The unique challenges of inventory planning for spare parts, large capital goods and other infrequently or irregularly moving items drives the importance of finding smarter methods to forecast this kind of intermittent demand. Robert Bowman, Editor of Supply Chain Brain Magazine, and I discussed this topic at the October APICS conference in Denver, and video of our conversation is available at Supply Chain Brain‘s website.
Why plan for intermittent demand? Well, why plan for any demand? If you can understand what the likely range of demand will be until you can get more, you will know how much stock to keep in reserve, so you have just enough. This is the heart of demand forecasting and inventory optimization. Intermittent demand is exceptionally difficult to forecast, but this same principle holds true.
Unlike other demand patterns, where historical data suggests regular trends, ebbs and flows, seasonality or other discernible patterns, intermittent demand appears to be random. There are many periods of zero demand interspersed with irregular, non-zero demand. This occurs frequently with service parts, where parts are replaced when they break, and you just don’t know when that will occur. Most service parts inventories (70% or more!) can experience intermittent demand. Demand for specialized or configured products is also likely to be intermittent.
Supply Chain Brain has made the more in-depth discussion of this topic Bowman and I shared available here. For new visitors to Supply Chain Brain, a quick account sign-up is required to access the video.
Jeff Scott serves as Vice President, Marketing & Alliances for Smart Software.
Related Posts
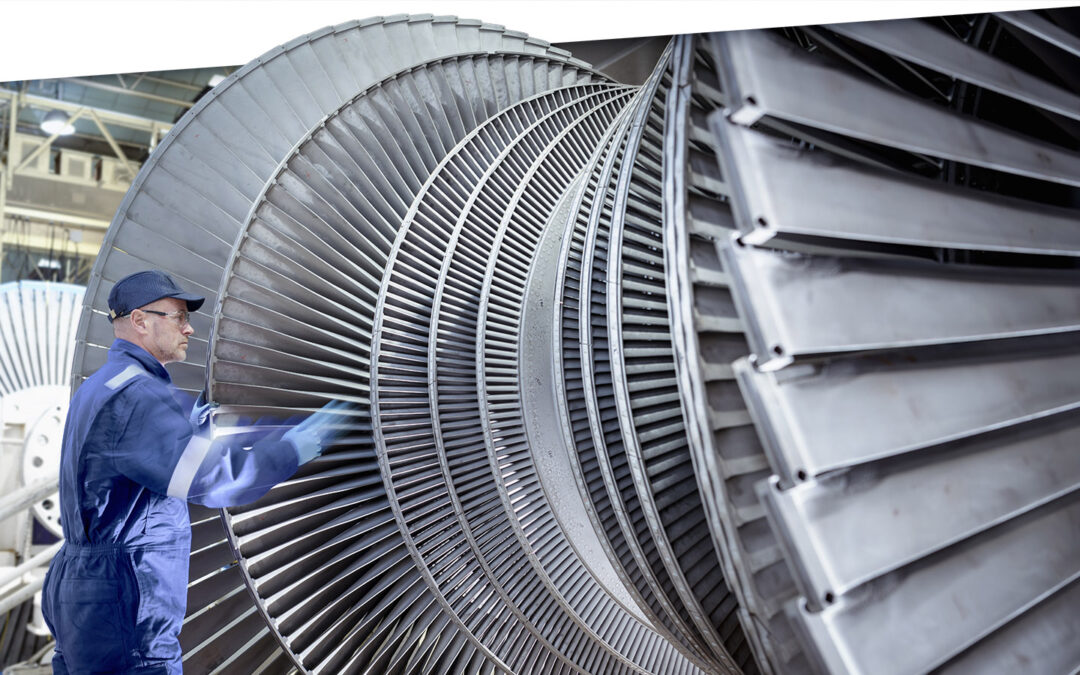
Why MRO Businesses Need Add-on Service Parts Planning & Inventory Software
MRO organizations exist in a wide range of industries, including public transit, electrical utilities, wastewater, hydro power, aviation, and mining. To get their work done, MRO professionals use Enterprise Asset Management (EAM) and Enterprise Resource Planning (ERP) systems. These systems are designed to do a lot of jobs. Given their features, cost, and extensive implementation requirements, there is an assumption that EAM and ERP systems can do it all. In this post, we summarize the need for add-on software that addresses specialized analytics for inventory optimization, forecasting, and service parts planning.
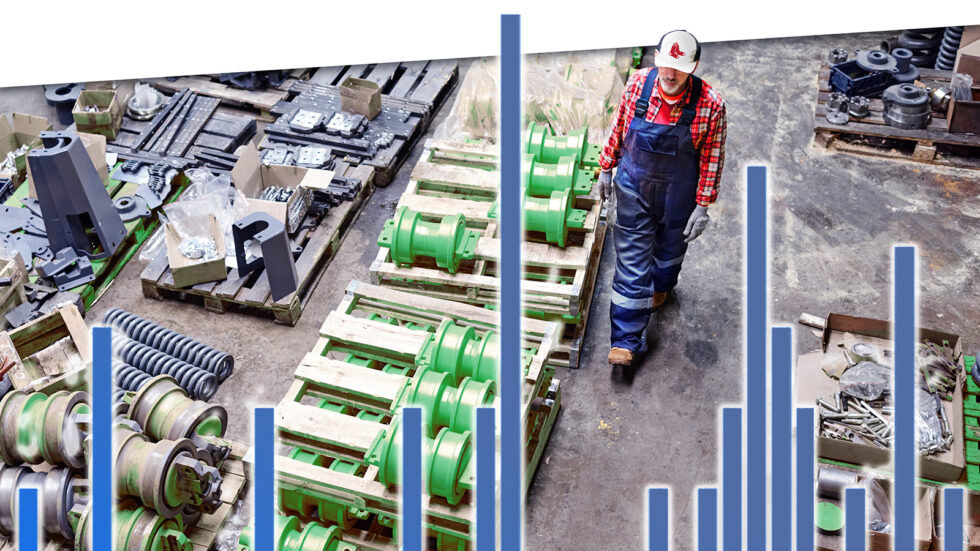
The Forecast Matters, but Maybe Not the Way You Think
True or false: The forecast doesn’t matter to spare parts inventory management. At first glance, this statement seems obviously false. After all, forecasts are crucial for planning stock levels, right? It depends on what you mean by a “forecast”. If you mean an old-school single-number forecast (“demand for item CX218b will be 3 units next week and 6 units the week after”), then no. If you broaden the meaning of forecast to include a probability distribution taking account of uncertainties in both demand and supply, then yes.
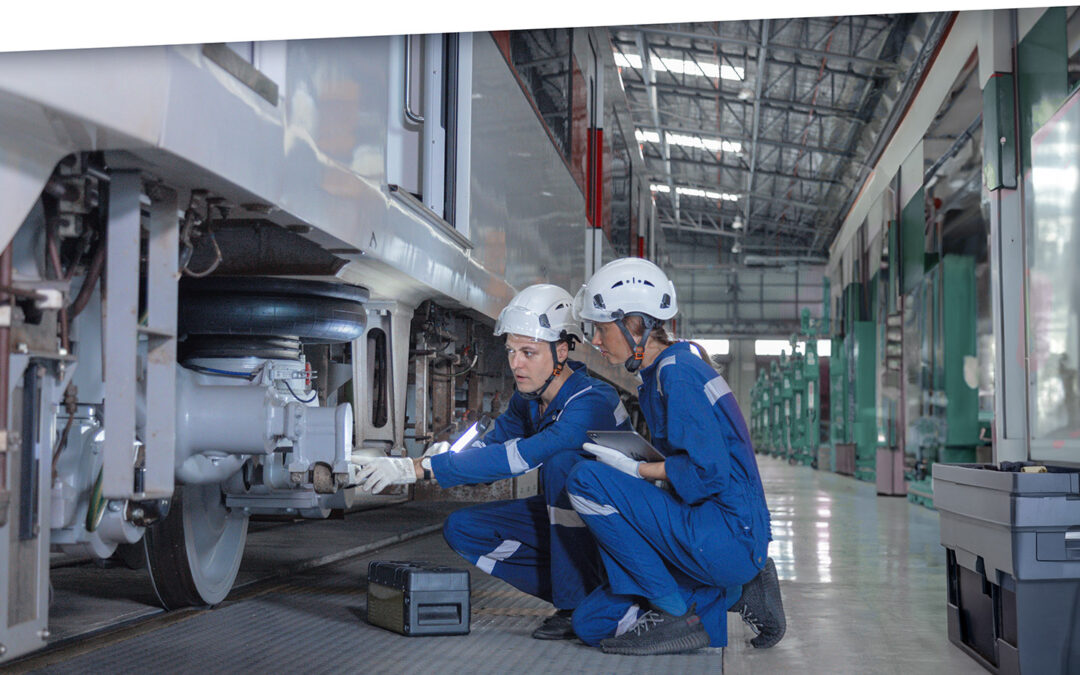
Why MRO Businesses Should Care About Excess Inventory
Do MRO companies genuinely prioritize reducing excess spare parts inventory? From an organizational standpoint, our experience suggests not necessarily. Boardroom discussions typically revolve around expanding fleets, acquiring new customers, meeting service level agreements (SLAs), modernizing infrastructure, and maximizing uptime. In industries where assets supported by spare parts cost hundreds of millions or generate significant revenue (e.g., mining or oil & gas), the value of the inventory just doesn’t raise any eyebrows, and organizations tend to overlook massive amounts of excessive inventory.