Anybody doing the job knows that managing inventory can be stressful. Common stressors include: Customers with “special” requests, IT departments with other priorities, balky ERP systems running on inaccurate data, raw material shortages, suppliers with long lead times in far-away countries where production often stops for various reasons and more. This note will address one particular and ever-present source of stress: demand variability.
Everybody Has a Forecasting Problem
Suppose you manage a large fleet of spare parts. These might be surgical equipment for your hospital, or repair parts for your power station. Your mission is to maximize up time. Your enemy is down time. But because breakdowns hit at random, you are constantly in reactive mode. You might hope for rescue from forecasting technologies. But forecasts are inevitably imperfect to some degree: the element of surprise is always present. You might wait for Internet of Things (IOT) tech to be deployed on your equipment to monitor and detect impending failures, helping you schedule repairs well in advance. But you know you can’t meter up the thousands of small things that can fail and disable a big thing.
So, you decide to combine forecasting with inventory management and build buffers or safety stock to protect against surprise spikes in demand. Now you have to work out how much safety stock to maintain, knowing that too little means vulnerability and too much means bloat.
Suppose you handle finished goods inventories for a make-to-stock company. Your problem is essentially the same as in managing service parts: You have external customers and uncertain demand. But you may also have additional problems in terms of synchronizing multiple suppliers of components that you assemble into finished goods. The suppliers want you to tell them how much of their stuff to make so you can make your stuff, but you don’t know how much of your own stuff you’ll need to make.
Finally, suppose you handle finished goods in a build-to-order company. You might think that you no longer have a forecasting problem, since you don’t build until you are paid to build. But you do have a forecasting problem. Since your finished goods might be assembled from a mixture of components and sub-assemblies, you have to translate some forecast of finished goods demand to work out a forecast of those components. Otherwise, you will go to make your finished goods and discover that you don’t have a required component and have to wait until you can re-actively assemble everything you need. And your customers might not be willing to wait.
So, everybody has a forecasting problem.
What Makes Forecasting Difficult
Forecasting can be quick, easy and dead accurate – as long as the world is simple. If demand for your product is 10 units every week, month after month, you can make very accurate forecasts. But life is not quite like that. If you’re lucky and life is almost like that – maybe weekly demand is more like {10, 9, 10, 8, 12, 10, 10…} — you can still make very accurate forecast and just make minor adjustments around the edges. But if life is as it more often is – maybe weekly demand looks like {0, 0, 7, 0, 0, 0, 23, 0 …} – demand forecasting is difficult indeed. The key distinction is demand variability: it’s the zigging and zagging that creates the pain.
Safety Stock Takes Over Where Forecasting Leaves Off
Statistical forecasting methods are an important part of the solution. They let you squeeze as much advantage as possible from the historical patterns of demand your company has recorded for each item. The job of forecasts is to describe what is typical, which provides the base on which to cope with randomness in demand. Statistical forecasting techniques work by finding “big picture” features in demand records, such as trend and seasonality, then projecting those into the future. They all implicitly assume that whatever patterns exist now will persist, so 5% growth will continue, and July demand will always be 20% higher than February demand. To get to that point, statistical forecasting methods use some form of averaging to smother the “noise” in the demand history.
But then the rest of the job falls on inventory management, because the atypical, random component of future demand will still be a hassle in the future. This inevitable level of uncertainty has to be handled by the “shock-absorber” called safety stock.
The same methods that produce forecasts of trend and/or seasonality can be used to estimate the amount of forecast error. This has to be done carefully using a method called “holdout analysis”. It works like this. Suppose you have 365 observations of daily demand for Item X, which has a replenishment lead time of 10 days. You want to know how many units will be demanded over some future 10-day period. You might input the first 305 days of demand history into the forecasting technique and get forecasts for the next 10 days, days 306-315.
The answer gives you one estimate of the 10-day total demand. Importantly, it also gives you one estimate of the variability around that forecast, i.e., the forecast error, the difference between what actually happened in days 306-315 and what was forecasted. Now you can repeat the process, this time using the first 306 days to forecast the next 10, the first 307 days to forecast the next 10, etc. You end up with 52 honest estimates of the variability of total demand over a 10-day lead time. Suppose 95% of those estimates are less than 28 units. Then 28 units would be a pretty safe safety stock to add to the forecast, since you will run into shortages only 5% of the time.
Modern statistical software does these calculations automatically. It can ease at least one of the chronic headaches of inventory management by helping you cope with demand variability.
Related Posts
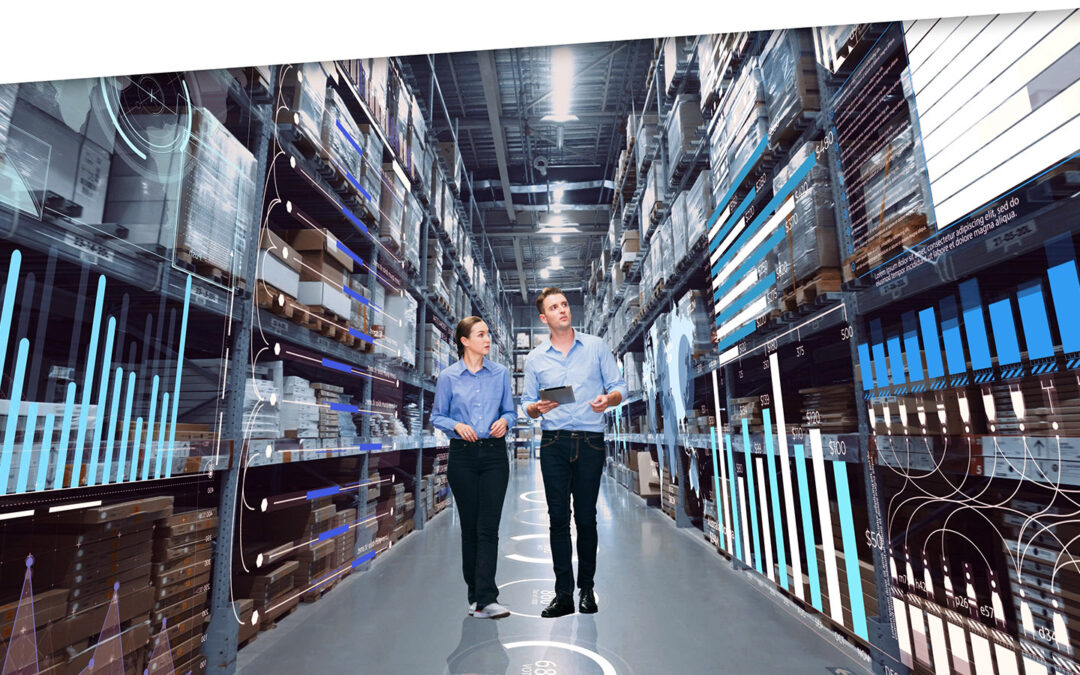
Make AI-Driven Inventory Optimization an Ally for Your Organization
In this blog, we will explore how organizations can achieve exceptional efficiency and accuracy with AI-driven inventory optimization. Traditional inventory management methods often fall short due to their reactive nature and reliance on manual processes. Maintaining optimal inventory levels is fundamental for meeting customer demand while minimizing costs. The introduction of AI-driven inventory optimization can significantly reduce the burden of manual processes, providing relief to supply chain managers from tedious tasks.
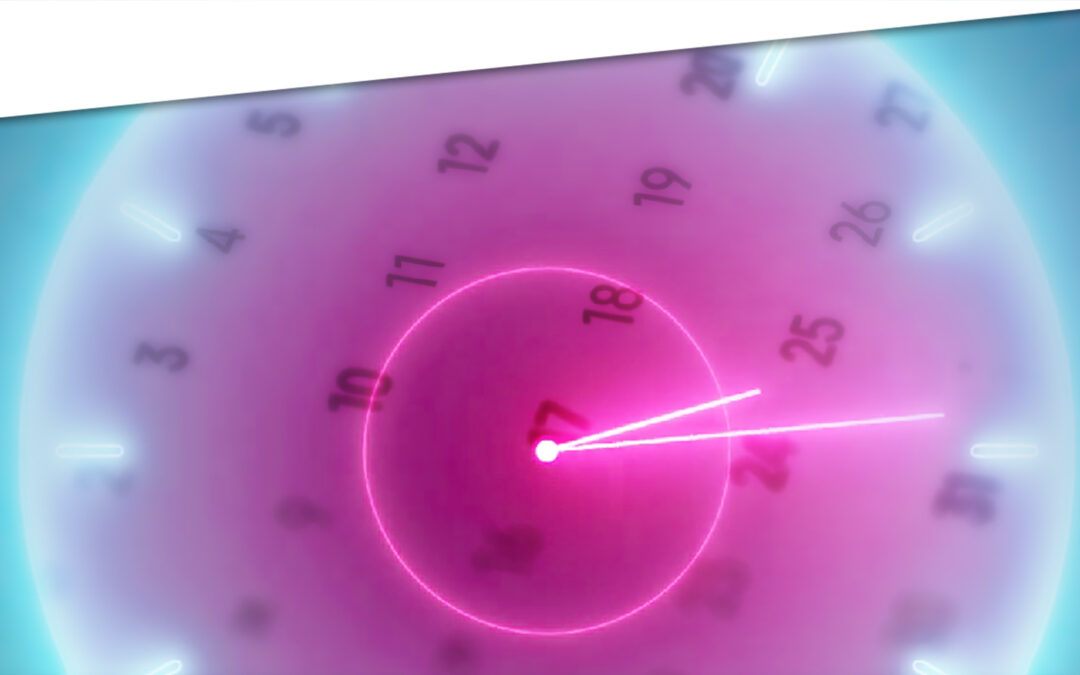
Daily Demand Scenarios
In this Videoblog, we will explain how time series forecasting has emerged as a pivotal tool, particularly at the daily level, which Smart Software has been pioneering since its inception over forty years ago. The evolution of business practices from annual to more refined temporal increments like monthly and now daily data analysis illustrates a significant shift in operational strategies.
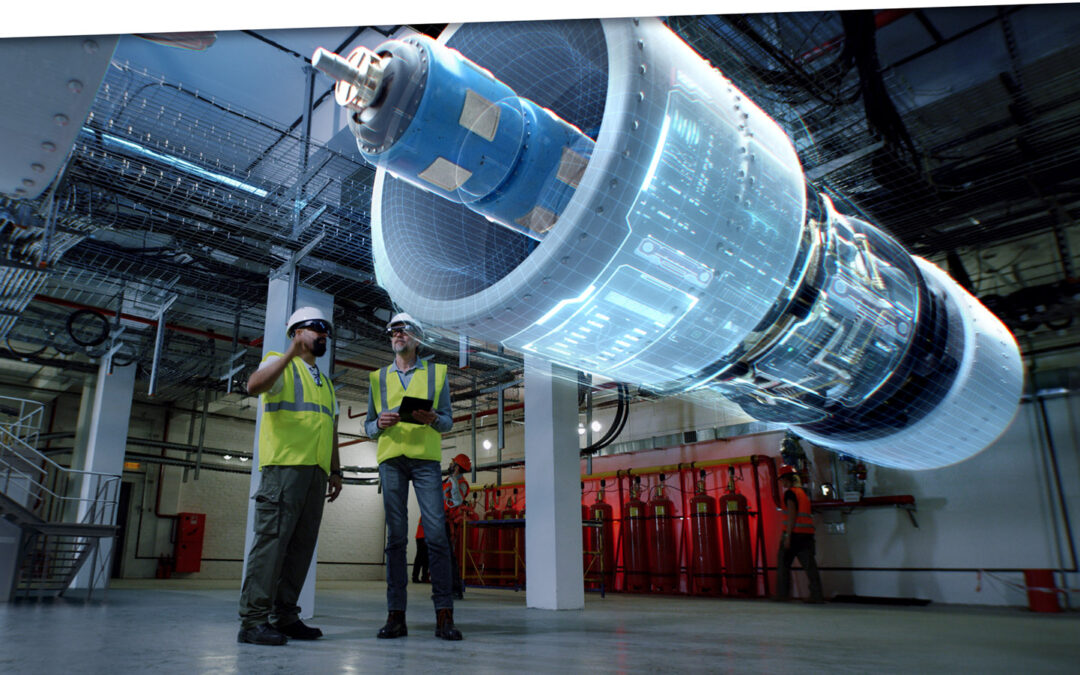
Constructive Play with Digital Twins
Those of you who track hot topics will be familiar with the term “digital twin.” Those who have been too busy with work may want to read on and catch up. While there are several definitions of digital twin, here’s one that works well: A digital twin is a dynamic virtual copy of a physical asset, process, system, or environment that looks like and behaves identically to its real-world counterpart. A digital twin ingests data and replicates processes so you can predict possible performance outcomes and issues that the real-world product might undergo.