Data verification and validation are essential to the success of the implementation of software that performs statistical analysis of data, like Smart IP&O. This article describes the issue and serves as a practical guide to doing the job right, especially for the user of the new application.
The less experience your organization has in validating historical transactions or item master attributes, the more likely it is there were problems or mistakes with data entry into the ERP that have so far gone unnoticed. The garbage in, garbage out rule means you need to prioritize this step of the software onboarding process or risk delay and possible failure to generate ROI.
Ultimately the best person to confirm data in your ERP is entered correctly is the person who knows the business and can assert, for example, “this part doesn’t belong to that product group.” That’s usually the same person who will open and use Smart. Though a database administrator or IT support can also play a key role by being able to say, “This part was assigned to that product group last December by Jane Smith.” Ensuring data is correct may not be a regular part of your day job but can be broken down into manageable small tasks that a good project manager will allocate the time and resources to complete.
The demand planning software vendor receiving the data also has a role. They will confirm that the raw data was ingested without issue. The vendor can also identify abnormalities in the raw data files that point to the need for validation. But relying on the software vendor to reassure you the data looks fine is not enough. You don’t want to discover, after go-live, that you can’t trust the output because some of the data “doesn’t make sense.”
Each step in the data flow needs verification and validation. Verification means the data at one step is still the same after flowing to the next step. Validation means the data is correct and usable for analysis.
The most common data flow looks like this:
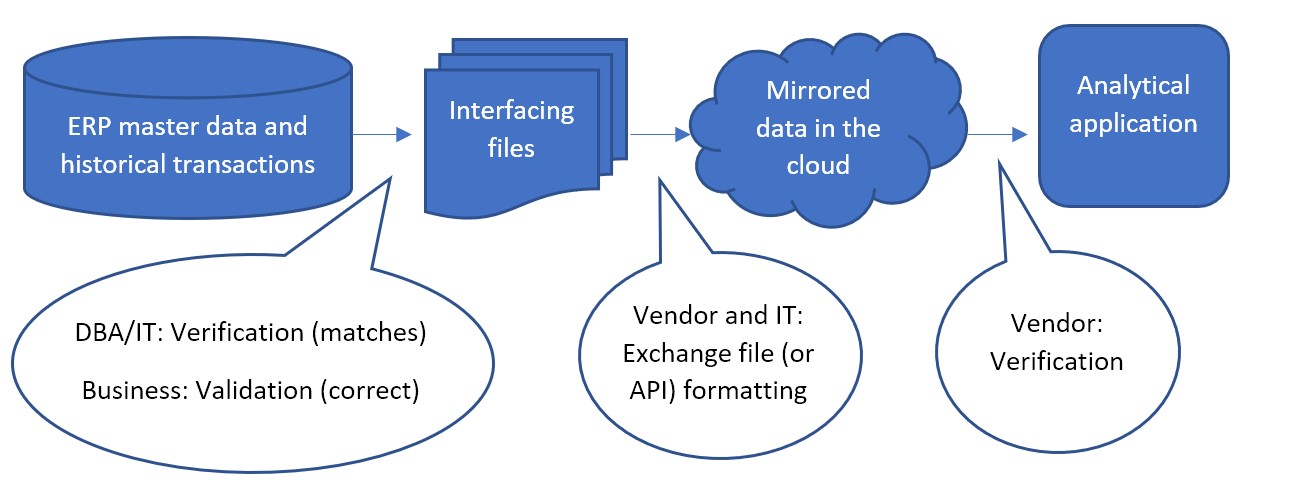
Less commonly, the first step between ERP master data and the interfacing files can sometimes be bypassed, where files are not used as an interface. Instead, an API built by IT or the inventory optimization software vendor is responsible for data to be written directly from the ERP to the mirrored database in the cloud. The vendor would work with IT to confirm the API is working as expected. But the first validation step, even in that case, can still be performed. After ingesting the data, the vendor can make the mirrored data available in files for the DBA/IT verification and business validation.
The confirmation that the mirrored data in the cloud completes the flow into the application is the responsibility of the vendor of software as a service. SaaS vendors continually test that the software works correctly between the front-end application their subscribers see and the back-end data in the cloud database. If the subscribers still think the data doesn’t make sense in the application even after validating the interfacing files before going live, that is an issue to raise with the vendor’s customer support.
However the interfacing files are obtained, the largest part of verification and validation falls to the project manager and their team. They must resource a test of the interfacing files to confirm:
- They match the data in the ERP. And that all and only the ERP data that was necessary to extract for use in the application was extracted.
- Nothing “jumps out” to the business as incorrect for each of the types of information in the data
- They are formatted as expected.
DBA/IT Verification Tasks
- Test the extract:
IT’s verification step can be done with various tools, comparing files, or importing files back to the database as temporary tables and joining them with the original data to confirm a match. IT can depend on a query to pull the requested data into a file but that file can fail to match. The existence of delimiters or line returns within the data values can cause a file to be different than its original database table. It is because the file relies heavily on delimiters and line returns to identify fields and records, while the table doesn’t rely on those characters to define its structure.
- No bad characters:
Free form data entry fields in the ERP, such as product descriptions, can sometimes themselves contain line returns, tabs, commas, and/or double quotes that can affect the structure of the output file. Line returns should not be allowed in values that will be extracted to a file. Characters equal to the delimiter should be stripped during extract or else a different delimiter used.
Tip: if commas are the file delimiter, numbers greater than 999 can’t be extracted with a comma. Use “1000” rather than “1,000”.
- Confirm the filters:
The other way that query extracts can return unexpected results is if conditions on the query are entered incorrectly. The simplest way to avoid mistaken “where clauses” is to not use them. Extract all data and allow the vendor to filter out some records according to rules supplied by the business. If this will produce extract files so large that too much computing time is spent on the data exchange, the DBA/IT team should meet with the business to confirm exactly what filters on the data can be applied to avoid exchanging records that are meaningless to the application.
Tip: Bear in mind that Active/Inactive or item lifecycle information should not be used to filter out records. This information should be sent to the application so it knows when an item becomes inactive.
- Be consistent:
The extract process must produce files of consistent format every time it is executed. File names, field names, and position, delimiter, and Excel sheet name if Excel is used, numeric formats and date formats, and the use of quotes around values should never differ from one execution of the extract one day to the next. A hands-off report or stored procedure should be prepared and used for every execution of the extract.
Business Validation Background
Below is a break down each of validation step into considerations, specifically in the case where the vendor has provided a template format for the interfacing files where each type of information is provided in its own file. Files sent from your ERP to Smart are formatted for easy export from the ERP. That sort of format makes the comparison back to the ERP a relatively simple job for IT, but it can be harder for the business to interpret. Best practice is to manipulate the ERP data, either by using pivot tables or similar in a spreadsheet. IT may assist by providing re-formatted data files for review by the business.
To delve into the interfacing files, you’ll need to understand them. The vendor will supply a precise template, but generally interfacing files consist into three types: catalog data, item attributes, and transactional data.
- Catalog data contains identifiers and their attributes. Identifiers are typically for products, locations (which could be plants or warehouses), your customers, and your suppliers.
- Item attributes contain information about products at locations that are needed for analysis on the product and location combination. Such as:
- Current replenishment policy in the form of a Min and Max, Reorder Point, or Review Period and Order Up To value, or Safety Stock
- Primary supplier assignment and nominal lead time and cost per unit from that supplier
- Order quantity requirements such as minimum order quantity, manufacturing lot size, or order multiples
- Active/Inactive status of the product/location combination or flags that identify its state in its lifecycle, such as pre-obsolete
- Attributes for grouping or filtering, such as assigned buyer/planner or product category
- Current inventory information like on hand, on order, and in transit quantities.
- Transactional data contains references to identifiers along with dates and quantities. Such as quantity sold in a sales order of a product, at a location, for a customer, on a date. Or quantity placed on purchase order of a product, into a location, from a supplier, on a date. Or quantity used in a work order of a component product at a location on a date.
Validating Catalog Data
Considering catalog data first, you may have catalog files similar to these examples:
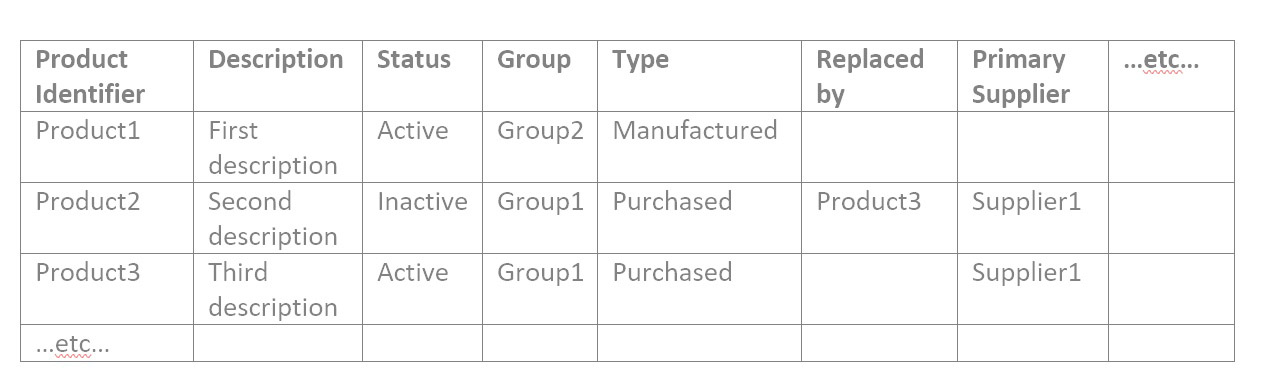
Location Identifier |
Description |
Region |
Source Location |
etc… |
Location1 |
First location |
North |
|
|
Location2 |
Second location |
South |
Location1 |
|
Location3 |
Third location |
South |
Location1 |
|
…etc… |
|
|
|
|
Customer Identifier |
Description |
SalesPerson |
Ship From Location |
etc… |
Customer1 |
First customer |
Jane |
Location1 |
|
Customer2 |
Second customer |
Jane |
Location3 |
|
Customer3 |
Third customer |
Joe |
Location2 |
|
…etc… |
|
|
|
|
Supplier Identifier |
Description |
Status |
Typical Lead Time Days |
etc… |
Supplier1 |
First supplier |
Active |
18 |
|
Supplier2 |
Second supplier |
Active |
60 |
|
Supplier3 |
Third Supplier |
Active |
5 |
|
…etc… |
|
|
|
|
1: Check for a reasonable count of catalog records
For each file of catalog data, open it in a spreadsheet tool like Google Sheets or MS Excel. Answer these questions:
- Is the record count in the ballpark? If you have about 50K products, there should not be only 10K rows in its file.
- If it’s a short file, maybe the Location file, you can confirm exactly that all expected Iidentifiers are in it.
- Filter by each attribute value and confirm again the count of records with that attribute value makes sense.
2: Check the correctness of values in each attribute field
Someone who knows what the products are and what the groups mean needs to take the time to confirm it is actually right, for all the attributes of all the catalog data.
So, if your Product file contains the attributes as in the example above, you would filter for Status of Active, and check that all resulting products are actually active. Then filter for Status of Inactive and check that all resulting products are actually inactive. Then filter for the first Group value and confirm all resulting products are in that group. Repeat for Group2 and Group3, etc. Then repeat for every attribute in every file.
It can help to do this validation with a comparison to an already existing and trusted report. If you have another spreadsheet that shows products by Group for any reason, you can compare the interfacing files to it. You may need to familiarize yourself with the VLOOKUP function that helps with spreadsheet comparison.
Validating Item Attribute Data
1: Check for a reasonable count of item records
The item attribute data confirmation is similar to the catalog data. Confirm the product/location combination count makes sense in total and for each of the unique item attributes, one by one. This is an example item data file:
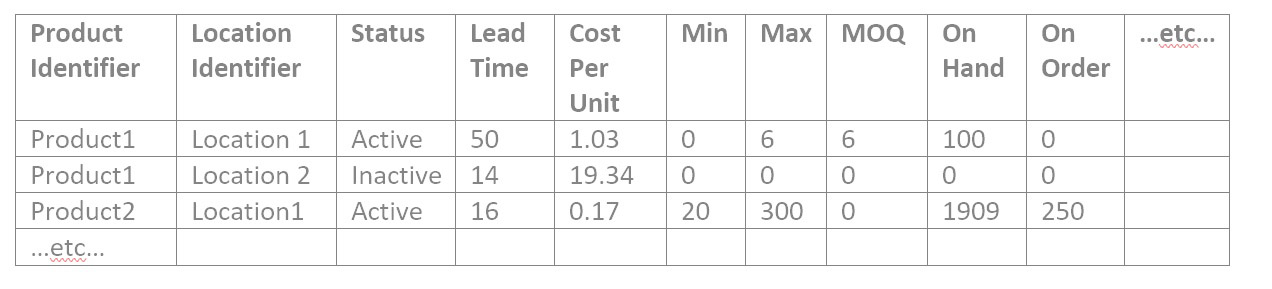
2: Find and explain weird numbers in item file
There tends to be many numerical values in the item attributes, so “weird” numbers merit review. To validate data for a numerical attribute in any file, search for where the number is:
- Missing entirely
- Equal to zero
- Less than zero
- More than most others, or less than most others (sort by that column)
- Not a number at all, when it should be
A special consideration of files that are not catalog files is they may not show the descriptions of the products and locations, just their identifiers, which can be meaningless to you. You can insert columns to hold the product and location descriptors that you are used to seeing and fill them into the spreadsheet to assist in your work. The VLOOKUP function works for this as well. Whether or not you have another report to compare the Items file to, you have the catalog files for Products and Location with show both the identifier and the description for each row.
3: Spot check
If you are frustrated to find that there are too many attribute values to manually check in a reasonable amount of time, spot checking is a solution. It can be done in a manner likely to pick up on any problems. For each attribute, get a list of the unique values in each column. You can copy a column into a new sheet, then use the Remove Duplicates function to see the list of possible values. With it:
- Confirm that no attribute values are present that shouldn’t be.
- It can be harder to remember which attribute values are missing that should be there, so it can help to look at another source to remind you. For example, if Group1 through Group12 are present, you might check another source to remember if these are all the Groups possible. Even if it is not required for the interfacing files for the application, it may be easy for IT to extract a list of all the possible Groups that are in your ERP which you can use for the validation exercise. If you find extra or missing values that you don’t expect, bring an example of each to IT to investigate.
- Sort alphabetically and scan down to see if any two values are similar but slightly different, maybe only in punctuation, which could mean one record had the attribute data entered incorrectly.
For each type of item, maybe one from each product group and/or location, check that all its attributes in every file are correct or at least pass a sanity check. The more you can spot check from a broad range of items, the less likely you will have issues post go live.
Validating Transactional Data
Transactional files may all have a format similar to this:
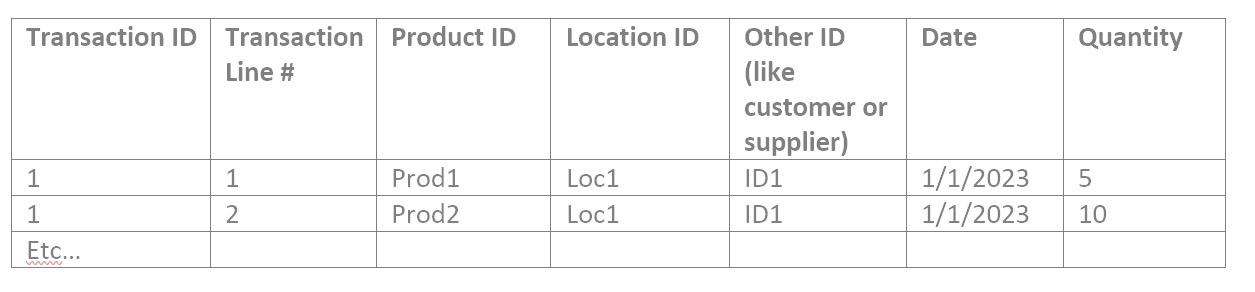
1: Find and explain weird numbers in each transactional file
These should be checked for “weird” numbers in the Quantity field. Then you can proceed to:
- Filter for dates outside the range you expect or missing expected dates entirely.
- Find where Transaction identifiers and line numbers are missing. They shouldn’t be.
- If there is more than one record for a given Transaction ID and Transaction Line Number combination, is that a mistake? Put another way, should duplicate records have their quantities summed together or is that double counting?
2: Sanity check summed quantities
Do a sanity check by filtering to a particular product you’re familiar with, and filter to a relatable date range such as last month or last year, and sum the quantities. Is that total amount what you expected for that product in that time frame? If you have information on total usage out of a location, you can slice the data that way to sum the quantities and compare to what you expect. Pivot tables come in handy for verification of transactional data. With them, you can view the data like:
Product |
Year |
Quantity Total |
Prod1 |
2022 |
9,034 |
Prod1 |
2021 |
8,837 |
etc |
|
|
The products’ yearly total may be simple to sanity check if you know the products well. Or you can VLOOKUP to add attributes, such as product group, and pivot on that to see a higher level that is more familiar:
Product Group |
Year |
Quantity Total |
Group1 |
2022 |
22,091 |
Group2 |
2021 |
17,494 |
etc |
|
|
3: Sanity check count of records
It may help to display a count of transactions rather than a sum of the quantities, especially for purchase order data. Such as:
Product |
Year |
Number of POs |
Prod1 |
2022 |
4 |
Prod1 |
2021 |
1 |
etc |
|
|
And/or the same summarization at a higher level, like:
Product Group |
Year |
Number of POs |
Group1 |
2022 |
609 |
Group2 |
2021 |
40 |
etc |
|
|
4: Spot checking
Spot checking the correctness of a single transaction, for each type of item and each type of transaction, completes due diligence. Pay special attention to what date is tied to the transaction, and whether it is right for the analysis. Dates may be a creation date, like the date a customer placed an order with you, or a promise date, like the date you expected to deliver on the customer’s order at the time of creating it, or a fulfilment date, when you actually delivered on the order. Sometimes a promise date gets modified days after creating the order if it can’t be met. Make sure the date in use reflects actual demand by the customer for the product most closely.
What to do about bad data
If the mis-entries are few or one-off, you can edit the ERP records by hand as they are found, cleaning up your catalog attributes, even after go-live with the application. But if large swathes of attributes or transaction quantities are off, this can spur an internal project to re-enter data correctly and possibly to change or start to document the process that needs to be followed when new records are entered into your ERP.
Care must be taken to avoid too long a delay in implementation of the SaaS application while waiting on clean attributes. Break the work into chunks and use the application to analyze the clean data first so the data cleansing project occurs in parallel with getting value out of the new application.
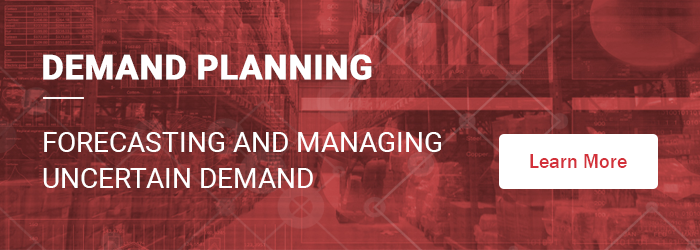