Those who produce forecasts owe it to those who consume forecasts, and to themselves, to be aware of the uncertainty in their forecasts. This note is about how to estimate forecast uncertainty and use the estimates in your demand planning process. We focus on forecasts made in support of demand planning as well as forecasts inherent in optimizing inventory policies involving reorder points, safety stocks, and min/max levels.
Reading this, you will learn about:
-Criteria for assessing forecasts
-Sources of forecast error
-Calculating forecast error
-Converting forecast error into prediction intervals
-The relationship between demand forecasting and inventory optimization.
-Actions you can take to use these concepts to improve your company’s processes.
Criteria for Assessing Forecasts
Forecast error alone is not reason enough to reject forecasting as a management tool. To twist a famous aphorism by George Box, “All forecasts are wrong, but some are useful.” Of course, business professionals will always search for ways to make forecasts more useful. This usually involves work to reduce forecast error. But while forecast accuracy is the most obvious criterion by which to judge forecasts, but it is not the only one. Here’s a list of criteria for evaluating forecasts:
Accuracy: Forecasts of future values should, in retrospect, be very close to the actual values that eventually reveal themselves. But there may be diminishing returns to squeezing another half percent of accuracy out of forecasts otherwise good enough to use in decision making.
Timeliness: Fighter pilots refer to the OODA Loop (Observe, Orient, Decide, and Act) and the “need to get inside the enemy’s OODA loop” so they can shoot first. Businesses too have decision cycles. Delivering a perfectly accurate forecast the day after it was needed is not helpful. Better is a good forecast that arrives in time to be useful.
Cost: Forecasting data, models, processes and people all cost money. A less expensive forecast might be fueled by data that are readily available; more expensive would be a forecast that runs on data that have to be collected in a special process outside the scope of a firm’s information infrastructure. A classic, off-the-shelf forecasting technique will be less costly to acquire, feed and exploit than a complex, custom, consultant-supplied method. Forecasts could be mass-produced by software overseen by a single analyst, or they might emerge from a collaborative process requiring time and effort from large groups of people, such as district sales managers, production teams, and others. Technically advanced forecasting techniques often require hiring staff with specialized technical expertise, such as a master’s degree in statistics, who tend to cost more than staff with less advanced training.
Credibility: Ultimately, some executive has to accept and act on each forecast. Executives have a tendency to distrust or ignore recommendations that they can neither understand nor explain to the next person above them in the hierarchy. For many, believing in a “black box” is too severe a test of faith, and they reject the black box’s forecasts in favor of something more transparent.
All that said, we will focus now on forecast accuracy and its evil twin, forecast error.
Sources of Forecast Error
Those seeking to reduce error can look in three places to find trouble:
1. The data that goes into a forecasting model
2. The model itself
3. The context of the forecasting exercise
There are several ways in which data problems can lead to forecast error.
Gross errors: Wrong data produce wrong forecasts. We have seen an instance in which computer records of product demand were wrong by a factor of two! Those involved spotted that problem immediately, but a less egregious situation can easily slip through to poison the forecasting process. In fact, just organizing, acquiring and checking data is often the largest source of delay in the implementation of forecasting software. Many data problems seem to derive from the data having been unimportant until a forecasting project made them important.
Anomalies: Even with perfectly curated forecasting databases, there are often “needle in a haystack” type data problems. In these cases, it is not data errors but demand anomalies that contribute to forecast error. In a set of, say, 50,000 products, some number of items are likely to have odd details that can distort forecasts.
Holdout analysis is a simple but powerful method of analysis. To see how well a method forecasts, use it with older known data to forecast newer data, then see how it would have turned out! For instance, suppose you have 36 months of demand data and need to forecast 3 months ahead. You can simulate the forecasting process by holding out (i.e., hiding) the most recent 3 months of data, forecasting using only data from months 1 to 33, then comparing the forecasts for months 34-36 against the actual values in months 34-36. Sliding simulation merely repeats the holdout analysis, sliding along the demand history. The example above used the first 33 months of data to get 3 estimates of forecast error. Suppose we start the process by using the first 12 months to forecast the next 3. Then we slide forward and use the first 13 months to forecast the next 3. We continue until finally we use the first 35 months to forecast the last month, giving us one more estimate of the error we make when forecasting one month ahead. Summarizing all the 1-step ahead, 2-step ahead and 3-step ahead forecast errors provides a way to calculate prediction intervals.
Calculating Prediction Intervals
The final step in calculating prediction intervals is to convert the estimates of average absolute error into the upper and lower limits of the prediction interval. The prediction interval at any future time is computed as
Prediction interval = Forecast ± Multiplier x Average absolute error.
The final step is the choice of the multiplier. The typical approach is to imagine some probability distribution of error around the forecast, then estimate the ends of the prediction interval using appropriate percentiles of that distribution. Usually, the assumed distribution of error is the Normal distribution, also called the Gaussian distribution or the “bell-shaped curve”.
Use of Prediction Intervals
The most immediate, informal use of prediction intervals is to convey a sense of how “squishy” a forecast is. Prediction intervals that are wide compared to the size of the forecasts indicate high uncertainty.
There are two more formal uses in demand forecasting: Hedging your bets about future demand and guiding forecast adjustment.
Hedging your bets: The forecast values themselves approximate the most likely values of future demand. A more ominous way to say the same thing is that there is about a 50% chance that the actual value will be above (or below) the forecast. If the forecast is being used to plan future production (or raw materials purchase or hiring), you might want to build in a cushion to keep from being caught short if demand spikes (assuming that under-building is worse than over-building). If the forecast is converted from units to dollars for revenue projections, you might want to use a value below the forecast to be conservative in projecting cash flow. In either case, you first have to choose the coverage of the prediction interval. A 90% prediction interval is a range of values that covers 90% of the possibilities. This implies that there is a 5% chance of a value falling above the upper limit of the 90% prediction interval. In other words, the upper limit of a 90% prediction interval marks the 95th percentile of the distribution of predicted demand at that time period. Similarly, there is a 5% chance of falling below the lower limit, which marks the 5th percentile of the demand distribution.
Guiding forecast adjustment: It is quite common for statistical forecasts to be revised by some sort of collaborative process. These adjustments are based on information not recorded in an item’s demand history, such as intelligence about competitor actions. Sometimes they are based on a more vaporous source, such as sales force optimism. When the adjustments are made on-screen for all to see, the prediction intervals provide a useful reference: If someone wants to move the forecasts outside the prediction intervals, they are crossing a fact-based line and should have a good story to justify their argument that things will be really different in the future.
Prediction Intervals and Inventory Optimization
Finally, the concept behind prediction intervals play an essential role in a problem related to demand forecasting: Inventory Optimization.
The core analytic task in setting reorder points (also called Mins) is to forecast total demand over a replenishment lead time. This total is called the lead time demand. When on-hand inventory falls down to or below the reorder point, a replenishment order is triggered. If the reorder point is high enough, there will be an acceptably small risk of a stockout, i.e., of lead time demand driving inventory below zero and creating either lost sales or backorders.
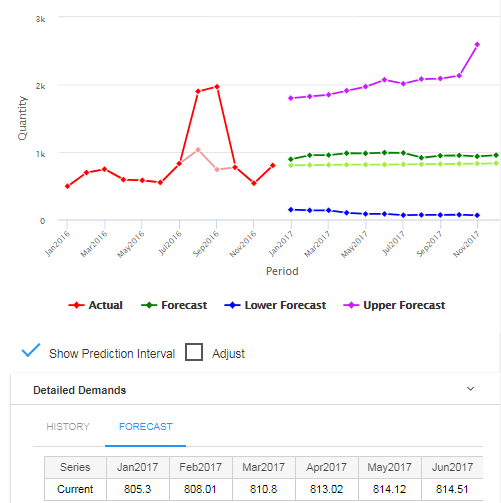
New statistical methods, and we can start planning more effectively.
The forecasting task is to determine all the possible values of cumulative demand over the lead time and their associated probabilities of occurring. In other words, the basic task is to determine a prediction interval for some future random variable. Suppose you have computed a 90% prediction interval for lead time demand. Then the upper end of the interval represents the 95th percentile of the distribution. Setting the reorder point at this level will accommodate 95% of the possible lead time demand values, meaning there will be only a 5% chance of stocking out before replenishment arrives to re-stock the shelves. Thus there is an intimate relationship between prediction intervals in demand forecasting and calculation of reorder points in inventory optimization.
5 Recommendations for Practice
1. Set expectations about error: Sometimes managers have unreasonable expectations about reducing forecast error to zero. You can point out that error is only one of the dimensions on which a forecasting process must be judged; you may be doing fine on both timeliness and cost. Also point out that zero error is no more realistic a goal than 100% conversion of prospects into customers, perfect supplier performance, or zero stock price volatility.
2. Track down sources of error: Double check the accuracy of demand histories. Use statistical methods to identify outliers in demand histories and react appropriately, replacing verified anomalies with more typical values and omitting data from before major changes in the character of the demand. If you use a collaborative forecasting process, compare its accuracy against a purely statistical approach to identify items for which collaboration does not reduce error.
3. Evaluate the error of alternative statistical methods: There may be off-the-shelf techniques that do better than your current methods, or do better for some subsets of your items. The key is to be empirical, using the idea of holdout analysis. Gather your data and do a “bake off” between different methods to see which work better for you. If you are not already using statistical forecasting methods, compare them against whoever’s “golden gut” is your current standard. Use the naïve forecast as a benchmark in the comparisons.
4. Investigate the use of new data sources: Especially if you have items that are heavily promoted, test out statistical methods that incorporate promotional data into the forecasting process. Also check whether information from outside your company can be exploited; for instance, see whether macroeconomic indicators for your sector can be combined with company data to improve forecast accuracy (this is usually done using a method called multiple regression analysis).
5. Use prediction intervals: Plots of prediction intervals can improve your feel for the uncertainty in your forecasts, helping you select items for additional scrutiny. While it’s true that what you don’t know can hurt you, it’s also true that knowing what you don’t know can help you.
Related Posts

Managing Spare Parts Inventory: Best Practices
In this blog, we’ll explore several effective strategies for managing spare parts inventory, emphasizing the importance of optimizing stock levels, maintaining service levels, and using smart tools to aid in decision-making. Managing spare parts inventory is a critical component for businesses that depend on equipment uptime and service reliability. Unlike regular inventory items, spare parts often have unpredictable demand patterns, making them more challenging to manage effectively. An efficient spare parts inventory management system helps prevent stockouts that can lead to operational downtime and costly delays while also avoiding overstocking that unnecessarily ties up capital and increases holding costs.

12 Causes of Overstocking and Practical Solutions
Managing inventory effectively is critical for maintaining a healthy balance sheet and ensuring that resources are optimally allocated. Here is an in-depth exploration of the main causes of overstocking, their implications, and possible solutions.

FAQ: Mastering Smart IP&O for Better Inventory Management.
Effective supply chain and inventory management are essential for achieving operational efficiency and customer satisfaction. This blog provides clear and concise answers to some basic and other common questions from our Smart IP&O customers, offering practical insights to overcome typical challenges and enhance your inventory management practices. Focusing on these key areas, we help you transform complex inventory issues into strategic, manageable actions that reduce costs and improve overall performance with Smart IP&O.