We often come into contact with potential customers who claim that they cannot use a forecasting system since they are a “build-to-order” manufacturing operation. I find this a puzzling perspective, because whatever these organizations build requires lower level raw materials or intermediate goods. If those lower level inputs are not available when an order for the finished good is received, the order cannot be built. Consequently, the order could be canceled and the associated revenue lost.
I agree that in such an environment, forecasting the finished good is not always possible or particularly helpful. Sometimes it’s helpful, but not sufficient. In any case, it is critical to make sure that the underlying raw materials and intermediate goods that go into the finished good are available. Demand for these can certainly be forecasted.
The organization’s goal would be to maintain service level inventories for these intermediate goods that are high but not unaffordable. Planners will need to set optimal stocking levels for these materials, balancing service level requirements against available budget. Since a given intermediate good could serve as an input to more than one finished good, the volatility of the demand for the intermediate good would be less than the volatility of the demand for a specific finished good. Hence, the safety stocks necessary to keep high service level inventories of the intermediate goods would be relatively lean.
Three companies, all users of SmartForecasts, serve as interesting examples. The first is a chemical company, Bedoukian Research, which manufactures custom chemicals for various clients. Each of these “finished goods” is a unique combination of intermediate chemical compounds. Bedoukian begins its demand planning with a finished goods forecast, which drives the production schedule and allocation of essential production resources. This requires exercising considerable judgment, as finished goods demand changes dynamically.
Once these finished good forecasts are created, raw material requirements can be estimated via a bill of material disaggregation. Bedoukian combines these results with safety stock estimates, based on actual utilization rates and service level objectives to be achieved, to generate the complete, service level-driven forecast for raw materials. This has allowed Bedoukian meet its production requirements with significantly less inventory.
The second company manufactures the internal components for mobile phones, where finished goods are specialized combinations of these components. For example, an order may call for a certain number of phones with unique labels on the case. This is the finished good for this order. Everything that goes into that order, except for the label, is built out of standard components. Again, SmartForecasts will be used to keep lean, high service level inventories of the components. This company thought that the only way to manage component inventories was via bill of material aggregations. They are now looking at the actual utilization rate for the components and setting much leaner inventories while maintaining high component availability.
A third company, NKK Switches, which explored this topic in their recent webinar (see CFO Bud Schultz’ guest blog post), considered their products to be “unforecastable”. You can read more about it below, but overall NKK Switches was able to forecast components and meaningful aggregations of product families. By tracking forecast vs. actuals over several months, NKK was able to demonstrate the accuracy of its forecasts to its Asian factory suppliers, and convince them to shift from a “build-to-order” model to “build-to-forecast.” This change has resulted in dramatic reductions in lead times, in many cases cutting them in half, increasing customer satisfaction and the overall sales close rate.
The bottom line here is that there is a perfectly viable—I would say essential—method of demand forecasting for build-to-order businesses, setting high service levels for pivotal input resources. If you would like to know more, please drop me a note, at nelsonh at smartcorp dot com.
Nelson Hartunian, PhD, co-founded Smart Software, formerly served as President, and currently oversees it as Chairman of the Board. He has, at various times, headed software development, sales and customer service.
Related Posts
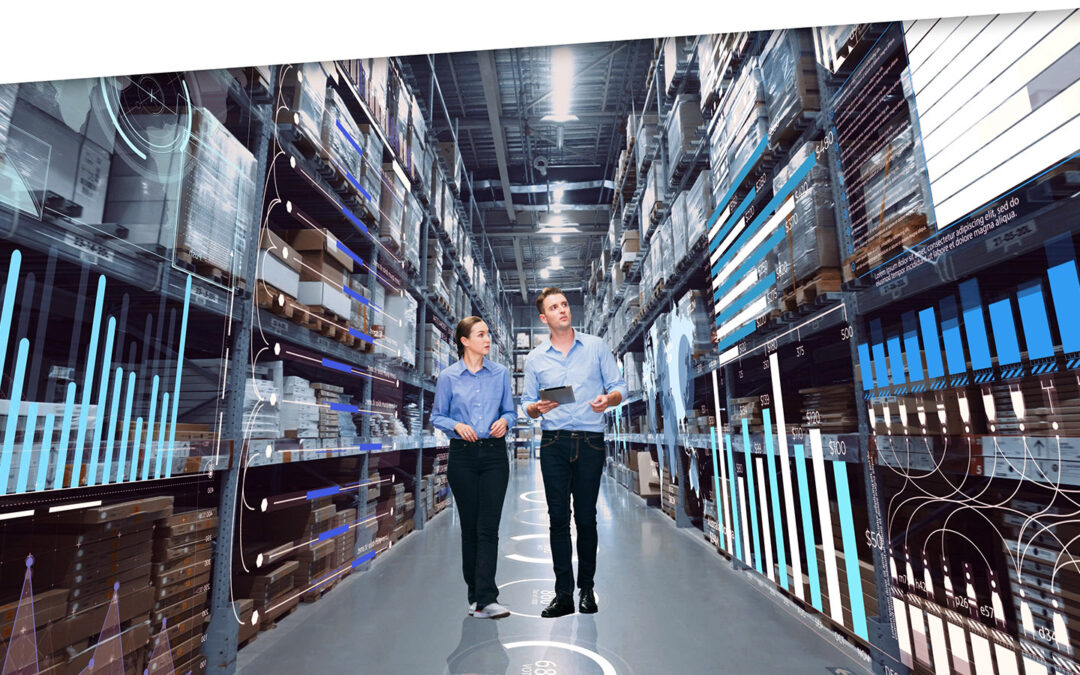
Make AI-Driven Inventory Optimization an Ally for Your Organization
In this blog, we will explore how organizations can achieve exceptional efficiency and accuracy with AI-driven inventory optimization. Traditional inventory management methods often fall short due to their reactive nature and reliance on manual processes. Maintaining optimal inventory levels is fundamental for meeting customer demand while minimizing costs. The introduction of AI-driven inventory optimization can significantly reduce the burden of manual processes, providing relief to supply chain managers from tedious tasks.
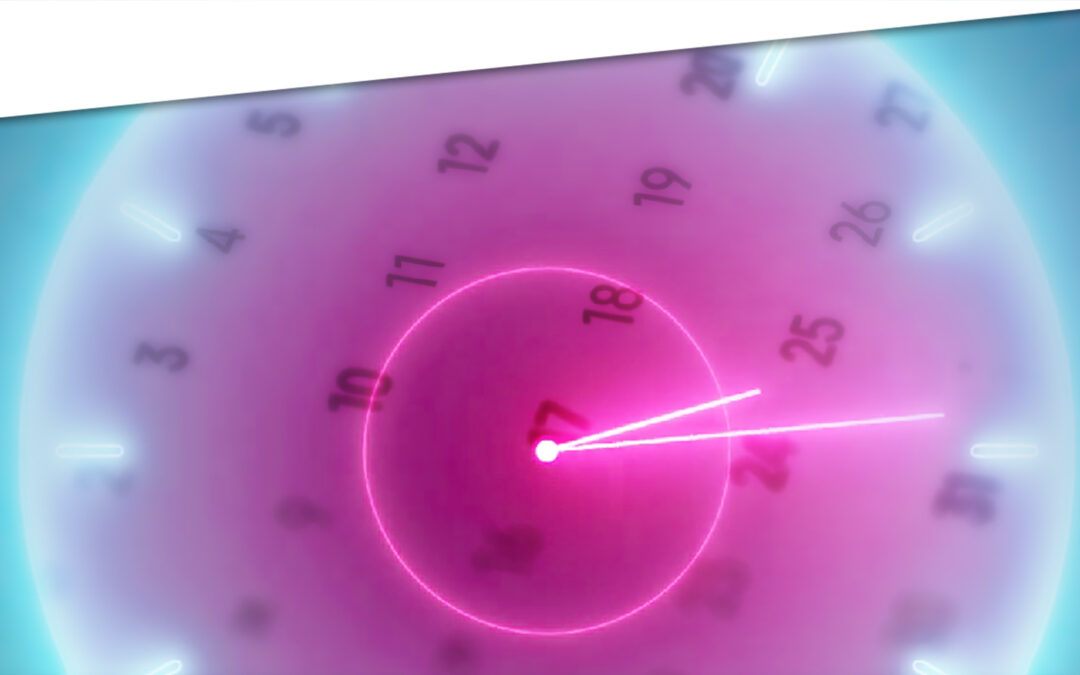
Daily Demand Scenarios
In this Videoblog, we will explain how time series forecasting has emerged as a pivotal tool, particularly at the daily level, which Smart Software has been pioneering since its inception over forty years ago. The evolution of business practices from annual to more refined temporal increments like monthly and now daily data analysis illustrates a significant shift in operational strategies.
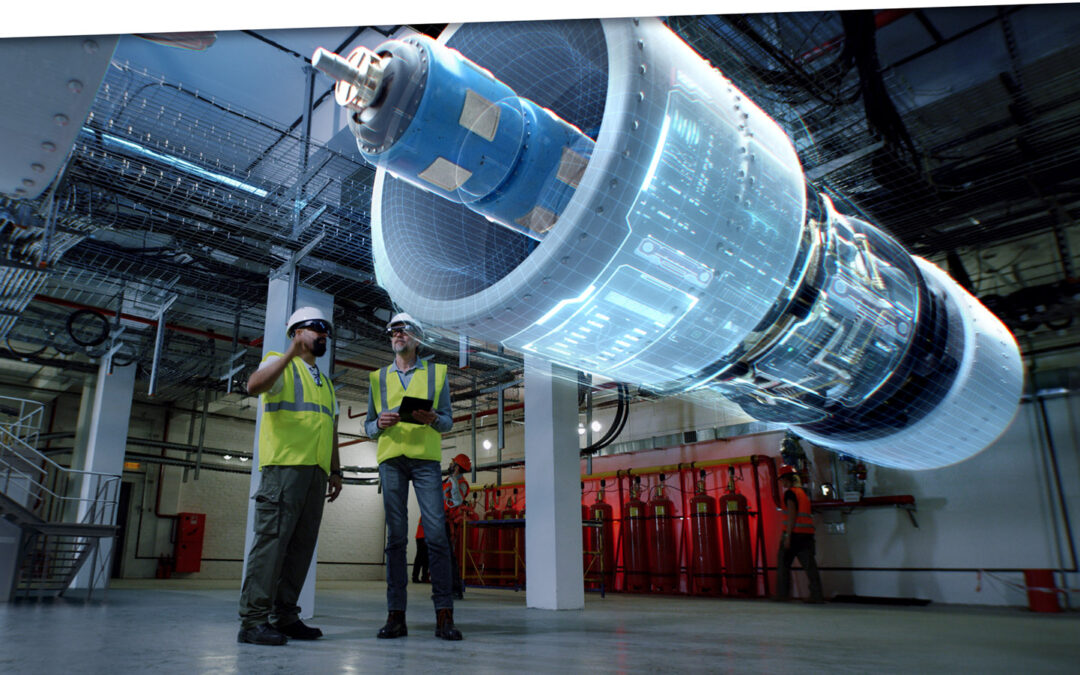
Constructive Play with Digital Twins
Those of you who track hot topics will be familiar with the term “digital twin.” Those who have been too busy with work may want to read on and catch up. While there are several definitions of digital twin, here’s one that works well: A digital twin is a dynamic virtual copy of a physical asset, process, system, or environment that looks like and behaves identically to its real-world counterpart. A digital twin ingests data and replicates processes so you can predict possible performance outcomes and issues that the real-world product might undergo.