendance is large enough so that the best in the field are attracted, yet small enough that you can meet and discuss one-on-one. The conference features content from leading analytics professionals, who share and showcase top analytics applications that save lives, save money, and solve problems.
Furthermore, to cutting-edge analytics content, the virtual analytics conference recognizes and prioritizes the need for quality “face-to-face” interactions, networking, and collaboration in a virtual setting.
About Dr. Thomas Willemain
Dr. Thomas Reed Willemain served as an Expert Statistical Consultant to the National Security Agency (NSA) at Ft. Meade, MD, and as a member of the Adjunct Research Staff at an affiliated think-tank, the Institute for Defense Analyses Center for Computing Sciences (IDA/CCS). He is Professor Emeritus of Industrial and Systems Engineering at Rensselaer Polytechnic Institute, having previously held faculty positions at Harvard’s Kennedy School of Government and Massachusetts Institute of Technology. He is also co-founder and Senior Vice President/Research at Smart Software, Inc. He is a member of the Association of Former Intelligence Officers, the Military Operations Research Society, the American Statistical Association, and several other professional organizations. Willemain received the BSE degree (summa cum laude, Phi Beta Kappa) from Princeton University and the MS and Ph.D. degrees from Massachusetts Institute of Technology. His other books include: Statistical Methods for Planners, Emergency Medical Systems Analysis (with R. C. Larson), and 80 articles in peer-reviewed journals on statistics, operations research, health care, and other topics. For more information, email: TomW@SmartCorp.com or visit www.TomWillemain.com.
About Smart Software, Inc.
Founded in 1981, Smart Software, Inc. is a leader in providing businesses with enterprise-wide demand forecasting, planning, and inventory optimization solutions. Smart Software’s demand forecasting and inventory optimization solutions have helped thousands of users worldwide, including customers at mid-market enterprises and Fortune 500 companies, such as Disney, Siemens, Metro Transit, APS, and The American Red Cross. Smart Inventory Planning & Optimization gives demand planners the tools to handle sales seasonality, promotions, new and aging products, multi-dimensional hierarchies, and intermittently demanded service parts and capital goods items. It also provides inventory managers with accurate estimates of the optimal inventory and safety stock required to meet future orders and achieve desired service levels. Smart Software is headquartered in Belmont, Massachusetts, and can be found on the World Wide Web at www.smartcorp.com.
SmartForecasts and Smart IP&O have registered trademarks of Smart Software, Inc. All other trademarks are their respective owners’ property.
For more information, please contact Smart Software, Inc., Four Hill Road, Belmont, MA 02478.
Phone: 1-800-SMART-99 (800-762-7899); FAX: 1-617-489-2748; E-mail: info@smartcorp.com
Recommended Resource: ‘Practical Time Series Forecasting: A Hands-On Guide’, by Galit Schmueli
A readable, well-organized textbook could be invaluable to “help corporate forecasters-in-training understand the basics of time series forecasting,” as Tom Willemain notes in the conclusion to this review, originally published in Foresight: The International Journal of Applied Forecasting. Principally written for an academic audience, the review also serves inexperienced demand planning professionals by pointing them to an in-depth resource.
This neat little book aims to “introduce the reader to quantitative forecasting of time series in a practical, hands-on fashion.” For a certain kind of reader, it will doubtless succeed, and do so in a stylish way.
The author, Dr. Galit Shmueli, is the SRITNE Chaired Professor of Data Analytics and Associate Professor of Statistics and Information Systems at the Indian School of Business, Hyderabad. She has authored or coauthored several other books on applied statistics and business analytics.
The book is meant to be a text for a “mini-semester” course for graduate or upper-level undergraduate students. I think it would be a stretch to believe there is enough technical material here to serve as the basis for a graduate course, but I could see it working well for undergraduates in industrial engineering or management who have had a prior statistics course (and therefore will indeed be able to “recall that a 95% prediction interval for normally distributed errors is…”).
There are end-of-chapter exercises of appropriate size and even setups for three real-world semester projects, so instructors could use the book as envisioned by the author. The book illustrates its points using XLMiner, an Excel add-in, and students can use the free demo version for almost all the exercises. Text datasets are available from the book’s web site, which also provides a free time series analysis “dashboard” application. The author notes that other software can be used in place of XLMiner and mentions Minitab, JMP, and Rob Hyndman’s forecast library in R.
While reading this book, I was delighted by its clarity. Having spent time recently correcting the technical prose of two otherwise good graduate students, I found the writing in this book to be a refreshing contrast, making technical concepts understandable.
Another virtue of this book is its selection of topics. The technical ones are reasonably standard (smoothing methods, regression using polynomial trends, and dummy variables) but also range a bit toward the more exotic (logistic regression, neural nets, a bit of ARIMA). More impressive is the inclusion of what might be called “meta-topics” relevant to forecasting: performance assessment, an overview of alternative technical approaches, and one on the forecasting process, from definition of goals to ways to gear reports differently for managerial and technical audiences. This is the kind of forecasting wisdom we find in Chris Chatfield’s book (2004), though presented rather less tartly and with less mathematical exposition. I typically recommend Chatfield’s introductory book for more technical readers interested in getting into time series; I would recommend Shmueli’s book for a more general audience.
No review is complete without quibbles. Here are a few—too few to undo my very positive view of this impressive little book:
• The text makes a good case for “well formatted and easily readable” charts (p. 179). But I found many of the screen shots to be poorly printed and difficult to see. The book is otherwise so visually pleasing that these defects seem very out of character. It uses luxurious amounts of white space and whimsical marginal art to great effect, producing a very “light” feel that must surely help comprehension.
• The author claims (p. 115) that smoothing methods (e.g., moving averages, exponential smoothing) cannot be fully automated because “the user must specify smoothing constants.” Of course, this is not so, since there are several software packages that do this, and the text later contradicts itself on this point on page 127.
• The otherwise good discussion of autocorrelation misleads when it claims (p. 88) that negative lag-1 autocorrelation means that “high values are immediately followed by low values and vice versa.” Well, usually, but not always.
When I finished reading this book, I realized immediately that there is another target audience outside the classroom. My company often conducts training sessions on the use of our software, and these include some general background on forecasting methods and processes. If we could excise the material on XLMiner, and even if we couldn’t, this text would make a wonderful “leave behind” to help corporate forecasters-in-training understand the basics of time series forecasting. The book is so well written, well organized and well designed that it might even be read. We can certainly use it to help our new programmers understand the applications they are developing. And this book might even serve as guilty reading for a graduate student who wants to really “get” what’s going on in Box, Jenkins and Reinsel (2008).
Thomas Willemain, PhD, co-founded Smart Software and currently serves as Senior Vice President for Research. Dr. Willemain also serves as Professor Emeritus of Industrial and Systems Engineering at Rensselaer Polytechnic Institute and as a member of the research staff at the Center for Computing Sciences, Institute for Defense Analyses.
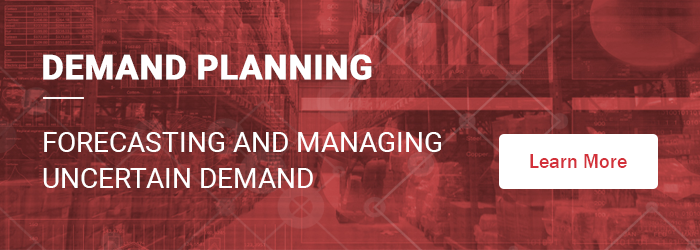
In this blog, we will explore the automatic forecasting for time series demand projections. There are multiple methods to predict future demand for an item, and this becomes complex when dealing with thousands of items, each requiring a different forecasting technique due to their unique demand patterns.
Forecast-based inventory management, or MRP (Material Requirements Planning) logic, is a forward-planning method that helps businesses meet demand without overstocking or understocking. By anticipating demand and adjusting inventory levels, it maintains a balance between meeting customer needs and minimizing excess inventory costs. This approach optimizes operations, reduces waste, and enhances customer satisfaction.
In this blog, we explore how leveraging Epicor Kinetic Planning BOMs with Smart IP&O can transform your approach to forecasting in a highly configurable manufacturing environment. Discover how Smart, a cutting-edge AI-driven demand planning and inventory optimization solution, can simplify the complexities of predicting finished goods demand, especially when dealing with interchangeable components. Learn how Planning BOMs and advanced forecasting techniques enable businesses to anticipate customer needs more accurately, ensuring operational efficiency and staying ahead in a competitive market.