Improve Forecast Accuracy, Eliminate Excess Inventory, & Maximize Service Levels
In this video, Dr. Thomas Willemain, co-Founder and SVP Research, talks about improving forecast accuracy by measuring forecast error. We begin by overviewing the various types of error metrics: scale-dependent error, percentage error, relative error, and scale-free error metrics. While some error is inevitable, there are ways to reduce it, and forecast metrics are necessary aids for monitoring and improving forecast accuracy. Then we will explain the special problem of intermittent demand and divide-by-zero problems. Tom concludes by explaining how to assess forecasts of multiple items and how it often makes sense to use weighted averages, weighting items differently by volume or revenue.
Four general types of error metrics
1. Scale-dependent error
2. Percentage error
3. Relative error
4 .Scale-free error
Remark: Scale-dependent metrics are expressed in the units of the forecasted variable. The other three are expresses as percentages.
1. Scale-dependent error metrics
- Mean Absolute Error (MAE) aka Mean Absolute Deviation (MAD)
- Median Absolute Error (MdAE)
- Root Mean Square Error (RMSE)
- These metrics express the error in the original units of the data.
- Ex: units, cases, barrels, kilograms, dollars, liters, etc.
- Since forecasts can be too high or too low, the signs of the errors will be either positive or negative, allowing for unwanted cancellations.
- Ex: You don’t want errors of +50 and -50 to cancel and show “no error”.
- To deal with the cancellation problem, these metrics take away negative signs by either squaring or using absolute value.
2. Percentage error metric
- Mean Absolute Percentage Error (MAPE)
- This metric expresses the size of the error as a percentage of the actual value of the forecasted variable.
- The advantage of this approach is that it immediately makes clear whether the error is a big deal or not.
- Ex: Suppose the MAE is 100 units. Is a typical error of 100 units horrible? ok? great?
- The answer depends on the size of the variable being forecasted. If the actual value is 100, then a MAE = 100 is as big as the thing being forecasted. But if the actual value is 10,000, then a MAE = 100 shows great accuracy, since the MAPE is only 1% of the actual.
3. Relative error metric
- Median Relative Absolute Error (MdRAE)
- Relative to what? To a benchmark forecast.
- What benchmark? Usually, the “naïve” forecast.
- What is the naïve forecast? Next forecast value = last actual value.
- Why use the naïve forecast? Because if you can’t beat that, you are in tough shape.
4. Scale-Free error metric
- Median Relative Scaled Error (MdRSE)
- This metric expresses the absolute forecast error as a percentage of the natural level of randomness (volatility) in the data.
- The volatility is measured by the average size of the change in the forecasted variable from one time period to the next.
- (This is the same as the error made by the naïve forecast.)
- How does this metric differ from the MdRAE above?
- They do both use the naïve forecast, but this metric uses errors in forecasting the demand history, while the MdRAE uses errors in forecasting future values.
- This matters because there are usually many more history values than there are forecasts.
- In turn, that matters because this metric would “blow up” if all the data were zero, which is less likely when using the demand history.
The special problem of intermittent demand
- “Intermittent” demand has many zero demands mixed in with random non-zero demands.
- MAPE gets ruined when errors are divided by zero.
- MdRAE can also get ruined.
- MdSAE is less likely to get ruined.
Recap and remarks
- Forecast metrics are necessary aids for monitoring and improving forecast accuracy.
- There are two major classes of metrics: absolute and relative.
- Absolute measures (MAE, MdAE, RMSE) are natural choices when assessing forecasts of one item.
- Relative measures (MAPE, MdRAE, MdSAE) are useful when comparing accuracy across items or between alternative forecasts of the same item or assessing accuracy relative to the natural variability of an item.
- Intermittent demand presents divide-by-zero problems which favor MdSAE over MAPE.
- When assessing forecasts of multiple items, it often makes sense to use weighted averages, weighting items differently by volume or revenue.
RECENT POSTS
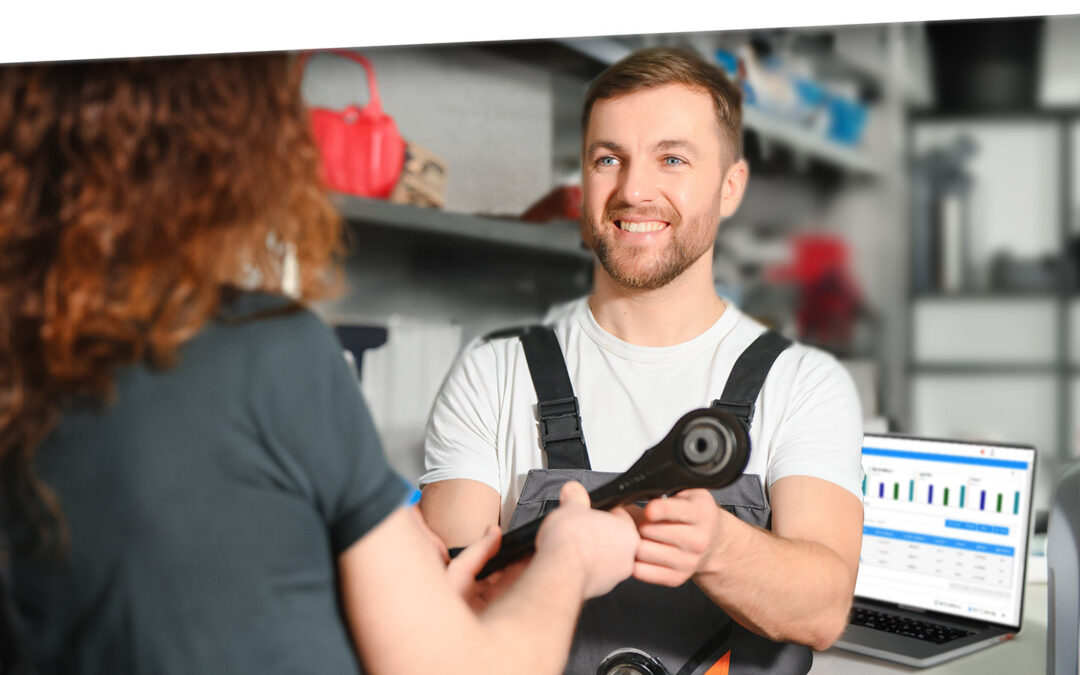
The Importance of Clear Service Level Definitions in Inventory Management
Inventory optimization software that supports what-if analysis will expose the tradeoff of stockouts vs. excess costs of varying service level targets. But first it is important to identify how “service levels” is interpreted, measured, and reported. This will avoid miscommunication and the false sense of security that can develop when less stringent definitions are used. Clearly defining how service level is calculated puts all stakeholders on the same page. This facilitates better decision-making.
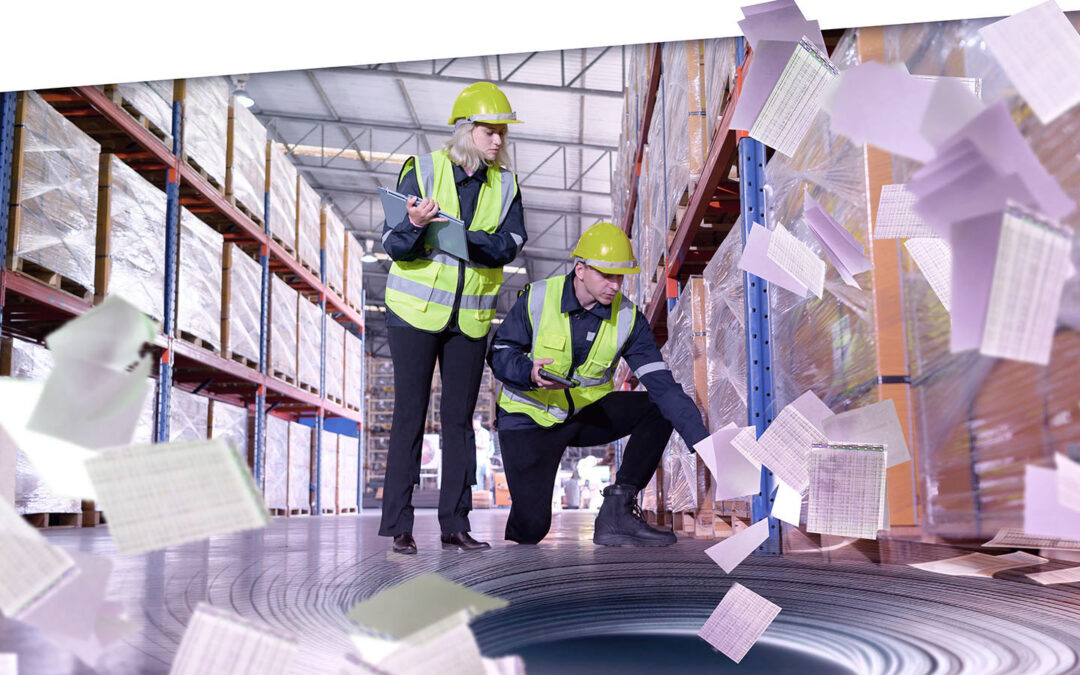
The Cost of Spreadsheet Planning
Companies that depend on spreadsheets for demand planning, forecasting, and inventory management are often constrained by the spreadsheet’s inherent limitations. This post examines the drawbacks of traditional inventory management approaches caused by spreadsheets and their associated costs, contrasting these with the significant benefits gained from embracing state-of-the-art planning technologies.
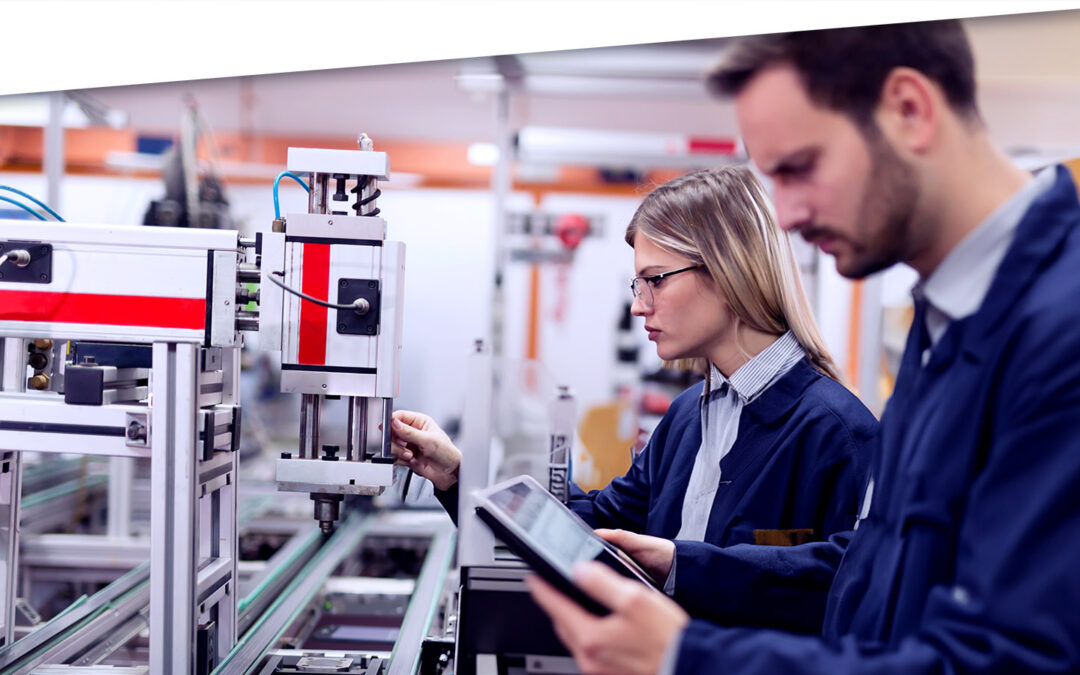
Leveraging Epicor Kinetic Planning BOMs with Smart IP&O to Forecast Accurately
In this blog, we explore how leveraging Epicor Kinetic Planning BOMs with Smart IP&O can transform your approach to forecasting in a highly configurable manufacturing environment. Discover how Smart, a cutting-edge AI-driven demand planning and inventory optimization solution, can simplify the complexities of predicting finished goods demand, especially when dealing with interchangeable components. Learn how Planning BOMs and advanced forecasting techniques enable businesses to anticipate customer needs more accurately, ensuring operational efficiency and staying ahead in a competitive market.