“Regardless of how much effort is poured into training forecasters and developing elaborate forecast support systems, decision-makers will either modify or discard the predictions if they do not trust them.” — Dilek Onkal, International Journal of Forecasting 38:3 (July-September 2022), p.802.
The words quoted above grabbed my attention and prompted this post. Those of a geekly persuasion, like your blogger, are inclined to think of forecasting as a statistical problem. While that is obviously true, those of a certain age, like your blogger, understand that forecasting is also a social activity and therefore has a large human component.
Who Do You Trust?
Trust is always a two-way street, but let’s stay on the demand forecaster’s side. What characteristics of and actions by forecasters and demand planners build trust in their work? The above quoted Professor Onkal reviewed academic research on this topic going back to 2006. She summarized results from practitioner surveys that identified key trust factors related to forecaster characteristics, forecasting process, and forecasting communication.
Forecaster characteristics
Key to building trust among the users of forecasts are perceptions of forecaster and demand planner competence and objectivity. Competence has a mathematical component, but many managers confuse computer skills with analytic skills, so users of forecasting software can usually clear this hurdle. However, since the two are not the same, it pays dividends to absorb your vendor’s training and learn not just the math but the lingo of your forecasting software. In my observation, trust can also be increased by showing knowledge of the company’s business.
Objectivity is also a key to trustworthiness. It may be uncomfortable for the forecaster to be put in the middle of occasional departmental squabbles, but those will come up and must be handled with tact. Squabbles? Well, silos exist and tilt in different directions. Sales departments favor higher demand forecasts that drive production increases, so that they never have to say “Sorry, we are fresh out of that.” Inventory managers are wary of high demand forecasts, because “excess enthusiasm” can leave them holding the bag, sitting on bloated inventory.
Sometimes the forecaster becomes a de facto referee, and in this role must display overt signs of objectivity. That can mean first recognizing that every management decision involves tradeoffs of good things against other good things, e.g., product availability versus lean operations, and then helping the parties strike a painful but tolerable balance by surfacing the links between operational decisions and the key performance metrics that matter to folks like Chief Financial Officers.
The Forecasting process
The forecasting process can be thought of as having three phases: data inputs, calculations, and outputs. Actions can be taken to increase trust in each phase.
Regarding inputs:
Trust can be increased if obviously relevant inputs are at least acknowledged if not directly used in calculations. Thus, factors like social media sentiment and regional sales managers’ gut instincts can be legitimate parts of a forecast consensus process. However, objectivity requires that these putative predictors of profit be tested objectively. For instance, a professional-grade forecasting process may well include subjective adjustment to statistical forecasts but must then also assess whether the adjustments actually end up improving accuracy, not just making some people feel listened to.
Regarding the second phase, calculations:
The forecaster will be trusted to the extent that they are able to deploy more than one way to calculate forecasts and then articulate a good reason why they chose the method eventually used. In addition, the forecaster should be able to explain in accessible language how even complicated techniques do their job. It is difficult to put trust in a “black box” method that is so opaque as to be inscrutable. The importance of explainability is amplified by the fact of life that the forecaster’s superior must themselves in turn be able to justify the choice of technique to their supervisor.
For instance, exponential smoothing uses this equation: S(t) = αX(t)+(1-α)S(t-1). Many forecasters are familiar with this equation, but many forecast users are not. There is a story that explains the equation in terms of averaging irrelevant “noise” in an item’s demand history and the need to strike a balance between smoothing out noise and being able to react to sudden shifts in the level of demand. The forecaster who can tell that story will be more credible. (My own version of that story uses phrases from sports, i.e., “head fakes” and “jukes”. Finding folksy analogs appropriate to your specific audience always pays dividends.)
A final point: best practice demands that any forecast be accompanied by an honest assessment of its uncertainty. A forecaster who tries to build trust by being overly specific (“Sales next quarter will be 12,184 units”) will always fail. A forecaster who says “Sales next quarter will have a 90% chance of falling between 12,000 and 12,300 units” will be both correct more often and also more helpful to decision makers. After all, forecasting is essentially a job of risk management, so the decision maker is best served by knowing the risks.
Forecasting communication:
Finally, consider the third phase, communication of forecast results. Research suggests that continual communication with forecast users builds trust. It avoids those horrible, deflating moments when a nicely formatted report is shot down because of some fatal flaw that could have been foreseen: “This is no good because you didn’t take account of X, Y or Z” or “We really wanted you to present results rolled up to the top of the product hierarchies (or by sales region or by product line or…)”.
Even when everybody is aligned as to what is expected, trust is enhanced by presenting results using well-crafted graphics, with massive numerical tables provided for backup but not as the main way of communicating results. My experience has been that, just as a meeting-control device, a graph is usually much better than a large numerical table. With a graph, everybody’s attention is focused on the same thing and many aspects of the analysis are immediately (and literally) visible. With a table of results, the table of participants often splinters into side conversations in which each voice is focused on different pieces of the table.
Onkal summarizes the research this way: “Take-aways for those who make forecasts and those who use them converge around clarity of communication as well as perceptions of competence and integrity.”
What Do You Trust?
There is a related dimension of trust: not who do you trust but what do you trust? By this I mean both data and software…. Read the 2nd part of this Blog “What do you Trust” here https://smartcorp.com/forecasting/the-role-of-trust-in-the-demand-forecasting-process-part-2-what/
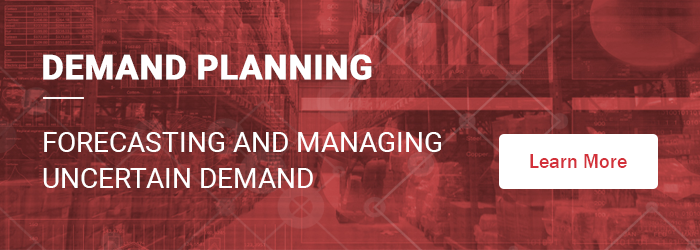