Inventory Planning from the Perspective of a Physicist
In a perfect world, Just in Time (JIT) would be the appropriate solution for inventory management. If you can exactly predict what you need and where you need it and your suppliers can get what you need without delay, then you do not need to maintain much inventory locally. But as the saying goes from famous pugilist Mike Tyson, “everyone has a plan until they get punched in the mouth.” And the latest punch in the mouth for the global supply chain was last week’s Suez Canal Blockage that held up $9.6B in trade costing an estimated $6.7M per minute[1]. Disruptions from these and similar events should be modeled and accounted for in your planning.
The assumption that you can exactly predict the future was apparent in Isaac Newton’s laws. Since the 1920’s with the introduction of quantum physics, uncertainty became fundamental to our understanding of nature. Uncertainty is built into fundamental reality. So too should it be built into Supply and Demand Planning processes. Yet too often, black swan events such as the Suez Canal blockage are often thought of as anomalies and as a result, discounted when planning. It is not enough to look back in hindsight and proclaim that it should have been expected. Something needs to be done about addressing the occurrence of other such events in the future and planning stocking levels accordingly.
We must move beyond the “thin tailed distribution” thinking where extreme outcomes are discounted and plan for “fat tails.” So how do we execute a real-world JIT plan when it comes to planning inventory? To do this, the first step is to estimate the realistic lead time to obtain an item. However, estimation is difficult due to lead time uncertainty. Using actual supplier lead times in your company database and external data, you can develop a distribution of possible future lead times and demands within those lead times. Probabilistic forecasting will allow you to account for disruptions and unusual events by not limiting your estimates to what has been observed solely on your own short-term demand and lead time data. You’ll be able to generate possible outcomes with associated probabilities for each occurrence.
Once you have an estimate of the lead time and demand distribution, you can then specify the service level you need to have for that part. Using solutions such as Smart Inventory Optimization (SIO), you will be able confidently stock based on the targeted stock-out risk with minimal inventory carrying cost. You may also consider letting the solution prescribe optimal service level targets by assessing the costs of additional inventory vs. cost of stockout.
Finalmente, como ya he señalado, debemos aceptar que nunca podremos eliminar toda la incertidumbre. Como físico, siempre me ha intrigado el hecho de que, incluso en los niveles más básicos de la realidad tal como la entendemos hoy, todavía hay incertidumbre. Albert Einstein creía en la certeza (determinismo) de la ley física. Si fuera gerente de inventario, podría haber abogado por JIT porque creía que las leyes físicas deberían permitir una previsibilidad perfecta. Él dijo célebremente: “Dios no juega con los dados”. ¿O podría ser posible que el universo en el que existimos fuera un evento de "cisne negro" en un "verso múltiple" anterior que produjo un tipo particular de universo que nos permitió existir?
En la planificación de inventarios, como en la ciencia, no podemos escapar de la realidad de la incertidumbre y el impacto de eventos inusuales. Debemos planificar en consecuencia.
[1] https://www.bbc.com/news/business-56559073#:~:text=Looking%20at%20the%20bigger%20picture,0.2%20to%200.4%20percentage%20points.
Artículos Relacionados
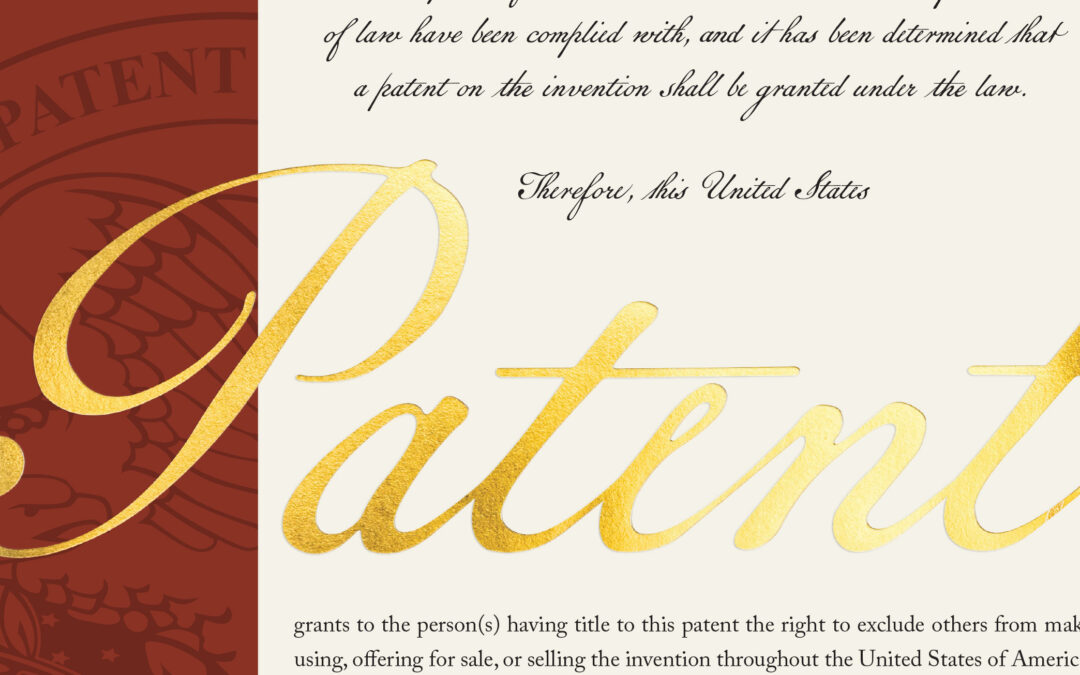
Smart Software anuncia patente de próxima generación
Smart Software se complace en anunciar la concesión de la patente estadounidense 11.656.887. La patente dirige “soluciones técnicas para analizar datos históricos de demanda de recursos en una plataforma tecnológica para facilitar la gestión de un proceso automatizado en la plataforma.
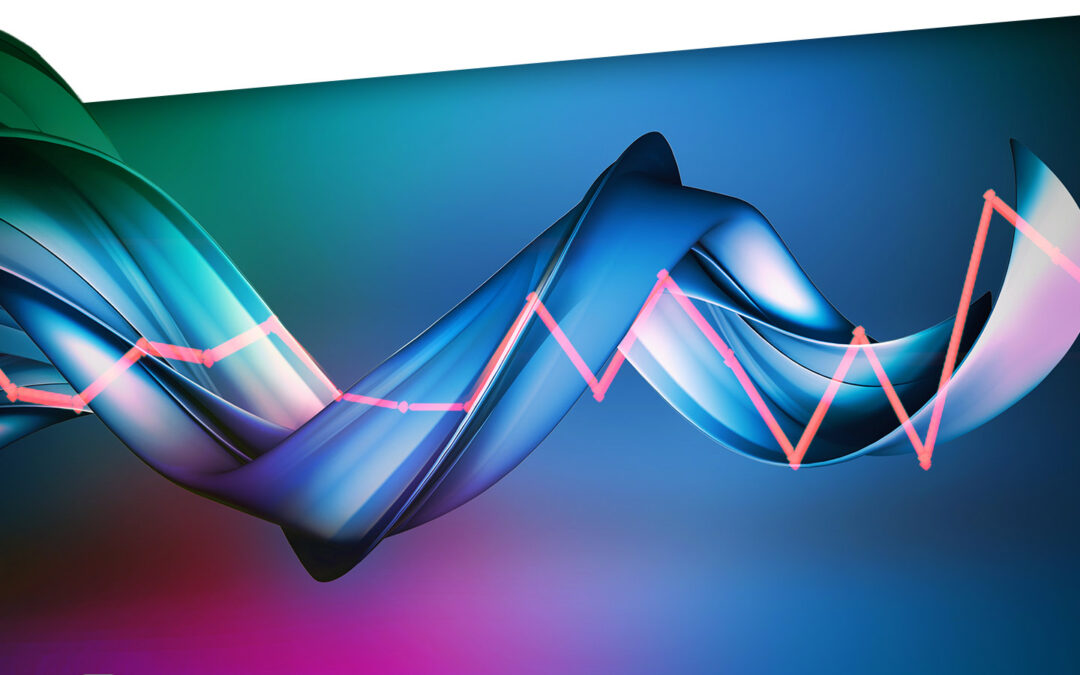
¿Sus pronósticos estadísticos sufren el efecto de oscilación?
¿Qué es el efecto meneo? Es cuando su pronóstico estadístico predice incorrectamente los altibajos observados en su historial de demanda cuando realmente no hay un patrón. Es importante asegurarse de que sus pronósticos no cambien a menos que haya un patrón real. Aquí hay una transcripción de un cliente reciente donde se discutió este problema:
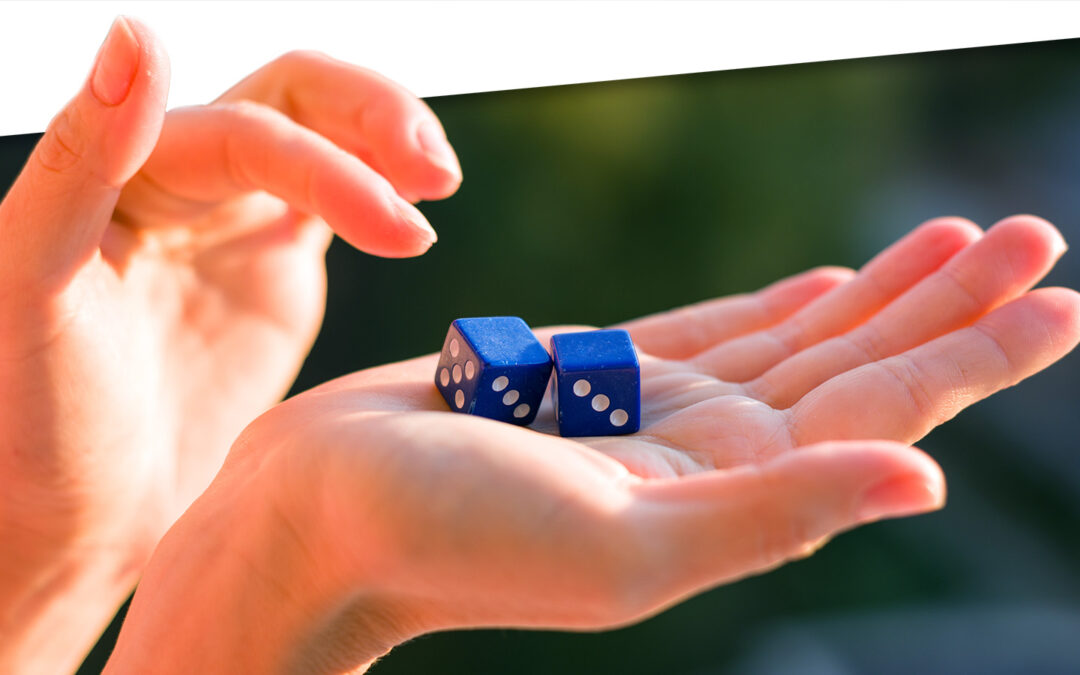
Cómo manejar pronósticos estadísticos de cero
Un pronóstico estadístico de cero puede causar mucha confusión a los pronosticadores, especialmente cuando la demanda histórica no es cero. Claro, es obvio que la demanda tiene una tendencia a la baja, pero ¿debería tener una tendencia a cero?