Improve Forecast Accuracy, Eliminate Excess Inventory, & Maximize Service Levels
In this video tutorial Dr. Thomas Willemain, co–Founder and SVP Research at Smart Software, presents Automatic Forecasting for Time Series Demand Projections, a specialized algorithmic tournament to determine an appropriate time series model and estimate the parameters to compute the best forecasts methods. Automatic forecasts of large numbers of time series are frequently used in business, some have trend either up or down, and some have seasonality so they are cyclic, and each of those specific patterns requires a suitable technical approach, and an appropriate statistical forecasting method. Tom explains how the tournament computes the best forecasts methods and works through a practical example.
RECENT POSTS
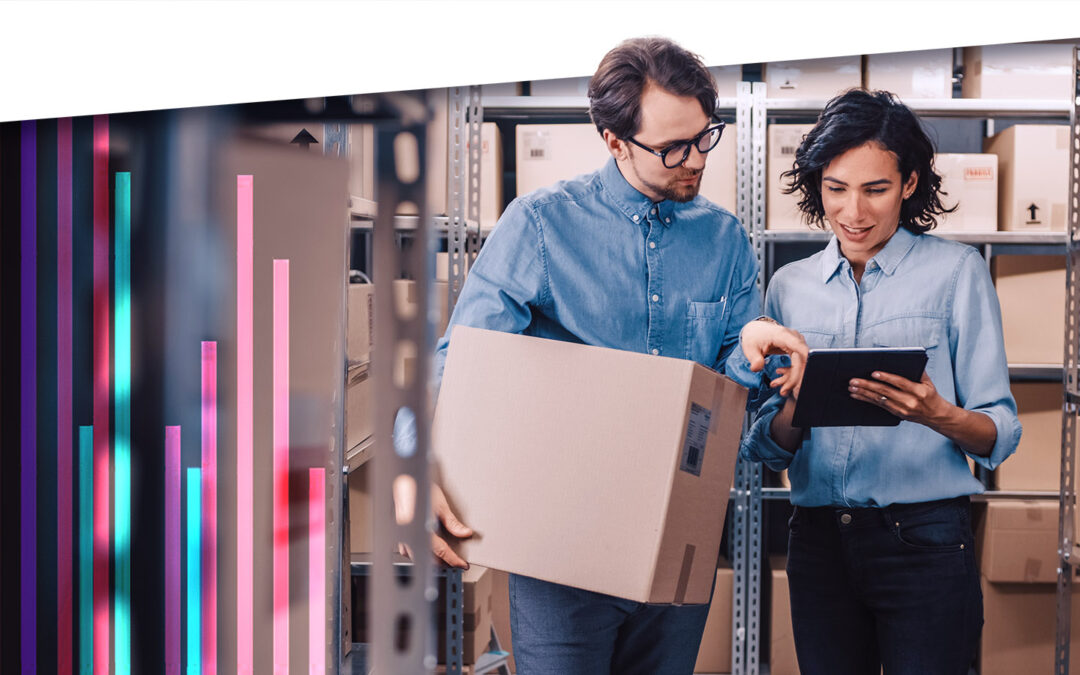
Forecast-Based Inventory Management for Better Planning
Forecast-based inventory management, or MRP (Material Requirements Planning) logic, is a forward-planning method that helps businesses meet demand without overstocking or understocking. By anticipating demand and adjusting inventory levels, it maintains a balance between meeting customer needs and minimizing excess inventory costs. This approach optimizes operations, reduces waste, and enhances customer satisfaction.
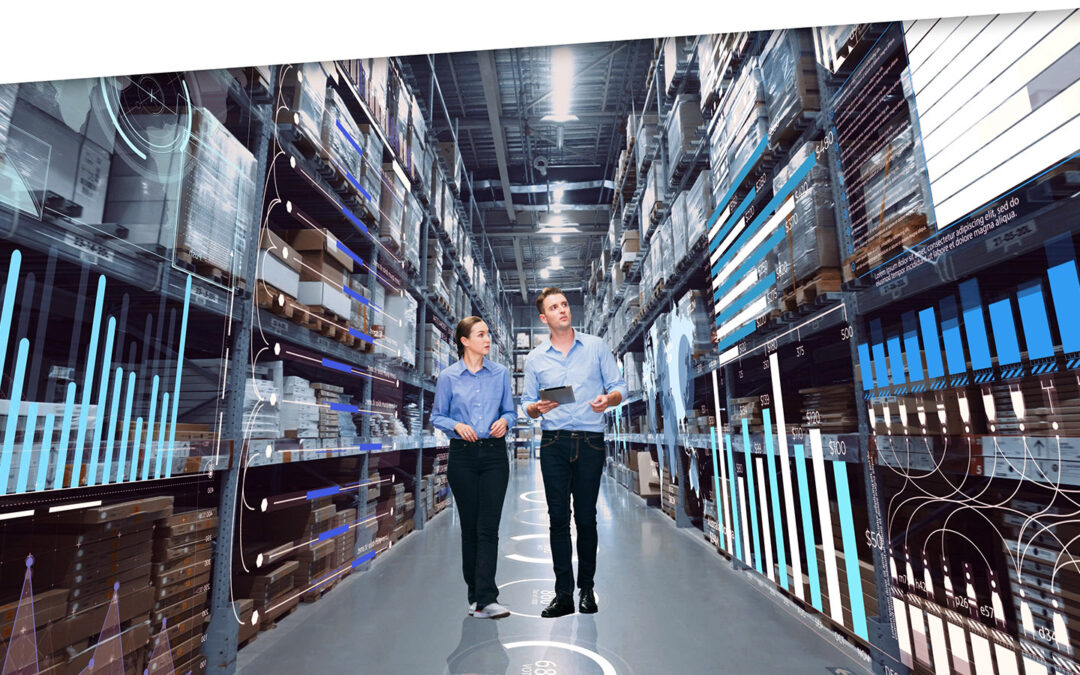
Make AI-Driven Inventory Optimization an Ally for Your Organization
In this blog, we will explore how organizations can achieve exceptional efficiency and accuracy with AI-driven inventory optimization. Traditional inventory management methods often fall short due to their reactive nature and reliance on manual processes. Maintaining optimal inventory levels is fundamental for meeting customer demand while minimizing costs. The introduction of AI-driven inventory optimization can significantly reduce the burden of manual processes, providing relief to supply chain managers from tedious tasks.
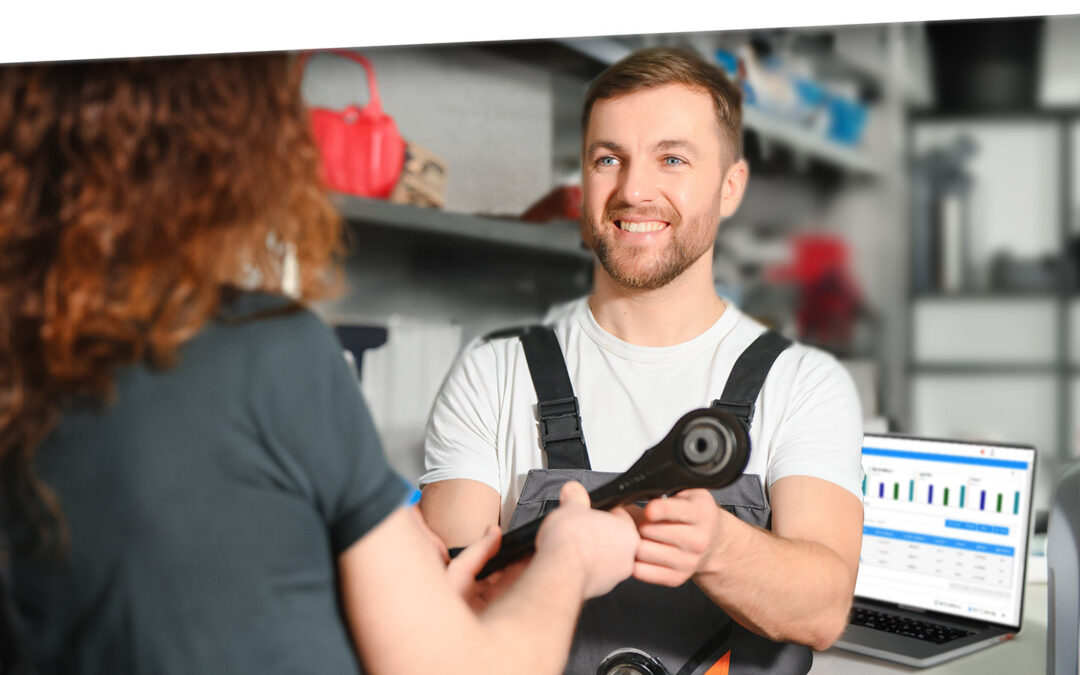
The Importance of Clear Service Level Definitions in Inventory Management
Inventory optimization software that supports what-if analysis will expose the tradeoff of stockouts vs. excess costs of varying service level targets. But first it is important to identify how “service levels” is interpreted, measured, and reported. This will avoid miscommunication and the false sense of security that can develop when less stringent definitions are used. Clearly defining how service level is calculated puts all stakeholders on the same page. This facilitates better decision-making.