Now More than Ever
Inventory optimization has become an even higher priority in recent months for many of our customers. Some are finding their products in vastly greater demand; more have the opposite problem. In either case, events like the Covid19 pandemic are forcing a reexamination of standard operating conditions, such as choices of reorder points and order quantities.
Even in quieter times, inventory control parameters like Mins and Maxes may be set far from their best values. We may ask “Why is the reorder point for SKU_1234 set at 20 units and the order quantify set at 35?” Those choices were probably the ossified result of years of accumulated guesses. A little investigation may show that the choices of 20 and 35 are no longer properly aligned with current demand level, demand volatility, supplier lead time and item costs.
The nagging feeling that “We should re-think all these choices” is often followed by “Oh no, we have to figure this out for all 10,000 items in inventory?” The savior here is advanced software that can scale up the process and make it not only desirable but feasible. The software uses sophisticated algorithms to translate changes in inventory parameters such as reorder points into key performance indicators such as service levels and operating costs (defined as the sum of holding costs, ordering costs, and shortage costs).
This blog describes how to gain the benefits of inventory optimization by outlining 4 approaches with varying degrees of automation.
Four Approaches to Inventory Optimization
Hunt-and Peck
The first way is item-specific “hunt and peck” optimization. That is, you isolate one inventory item at a time and make “what if” guesses about how to manage that item. For instance, you may ask software to evaluate what happens if you change the reorder point for SKU123 from 20 to 21 while leaving the order quantity fixed at 35. Then you might try leaving 20 alone and reducing 35 to 34. Hours later, because your intuitions are good, you may have hit on a better pair of choices, but you don’t know if there is an even better combination that you didn’t try, and you may have to move on to the next SKU and the next and the next… You need something more automated and comprehensive.
There are three ways to get the job done more productively. The first two combine your intuition with the efficiency of treating groups of related items. The third is a fully automatic search.
Service-level Driven Optimization
- Identify items that you want to all have the same service level. For instance, you might manage hundreds of “C” items and wonder whether their service level target should be 70%, or more, or less.
- Input a potential service level target and have the software predict the consequences in terms of inventory dollar investment and inventory operating cost.
- If you don’t like what you see, try another service level target until you are comfortable. Here the software does group-level predictions of the consequences of your choices, but you are still exploring your choices.
Optimization by Reallocation from a Benchmark
- Identify items that are related in some way, such as “all spares for undercarriages of light rail vehicles.”
- Use the software to assess the current spectrum of service levels and costs across the group of items. Usually, you will discover some items to be grossly overstocked (as indicated by service levels unreasonably high) and others grossly understocked (service levels embarrassingly low).
- Use the software to calculate the changes needed to lower the highest service levels and raise the lowest. This adjustment will often result in achieving two goals at once: increasing average service level while simultaneously decreasing average operating costs.
Fully automated, Item-Specific Optimization
- Identify items that all require service levels above a certain minimum. For instance, maybe you want all your “A” items to have at least a 95% service level.
- Use the software to identify, for each item, the choice of inventory parameters that will minimize the cost of meeting or exceeding the service level minimum. The software will efficiently search the “design space” defined by pairs of inventory parameters (e.g., Min and Max) for designs (e.g., Min=10, Max=23) that satisfy the service level constraint. Among those, it will identify the least cost design.
This approach goes farthest to shift the burden from the planner to the program. Many would benefit from making this the standard way they manage huge numbers of inventory items. For some items, it may be useful to put in a little more time to make sure that additional considerations are also accounted for. For instance, limited capacity in a purchasing department may force the solution away from the ideal by requiring a decrease in the frequency of orders, despite the price paid in higher overall operating costs.
Going Forward
Optimizing inventory parameters has never been more important, but it has always seemed like an impossible dream: it was too much work, and there were no good models to relate parameter choices to key performance indicators like service level and operating cost. Modern software for supply chain analytics has changed the game. Now the question is not “Why would we do that?” but “Why are we not doing that?” With software, you can connect “Here’s what we want” to “Make it so.”
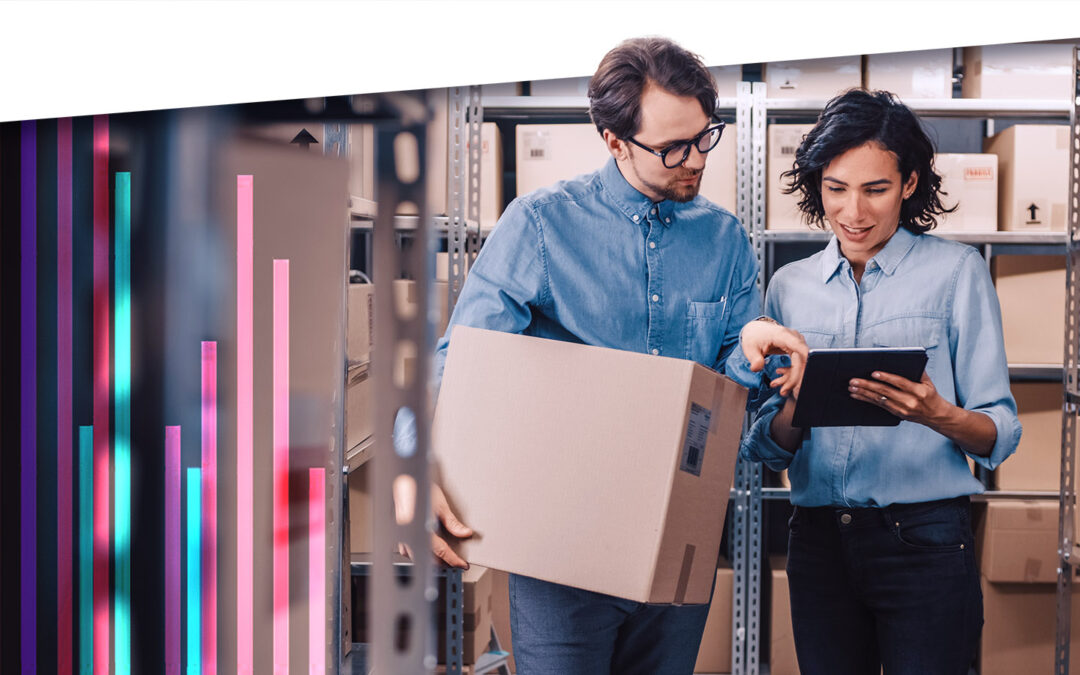
Forecast-Based Inventory Management for Better Planning
Forecast-based inventory management, or MRP (Material Requirements Planning) logic, is a forward-planning method that helps businesses meet demand without overstocking or understocking. By anticipating demand and adjusting inventory levels, it maintains a balance between meeting customer needs and minimizing excess inventory costs. This approach optimizes operations, reduces waste, and enhances customer satisfaction.
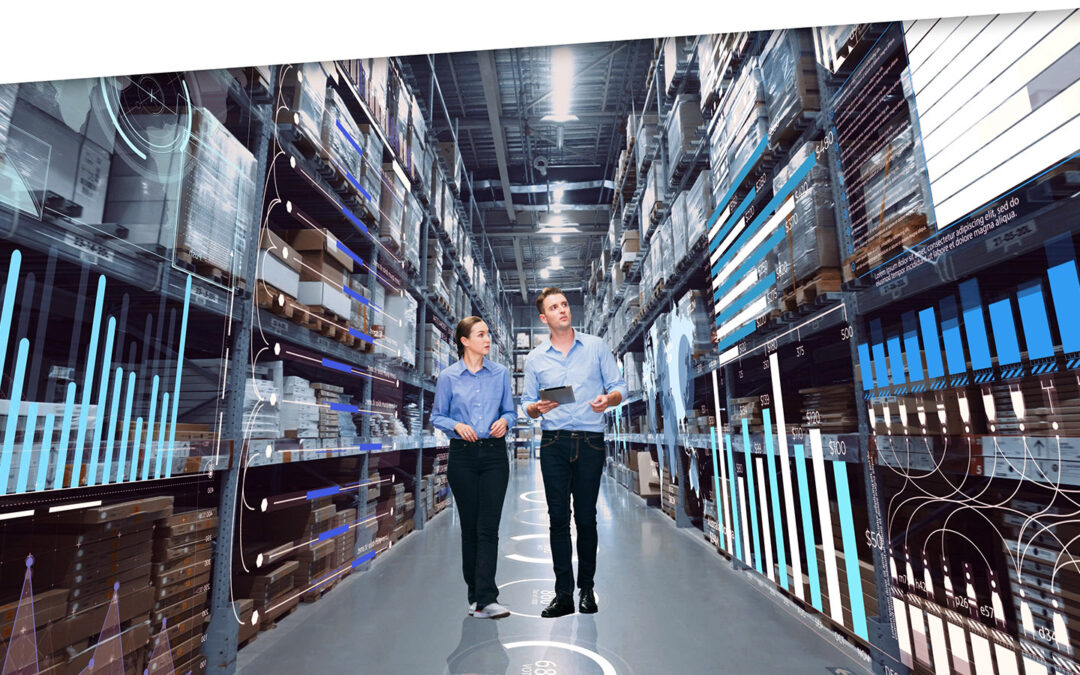
Make AI-Driven Inventory Optimization an Ally for Your Organization
In this blog, we will explore how organizations can achieve exceptional efficiency and accuracy with AI-driven inventory optimization. Traditional inventory management methods often fall short due to their reactive nature and reliance on manual processes. Maintaining optimal inventory levels is fundamental for meeting customer demand while minimizing costs. The introduction of AI-driven inventory optimization can significantly reduce the burden of manual processes, providing relief to supply chain managers from tedious tasks.
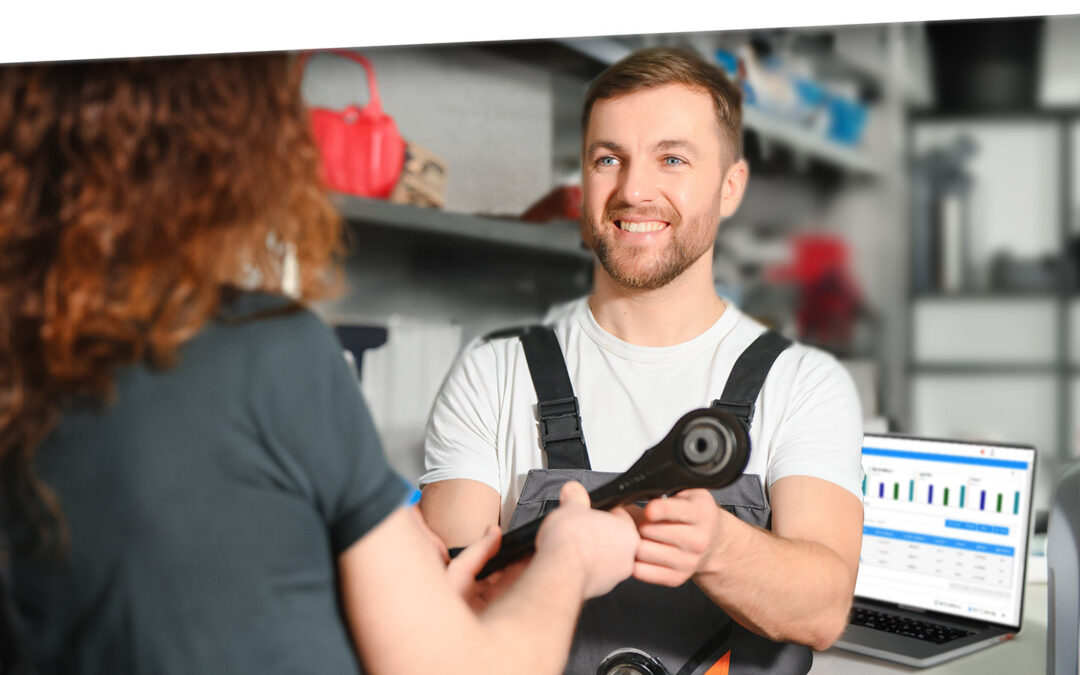
The Importance of Clear Service Level Definitions in Inventory Management
Inventory optimization software that supports what-if analysis will expose the tradeoff of stockouts vs. excess costs of varying service level targets. But first it is important to identify how “service levels” is interpreted, measured, and reported. This will avoid miscommunication and the false sense of security that can develop when less stringent definitions are used. Clearly defining how service level is calculated puts all stakeholders on the same page. This facilitates better decision-making.