Does your extended supply chain suffer from extreme seasonal variability? Does this situation challenge your ability to meet service level commitments to your customers? I have grappled with this at Rev-A-Shelf, addressing unusual conditions created by Chinese New Year and other global events, and would like to share the experience and a few things I learned along the way.
First, let me explain our situation. We import 60% of the parts we use to build our kitchen and bath accessories from China and Europe. Most of the year we were able to plan our inventory needs using a spreadsheet-based min/max approach. But not during Chinese New Year, which drives the planet’s greatest annual population migration. Chinese New Year shuts down production for up to two months, creating significant supply risk as we strive to meet our three day order fulfillment commitment.
We solved our problem, introducing statistical demand forecasting with the flexibility to extend lead times when necessary, the ability to reliably establish safety stocks that achieve our required service levels and a continuous reporting system that lets everyone know exactly where we stand. However, success required much more than a new piece of software. We needed to change the way we view future demand, supply risk and safety stock. Here are a few key things we did that made all the difference.
Stakeholder education and buy-in
Regardless of the project, it’s always best to enlist the buy-in of all stakeholders. We knew we had to do something to solve our problem, but there was bound to be resistance. Senior managers, for example, had developed a healthy distrust of software and wondered whether demand forecasting software could help. Our buyers had developed their own perspectives and procurement methods, and felt personally at risk as we considered new approaches.
People came around as they developed a common understanding of the problem and how we would address it. Education was a big part of the solution. We explained how forecasting works and key factors we should all understand: how to analyze trends, how to use “what if” scenarios, impact of shifting lead times, how to relate service levels to supply risk and safety stock and key performance indicators like inventory turns. Going through this process together, we all became stakeholders in the solution.
Use the Right software
When you have lots of part numbers and any sort of supply or demand variability, you just cannot forecast effectively with a spreadsheet. With our min/max forecasting system, we were planning to an average, and it wasn’t working. Average usage has inherent flaws for planning purposes—it’s always looking backward!
You need software that looks ahead, recognizes seasonal patterns and enables you to determine how much stock you’ll need to meet required service levels over varying lead times.
Fine-tune processes
When the old ways don’t work, you need to be open to adjusting your assumptions. Think less about where you’ve been, and more about where you want to be. Take a look at your lead times and plan to your desired service level. Last year’s history may not be the best predictor of this year’s demand. The same forecast horizon may not be appropriate for all products or certain time of the year.
Make the Forecast Actionable
It’s not enough to produce an accurate forecast and estimated inventory stocking levels. You’ve got to develop a way to make the information actionable for those tasked with using it. We developed a set of reports that enabled buyers to leverage better forecast and safety stock information. Now, at the end of every month, we produce a forecast report that provides a clear picture of current inventory, safety stock, past usage, forecasted usage, incoming deliveries (PO’s) and recommended order quantities.
Validate Results
You can, and we did, test our new methods against our own demand history. Still, an authoritative outsider can make acceptance easier. We commissioned a study by a professor at Louisville University’s College of Business who set one of her graduate students to the task. Through them we were able to reinforce what we saw happening from our results, and feel comfortable that we were on a good path.
All of these factors helped Rev-A-Shelf transform its demand planning process, to great effect. Today we are exceeding our service level targets, and our fill rate, based on a three day ship cycle, is showing steady improvement, and trending up. Overall, units-in-stock have stayed flat while supporting a 13% increase in sales.
John Engelhardt is currently Director of Purchasing and Asian Operations for Rev-a-Shelf, LLC in Louisville, KY. He has held a variety of management positions both in private business and public organizations. At Rev-A-Shelf he held the position of International Sales Manager and Director of Sales Support before assuming his current position. He can be reached at johne at rev-a-shelf dot com.
Related Posts
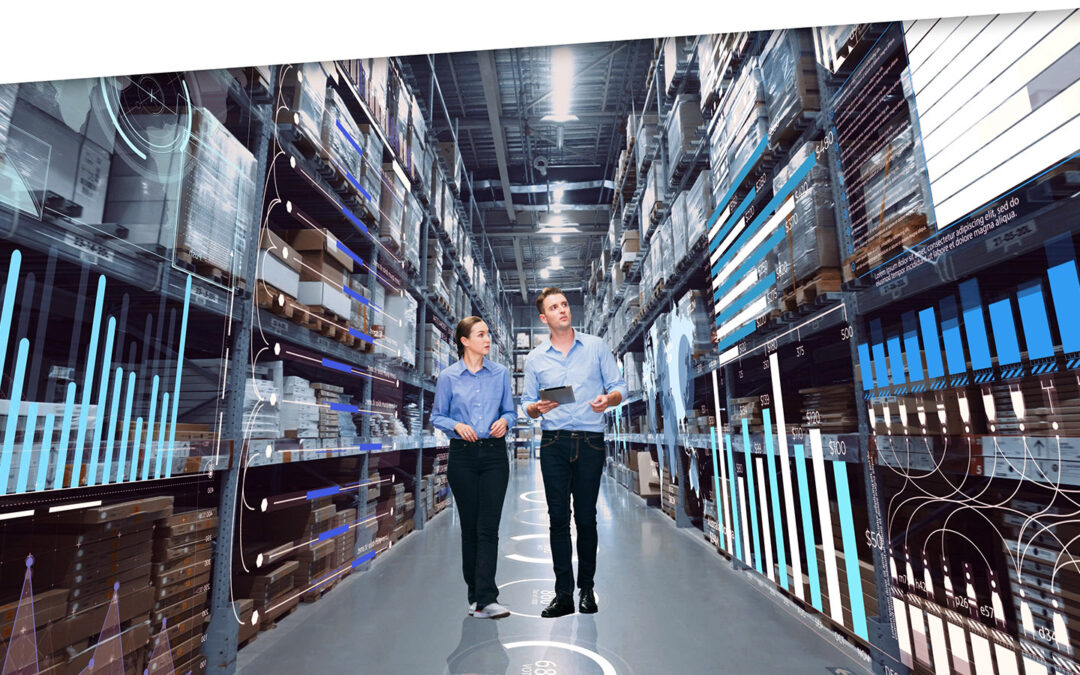
Make AI-Driven Inventory Optimization an Ally for Your Organization
In this blog, we will explore how organizations can achieve exceptional efficiency and accuracy with AI-driven inventory optimization. Traditional inventory management methods often fall short due to their reactive nature and reliance on manual processes. Maintaining optimal inventory levels is fundamental for meeting customer demand while minimizing costs. The introduction of AI-driven inventory optimization can significantly reduce the burden of manual processes, providing relief to supply chain managers from tedious tasks.

Future-Proofing Utilities: Advanced Analytics for Supply Chain Optimization
Utilities in the electrical, natural gas, urban water, and telecommunications fields are all asset-intensive and reliant on physical infrastructure that must be properly maintained, updated, and upgraded over time. Maximizing asset uptime and the reliability of physical infrastructure demands effective inventory management, spare parts forecasting, and supplier management. A utility that executes these processes effectively will outperform its peers, provide better returns for its investors and higher service levels for its customers, while reducing its environmental impact.
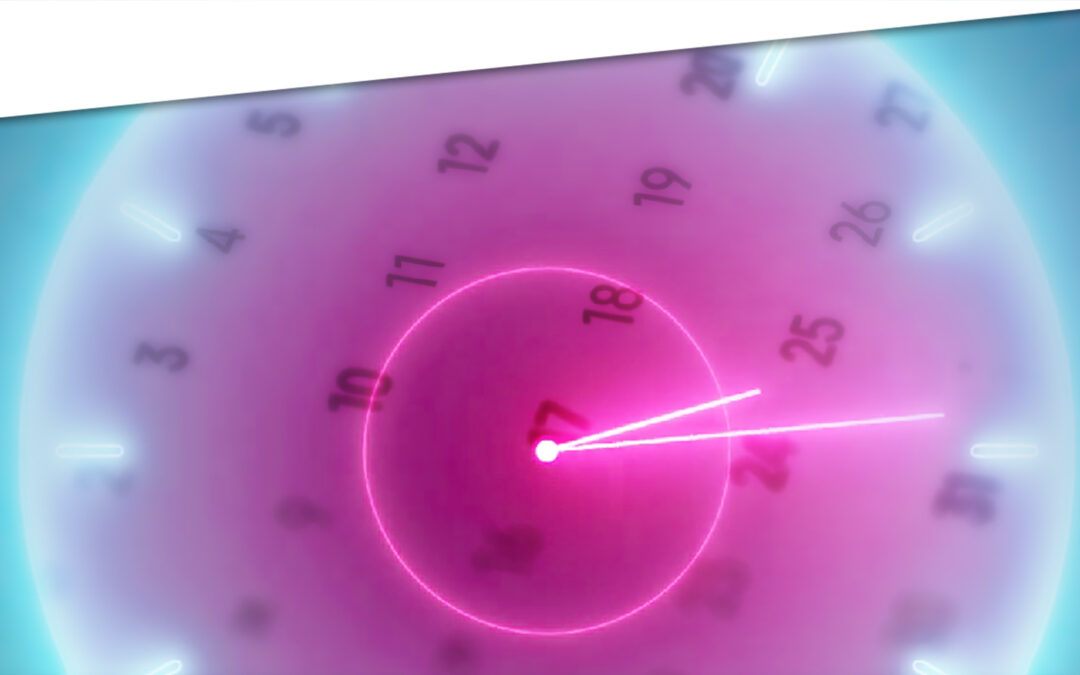
Daily Demand Scenarios
In this Videoblog, we will explain how time series forecasting has emerged as a pivotal tool, particularly at the daily level, which Smart Software has been pioneering since its inception over forty years ago. The evolution of business practices from annual to more refined temporal increments like monthly and now daily data analysis illustrates a significant shift in operational strategies.